Ethical Challenges in AI-Driven Credit Scoring
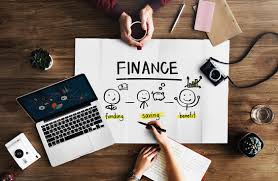
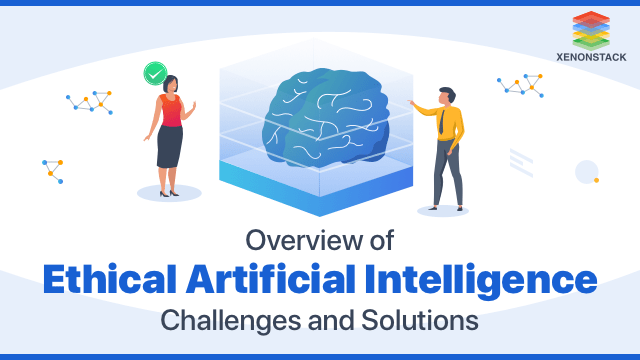
The use of AI in credit scoring mechanisms revolutionized the manner in which creditworthiness was ascertained in terms of speed and accuracy, thus expanding access to financial services to more people. Conversely, with increasing use of AI in credit scoring comes ethical challenges. The challenges range from the possibility of bias to the opacity of the algorithms used in AI, the violation of privacy, to larger ramifications socially where decisions are being automated.
One pressing ethical issue faced in credit scoring with AI involves bias. The performance of an AI system depends heavily on the size of datasets with which training is done. Where these biases are not well-considered at the development and training of AI models, they sometimes result in discriminatory outcomes. For example, specific demographic groups may be systematically prejudiced against, such as racial minorities, women, or others, by previous patterns of discrimination concelled in the data. This gives rise to a market known as carbon trading.
Second, AI credit-scoring models are voracious consumers of alternative data sources: social media activity, online behavior, even geolocation. These additional data points can enhance an estimate of a person's creditworthiness. But they also risk introducing new forms of bias. Some individuals do not leave an online footprint through social media platforms or reside in given locations, thus forming unconscious biases against them. This again begs questions about reliance on alternative data that might be less accurate or relevant for credit decisions, thus yielding unfair outcomes.
Another important ethical concern has to do with the lack of transparency of AI algorithms. Whereas traditional credit-score models are generally built on understandable and fairly clear criteria, including income, credit history, and debt-to-income ratio, AI models are often complex and hard to interpret. As these AI systems become increasingly "black box," citizens are less and less able to understand how their credit scores have been calculated and to appeal against decisions with which they disagree. Where there is no transparency, trust in the financial system, as well as consumer confidence, will be undermined when people feel they are subject to a judgment against criteria they either don't understand or can't control.
Another key issue in the realm of AI-driven credit scoring has to do with privacy. Large amounts of personal information in training AI models raise significant collection, storage, and usage questions. People may have no idea how their data is used in credit-scoring processes and may, in fact, be having their privacy rights violated. This will continue to exacerbate the risk of data breach and unauthorized access from various sources, as sensitive information will be at risk of malicious actors. Making sure that data is treated securely, transparently, and without any arbitrariness is key to gaining consumer confidence and protecting personal privacy.
The broader social context within which AI credit scoring operates is considered next. With AI increasingly being used in decision-making in finance, there is a likelihood that these systems will further inflate existing inequalities and pad systemic biases. For example, those who have traditionally been at the behest of marginalization from the financial system will have it even more difficult to get credit if the AI models are disproportionately penalizing them. This will further lead to a vicious circle of exclusion whereby those people who need financial services most will be systematically excluded from access. Also, automation in credit decisions may further reduce space for human discretion and oversight, thereby restricting the possibility of considering individual circumstances and applying more inflexible impersonal decision-making processes.
However, this ethical issue calls for an approach from many dimensions:. They will need to ensure their algorithms are designed in such a way to reduce bias and engender fair opportunities for credit access. It will include very conscious choices in data used for training, besides regular auditing and renewing of models of AI that could lead to emerging biases. Second, which would be transparency as to how AI systems work and how they reach credit decisions; this will make consumers understand, if possible, the challenging outcome of such a system.
Subscribe to my newsletter
Read articles from Finance Freak directly inside your inbox. Subscribe to the newsletter, and don't miss out.
Written by
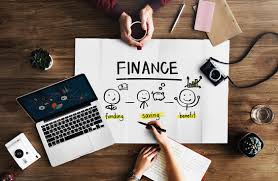