Integrating AI Analytics Tool with Existing Data Systems

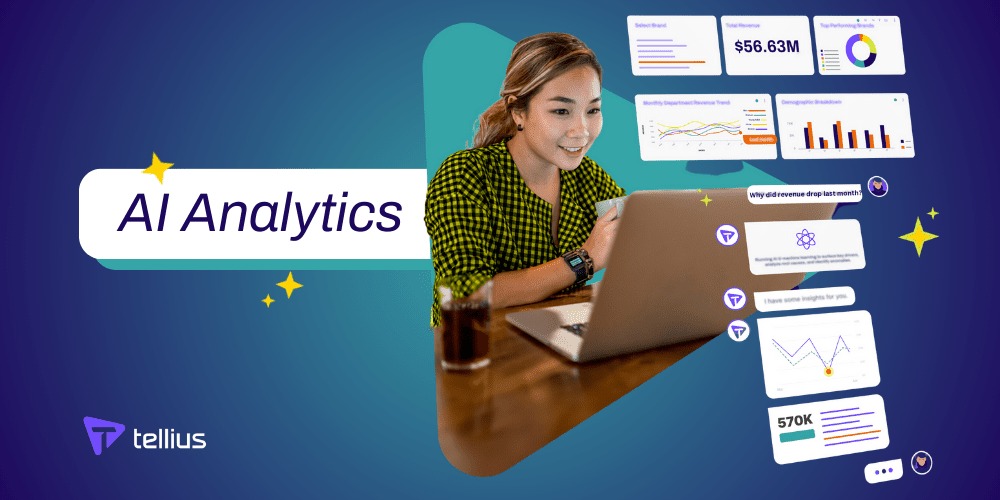
In the era of big data, businesses are continually seeking innovative ways to extract actionable insights and drive growth. One of the most transformative advancements in this quest is the use of AI analytics tools. These tools leverage artificial intelligence to analyze large datasets, uncover patterns, and provide predictive insights. However, for organizations to fully capitalize on these benefits, integrating AI analytics tools with existing data systems is essential. This integration is not merely a technical challenge but a strategic opportunity to enhance decision-making and operational efficiency.
1. Understanding the Integration Process
Integrating an AI analytics tool with your existing data systems involves several key steps. First, you need to ensure that the AI tool can seamlessly connect with your current data sources, such as databases, data warehouses, and cloud storage solutions. This typically requires configuring APIs (Application Programming Interfaces) or data connectors that allow the AI tool to access and interact with your data.
The integration process also involves data cleansing and transformation. AI analytics tools rely on high-quality data to generate accurate insights. Therefore, it’s crucial to clean and format your existing data to ensure it’s consistent and ready for analysis. This step helps in avoiding potential issues like incomplete data or discrepancies that could affect the AI tool's performance.
2. Aligning AI Analytics with Business Objectives
A successful integration requires aligning the AI analytics tool with your business objectives. This means understanding what specific insights or outcomes you aim to achieve with the AI tool. Whether it's improving customer segmentation, forecasting sales, or optimizing supply chain operations, your integration strategy should focus on these goals.
Work closely with stakeholders across different departments to identify their needs and expectations. This collaboration ensures that the AI analytics tool is configured to address real business challenges and deliver relevant insights. For example, if your goal is to enhance marketing efforts, integrate the AI tool with customer data systems to analyze buying patterns and campaign effectiveness.
3. Ensuring Data Security and Compliance
Data security and compliance are critical considerations when integrating AI analytics tools. Ensure that your integration adheres to data protection regulations, such as GDPR or CCPA, and that robust security measures are in place. This includes encrypting data during transit and at rest, implementing access controls, and regularly monitoring for potential breaches.
Moreover, establish clear protocols for data governance to maintain data integrity and accuracy. This involves setting up rules for data access, data sharing, and data quality management to ensure that the AI analytics tool operates within a secure and compliant environment.
4. Testing and Validation
Before fully deploying the AI analytics tool, conduct thorough testing to validate its performance and accuracy. This includes running pilot tests with sample data to evaluate how well the tool integrates with your existing systems and whether it generates reliable insights. Testing helps identify any issues or discrepancies that need to be addressed before a full-scale rollout.
Gather feedback from end-users who interact with the AI tool to ensure it meets their needs and expectations. This feedback is invaluable for making adjustments and improvements, ensuring that the tool delivers maximum value.
5. Training and Support
Effective integration also involves training your team to use the AI analytics tool effectively. Provide comprehensive training sessions that cover the tool’s features, functionalities, and best practices. Ensure that your team understands how to interpret the insights generated by the AI tool and how to leverage these insights for decision-making.
Additionally, establish a support system for addressing any technical issues or questions that may arise post-integration. This support helps ensure that the AI analytics tool continues to function smoothly and provides ongoing value to your organization.
Integrating an AI analytics tool with existing data systems is a strategic move that can significantly enhance your organization’s ability to make data-driven decisions. By carefully managing the integration process, aligning the tool with business objectives, ensuring data security, and providing adequate training and support, you can unlock the full potential of AI analytics. This integration not only streamlines operations but also empowers your organization with deeper insights and a competitive edge in the marketplace.
Subscribe to my newsletter
Read articles from Tellius Usa directly inside your inbox. Subscribe to the newsletter, and don't miss out.
Written by
