The Role of Machine Learning in Data Science: A Comprehensive Guide

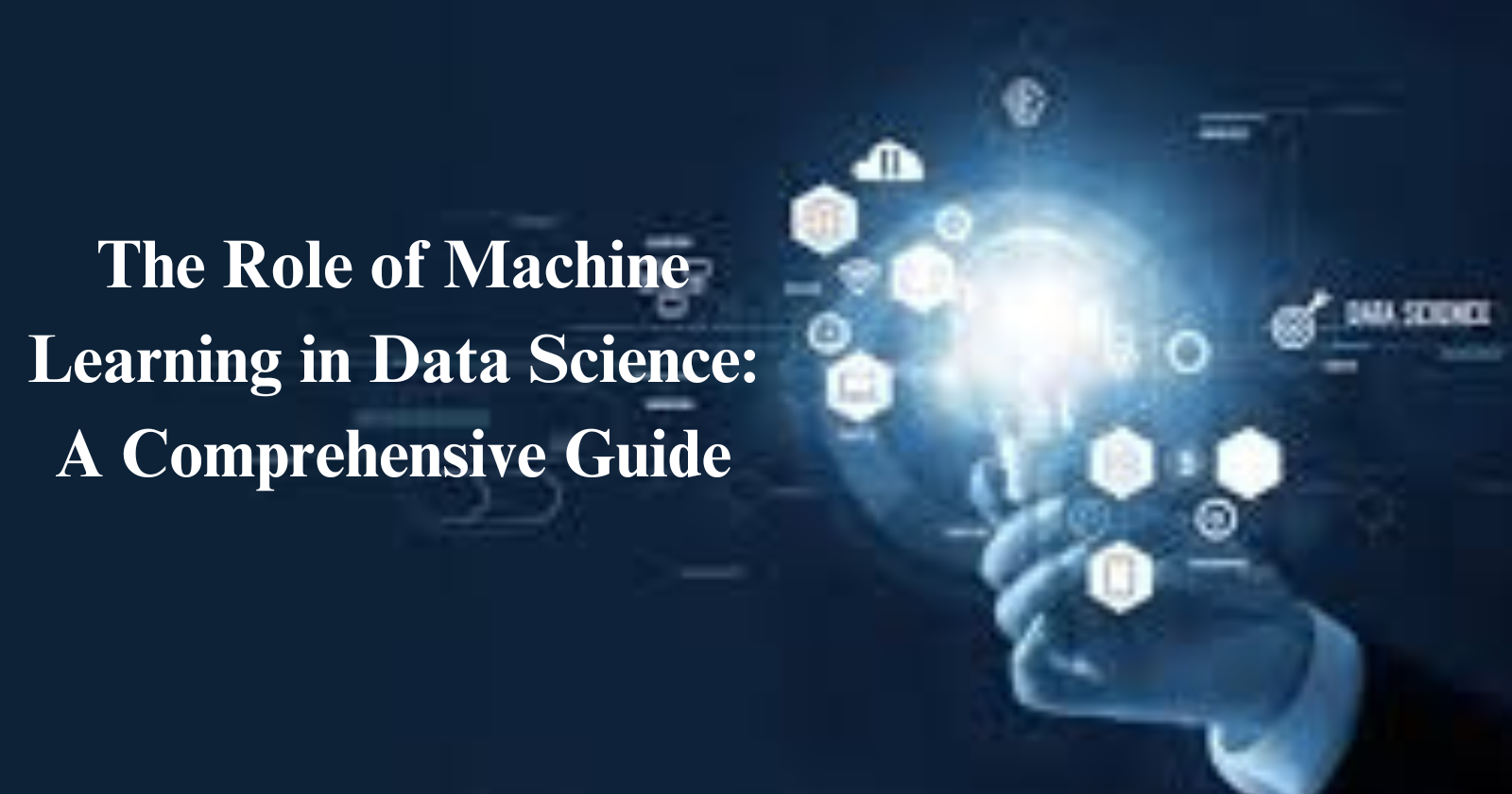
In the realm of data science, machine learning has emerged as a pivotal component, driving innovation and enhancing the capabilities of data analysis. As organizations increasingly rely on data to make informed decisions, the integration of machine learning (ML) into data science practices has become essential. This comprehensive guide explores the role of machine learning in data science, its impact on various industries, and how it shapes the future of data-driven insights.
Understanding Machine Learning and Data Science
To appreciate the role of machine learning in data science, it’s important to understand both fields individually. Data science involves the extraction of knowledge and insights from structured and unstructured data. It encompasses a range of techniques, including data mining, statistical analysis, and data visualization, to uncover patterns and inform decision-making.
Machine learning, on the other hand, is a subset of artificial intelligence (AI) that focuses on the development of algorithms that enable computers to learn from and make predictions based on data. ML models improve their performance over time as they are exposed to more data, allowing them to identify patterns and make decisions with increasing accuracy.
The Alliance Between Data Science and Machine Learning
Machine learning and data science are intrinsically linked. While data science provides the tools and methodologies for analyzing data, machine learning enhances these processes by automating and optimizing data analysis. Here’s how the synergy between these two fields unfolds:
Enhanced Predictive Analytics: Machine learning algorithms excel in predictive analytics, which is a core component of data science. By training models on historical data, machine learning can forecast future trends and behaviors with remarkable precision. This capability is invaluable for industries such as finance, healthcare, and retail, where predicting customer behavior, market trends, and disease outbreaks can lead to significant strategic advantages.
Automation of Data Processing: Data science often involves handling large volumes of data, which can be time-consuming and complex. Machine learning algorithms can automate data processing tasks, such as data cleaning, transformation, and feature selection. This automation not only speeds up the analysis but also reduces the likelihood of human error, ensuring more accurate and reliable results.
Advanced Pattern Recognition: One of the most powerful applications of machine learning in data science is its ability to recognize complex patterns and correlations within data. Traditional statistical methods may struggle with high-dimensional or unstructured data, but machine learning algorithms, such as neural networks and clustering techniques, can identify intricate patterns that are not immediately apparent. This capability enables data scientists to uncover hidden insights and make data-driven decisions with greater confidence.
Personalization and Recommendation Systems: Machine learning plays a crucial role in developing personalized experiences and recommendation systems. By analyzing user behavior and preferences, ML models can deliver tailored recommendations for products, content, and services. This application is widely used in e-commerce platforms, streaming services, and social media, where personalized recommendations enhance user engagement and satisfaction.
Real-Time Data Analysis: In today’s fast-paced world, the ability to analyze data in real-time is essential for timely decision-making. Machine learning algorithms can process streaming data and provide real-time insights, allowing organizations to respond quickly to changing conditions. This capability is particularly valuable in industries such as finance, where rapid analysis of market data can lead to more informed trading decisions.
Practical Applications Across Industries
The merging of machine learning with data science holds significant potential for revolutionizing many industries. Here is an overview at some of the main applications:
Healthcare: Machine learning algorithms are used to analyze medical data, predict patient outcomes, and assist in diagnosing diseases. For example, ML models can analyze medical imaging data to detect anomalies, such as tumors, with high accuracy. Additionally, predictive models can help identify patients at risk of developing chronic conditions, enabling early intervention and personalized treatment plans.
Finance: In the financial sector, machine learning is employed for fraud detection, algorithmic trading, and risk management. ML models can analyze transaction patterns to identify suspicious activities and prevent fraudulent transactions. Algorithmic trading strategies use machine learning to analyze market data and execute trades based on predictive signals, enhancing trading efficiency and profitability.
Retail: Retailers leverage machine learning for inventory management, demand forecasting, and customer segmentation. ML algorithms can predict product demand based on historical sales data and external factors, optimizing inventory levels and reducing stockouts. Customer segmentation models help retailers understand customer preferences and tailor marketing strategies to specific segments, improving customer engagement and sales.
Manufacturing: Machine learning is used in predictive maintenance, quality control, and process optimization within the manufacturing industry. Machine learning models can assess sensor data from machines to predict equipment breakdowns and schedule maintenance, thereby minimizing downtime and expenses. Quality control systems use machine learning to detect defects in products and ensure consistent quality.
The Future of Machine Learning in Data Science
As technology continues to advance, the role of machine learning in data science is expected to grow even more significant. Innovations in areas such as deep learning, reinforcement learning, and explainable AI will further enhance the capabilities of machine learning models. Additionally, the integration of machine learning with other emerging technologies, such as edge computing and the Internet of Things (IoT), will create new opportunities for data-driven insights and automation.
Data scientists will need to stay abreast of these developments and continuously refine their skills to leverage the full potential of machine learning. Collaboration between data scientists and machine learning engineers will be crucial in developing and deploying effective ML solutions that drive business value and innovation.
Conclusion
Machine learning has become an indispensable tool within the field of data science, offering advanced capabilities for predictive analytics, pattern recognition, and real-time data analysis. Its integration into data science practices has transformed how organizations harness the power of data, driving innovation and enabling more informed decision-making. As the field continues to evolve, the synergy between machine learning and data science will undoubtedly shape the future of data-driven insights and technological advancement. For those interested in diving deeper into these concepts, a Data Science course in Delhi, Noida, Greater Noida, Gurgaon, Faridabad and other cities in India can provide valuable knowledge and hands-on experience in leveraging machine learning to its fullest potential.
Subscribe to my newsletter
Read articles from kanika chauhan directly inside your inbox. Subscribe to the newsletter, and don't miss out.
Written by
