#AISprint Multimodal-verse: I - Intro to the Multimodal-Verse

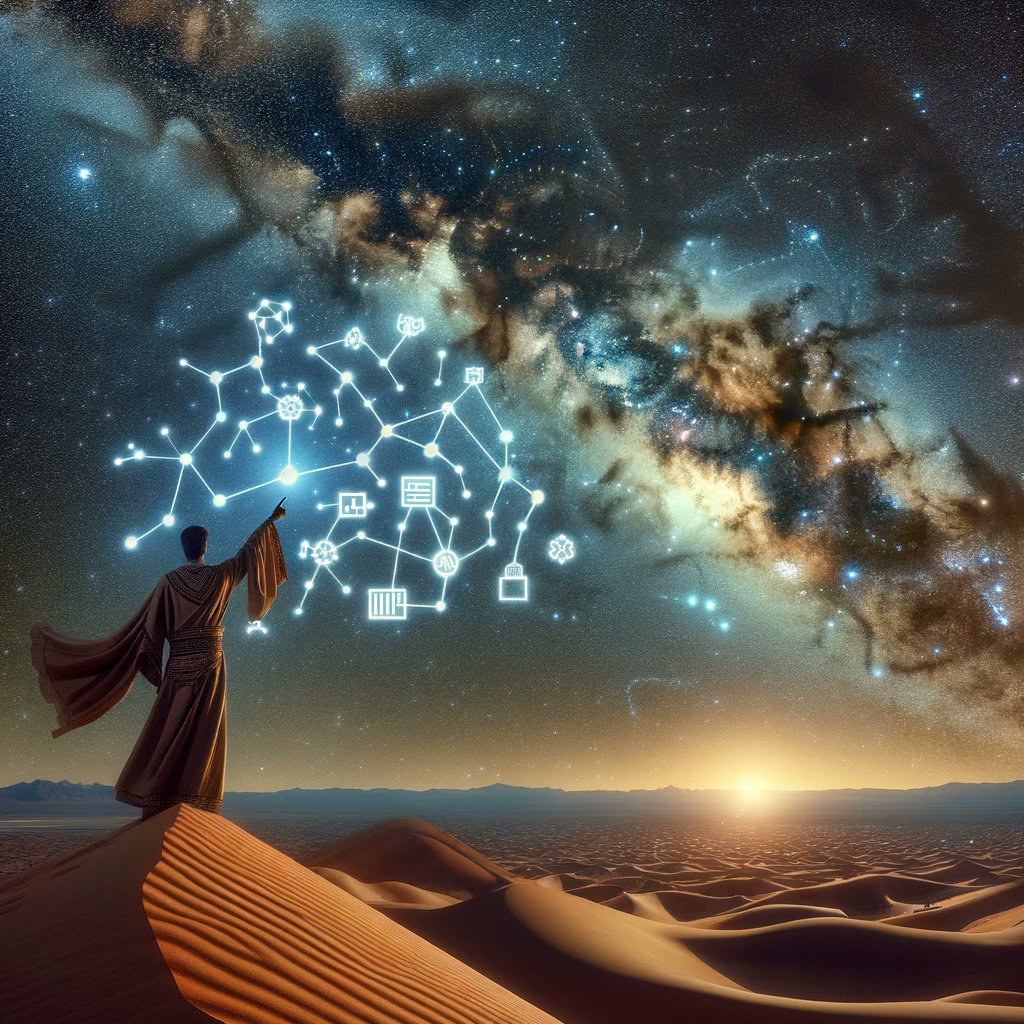
Hey there, AI adventurer!
Ready to step into the wild world of multimodality? Buckle up, because we're about to take your AI knowledge from "meh" to "mind-blowing"!
First things first: What's this multimodal business all about?
Picture this: You're scrolling through your social media feed. You see a meme with a picture and a caption.
Your brain instantly processes both the image and the text, combining them to understand the joke. That, my friend, is multimodality in action!
In the AI world, multimodality is all about combining different types of data or "modalities" - like text, images, audio, or video - to create smarter, more human-like AI systems. It's like giving your AI superpowers!
Let's break it down with some cool concepts:
Plato's Cave: The OG Multimodal Thinker
Remember Plato's allegory of the cave?
If not, here's the TL;DR: Imagine prisoners chained in a cave, only able to see shadows on a wall. They think those shadows are reality. But when one escapes and sees the real world, they realize how limited their perception was.
This is like unimodal AI models - they're stuck looking at shadows (one type of data), while multimodal models get to experience the full, glorious reality! (seeing, smelling, and even tasting the reality)
Unimodal AI models are like that friend who's really good at one thing but clueless about everything else. They might be text wizards or image gurus, but they're missing out on the bigger picture.
Unique Information in Different Modalities: The Spice of (AI) Life
Let's take a stroll through some different ways we take in information and how we use it - you know, like how our brains make sense of the world around us. It's pretty cool when you think about it!
Starting with good old text, it's our go-to for spelling things out clearly and getting into those big, abstract ideas. Like, imagine trying to explain quantum physics without writing it down - yikes! But text has its limits too. Ever had a friend send you a message that just says "I'M FINE" in all caps? You know they're probably not fine, but you can't hear the exasperation in their voice or see the eye roll.
Now, pictures - they're worth a thousand words, right? Images are awesome for showing us how things look and fit together. Think about trying to assemble IKEA furniture with just written instructions. The diagrams save us from ending up with a chair that looks more like abstract art!
Here's a wild one - the thermal spectrum. It's like having superhero vision, showing us heat patterns we can't normally see. Imagine being able to spot a warm-blooded critter hiding in the bushes at night, or finding where your house is leaking heat in winter. Engineers and doctors use this all the time, like checking for hotspots in electrical systems or looking for inflammation in the body.
Audio is where things get really personal. It's not just about hearing words; it's about feeling them. Remember that example of your friend yelling? In a voice message, you'd hear the frustration, maybe even a bit of a voice crack. That's way different from seeing "I'M ANGRY" typed out. Audio lets us pick up on all those little cues - the excitement in someone's voice when they're talking about their passion project, or the soothing tones of your favorite chill-out playlist.
Video is like the superhero of information - it's got visuals and sound working together. It's perfect for when you need to see how something moves or changes over time. Think about learning a new dance move - reading about it? Tricky. Seeing a picture? Better. But watching a video where you can see and hear the instructor? Now we're talking!
All these different ways of taking in info work together to give us a fuller picture of what's going on. It's like having a toolbox where each tool has its own special job, but when you use them all together, you can build something amazing. Pretty neat how our brains juggle all this stuff, huh?
And you know what's even cooler? By combining these modalities, we can create AI systems that understand the world more like humans do. It's like giving your AI a pair of glasses, a hearing aid, and a really good book all at once! This multi-modal approach brings us one step closer to developing AI that can perceive and interpret the world with the same richness and complexity that we do. Imagine an AI that can not only read a recipe, but also watch a cooking video, listen to the sizzle of the pan, and even detect when something's starting to burn - now that's a kitchen assistant I'd want on my team cooking those Tajines!
Glimpse on Multimodality in action in the present/future world:
Multimodal AI isn't just a cool party trick. It's revolutionizing fields in ways that affect our daily lives. Let's break it down.
Healthcare is getting a major upgrade thanks to multimodal AI. Imagine your doctor having a super-smart assistant that can look at your medical records, analyze your X-rays, and even process data from wearable devices - all at once. This AI can spot patterns and make connections that might be missed otherwise. It's like having a whole team of specialists working together to give you the best possible care. The result? More accurate diagnoses, personalized treatment plans, and potentially catching health issues before they become serious problems.
When it comes to autonomous vehicles, multimodal AI is literally driving the future. These smart cars aren't just using one type of sensor - they're combining data from cameras, LiDAR (that's like radar, but with lasers), GPS, and more. It's as if the car has eyes, ears, and an excellent sense of direction all working together. This fusion of data helps the vehicle understand its environment more completely, making split-second decisions to navigate safely. It's not just about getting from A to B; it's about making the journey as safe as possible for everyone on the road.
Everyone saw the virtual assistants announced by Google and OpenAI, cool right? Well, virtual assistants are getting a whole lot smarter too. Gone are the days of simple voice commands. Multimodal AI is helping create assistants that can understand context, pick up on visual cues, and interact more naturally. Imagine talking to your smart home system while cooking, and it can see that your hands are full, hear the sizzle of the pan, and automatically set a timer without you having to ask. It's like having a helpful friend in the room who just gets what you need.
Lastly, content moderation is becoming more effective and nuanced with multimodal AI. In today's digital world, harmful content isn't limited to just text. By analyzing text, images, and videos together, AI can better understand context and nuance. This means it can more accurately identify things like hate speech, misinformation, or inappropriate content across different formats. It's like having a really smart, really fast team of moderators working 24/7 to keep online spaces safer for everyone.
Too Much philosophy, let's Math! just a bit.
Too much philosophy for you? No problem! Let's speak Math and whip up a delicious info-theory smoothie. It's like the nutritional science of data, but way cooler!
Imagine each modality as a different food group in your AI diet. Here's our menu:
Text: Your proteins, are the building blocks of information. It's like chicken breast for your AI - lean, mean, and full of explicit facts.
Images: The carbs of the data world. They give you that quick energy boost of visual information, helping you picture things instantly.
Thermal images: Think of these as your healthy fats. They might seem extra, but they provide that crucial layer of information about heat and energy that you can't get elsewhere.
Depth Images: These are your vitamins and minerals, adding that extra dimension (literally) to your AI's understanding. They're like the spinach of your data diet - packed with spatial goodness!
Now, the task you're trying to solve? That's like your specific dietary need. Sometimes, you can get by on just protein shakes (hello, text-only models!). But for optimal health - or in our case, top-notch AI performance - you often need a balanced diet. That's where our multimodal approach comes in, like a perfectly planned meal prep!
Here's where it gets juicy (pun intended):
Information Overlap: Different modalities often contain overlapping information. It's like getting vitamin C from both oranges and bell peppers. Your AI might learn about an object's shape from both an image and a depth map. This redundancy? It's not a waste - it's reinforcement!
Unique Information: Each modality brings its own special flavor to the table. Text might give you the name of a dish, images show you how it looks, thermal images reveal how it's cooked, and depth images let you appreciate its texture. It's like how you can only get certain omega-3s from fish - some info is modality-exclusive.
Synergistic Information: This is where the magic happens! Combine modalities, and suddenly, 1+1=3. A sarcastic text message + an audio clip of the tone = understanding the true meaning. It's like how calcium and vitamin D work together for stronger bones. In multimodal AI, this synergy can lead to insights greater than the sum of its parts.
Task-Relevant Information: The secret sauce is extracting what's most relevant to your task. It's like customizing your diet for specific fitness goals. Want to build muscle? Up the protein. Training an AI to recognize emotions? Maybe prioritize facial expressions (images) and tone of voice (audio) over thermal data.
By mixing multiple modalities, we're essentially increasing the mutual information between our input buffet and the desired output. It's like expanding our menu to make sure we're getting all the nutrients (information) we need for a specific health goal (task).
So there you have it - multimodal AI, served up with a side of nutritional metaphors and a sprinkle of information theory. Bon appétit, data scientists!
The Bottom Line
Multimodality is the secret sauce that's taking AI to the next level. By breaking free from the limitations of unimodal approaches, we're creating AI systems that can see the world more like we do - in all its complex, multifaceted glory.
So, the next time you effortlessly understand a meme or instantly recognize your friend's sarcastic tone in a voice message, remember: that's multimodal processing in action. And now, we're teaching machines to do the same!
Stay tuned for our next post, where we'll dive deeper into why going multimodal is not just cool, but crucial for the future of AI. Until then, keep your eyes, ears, and mind open to the multimodal world around you, and don't stop sharing those memes.
Acknowledgments
Google AI/ML Developer Programs team supported this work by providing Google Cloud Credit.
References
I will try to use the same numbers for citations for the rest of the blogs.
Resources
- Plato’s Cave: https://nofilmschool.com/allegory-of-the-cave-in-movies
Papers and Theses
Le-Khac, P. H., Healy, G., & Smeaton, A. F. (2020). Contrastive representation learning: A framework and review. IEEE Access, 8, 193907–193934.
Jia, C., Yang, Y., Xia, Y., Chen, Y., Parekh, Z., Pham, H., Le, Q., Sung, Y., Li, Z., & Duerig, T. (2021). Scaling up Visual and Vision-Language representation learning with noisy text supervision. International Conference on Machine Learning, 4904–4916. http://proceedings.mlr.press/v139/jia21b/jia21b.pdf
Radford, A., Kim, J. W., Hallacy, C., Ramesh, A., Goh, G., Agarwal, S., Sastry, G., Askell, A., Mishkin, P., Clark, J., Krueger, G., & Sutskever, I. (2021). Learning transferable visual models from natural language supervision. arXiv. https://arxiv.org/abs/2103.00020
Zhai, X., Mustafa, B., Kolesnikov, A., & Beyer, L. (2023, October). Sigmoid loss for language image pre-training. In 2023 IEEE/CVF International Conference on Computer Vision (ICCV) (pp. 11941-11952). IEEE. https://doi.org/10.1109/ICCV51070.2023.01100
Li, S., Zhang, L., Wang, Z., Wu, D., Wu, L., Liu, Z., Xia, J., Tan, C., Liu, Y., Sun, B., & Stan Z. Li. (n.d.). Masked modeling for self-supervised representation learning on vision and beyond. In IEEE [Journal-article]. https://arxiv.org/pdf/2401.00897
Jia, C., Yang, Y., Xia, Y., Chen, Y., Parekh, Z., Pham, H., Le, Q., V., Sung, Y., Li, Z., & Duerig, T. (2021, February 11). Scaling up Visual and Vision-Language representation learning with noisy text supervision. arXiv.org. https://arxiv.org/abs/2102.05918
Bachmann, R., Kar, O. F., Mizrahi, D., Garjani, A., Gao, M., Griffiths, D., Hu, J., Dehghan, A., & Zamir, A. (2024, June 13). 4M-21: An Any-to-Any Vision model for tens of tasks and modalities. arXiv.org. https://arxiv.org/abs/2406.09406
Bao, H., Dong, L., Piao, S., & Wei, F. (2021, June 15). BEIT: BERT Pre-Training of Image Transformers. arXiv.org. https://arxiv.org/abs/2106.08254
Balestriero, R., Ibrahim, M., Sobal, V., Morcos, A., Shekhar, S., Goldstein, T., Bordes, F., Bardes, A., Mialon, G., Tian, Y., Schwarzschild, A., Wilson, A. G., Geiping, J., Garrido, Q., Fernandez, P., Bar, A., Pirsiavash, H., LeCun, Y., & Goldblum, M. (2023, April 24). A cookbook of Self-Supervised Learning. arXiv.org. https://arxiv.org/abs/2304.12210
Zadeh, A., Chen, M., Poria, S., Cambria, E., & Morency, L. (2017, July 23). Tensor Fusion Network for Multimodal Sentiment Analysis. arXiv.org. https://arxiv.org/abs/1707.07250
Tian, Y., Sun, C., Poole, B., Krishnan, D., Schmid, C., & Isola, P. (2020, May 20). What makes for good views for contrastive learning? arXiv.org. https://arxiv.org/abs/2005.10243
Huang, Y., Du, C., Xue, Z., Chen, X., Zhao, H., & Huang, L. (2021, June 8). What Makes Multi-modal Learning Better than Single (Provably). arXiv.org. https://arxiv.org/abs/2106.04538
Nagrani, A., Yang, S., Arnab, A., Jansen, A., Schmid, C., & Sun, C. (2021, June 30). Attention bottlenecks for multimodal fusion. arXiv.org. https://arxiv.org/abs/2107.00135
Liu, Z., Shen, Y., Lakshminarasimhan, V. B., Liang, P. P., Zadeh, A., & Morency, L. (2018, May 31). Efficient Low-rank Multimodal Fusion with Modality-Specific Factors. arXiv.org. https://arxiv.org/abs/1806.00064
Wang, X., Chen, G., Qian, G., Gao, P., Wei, X., Wang, Y., Tian, Y., & Gao, W. (2023, February 20). Large-scale Multi-Modal Pre-trained Models: A comprehensive survey. arXiv.org. https://arxiv.org/abs/2302.10035
Wang, W., Bao, H., Dong, L., Bjorck, J., Peng, Z., Liu, Q., Aggarwal, K., Mohammed, O. K., Singhal, S., Som, S., & Wei, F. (2022, August 22). Image as a Foreign Language: BEIT Pretraining for all Vision and Vision-Language tasks. arXiv.org. https://arxiv.org/abs/2208.10442
Liang, P. P. (2024, April 29). Foundations of multisensory artificial Intelligence. arXiv.org. https://arxiv.org/abs/2404.18976
Huang, S., Pareek, A., Seyyedi, S., Banerjee, I., & Lungren, M. P. (2020). Fusion of medical imaging and electronic health records using deep learning: a systematic review and implementation guidelines. Npj Digital Medicine, 3(1). https://doi.org/10.1038/s41746-020-00341-z
Subscribe to my newsletter
Read articles from Taha Bouhsine directly inside your inbox. Subscribe to the newsletter, and don't miss out.
Written by
