Rethinking "Hallucinations" in AI: Why We Need New Terminology for AI Errors
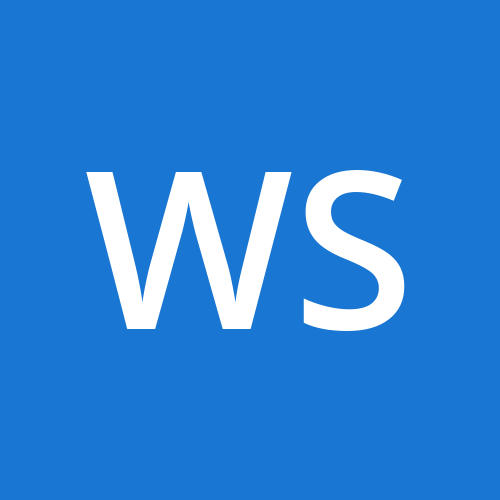
September 21, 2023
As artificial intelligence continues to integrate into our daily lives, the language we use to describe its behaviors becomes increasingly important. One term that has gained traction is "hallucination," used to describe instances where AI models generate outputs that don't align with reality. But is this term accurate, or does it inadvertently anthropomorphize AI systems by attributing human-like cognitive processes they don't possess?
The Problem with "Hallucination"
In human psychology, a hallucination refers to a sensory experience of something that isn't present, often linked to mental health conditions. Applying this term to AI suggests that models experience perceptions similar to humans, which isn't the case. AI models, particularly large language models (LLMs), generate outputs based on patterns in data, not conscious thought or sensory experiences.
LLMs operate by predicting the next word in a sequence, relying on statistical probabilities derived from their training data. When they produce information that doesn't correspond to factual reality, it's not due to a misperception but rather a reflection of data limitations or biases within their training sets.
Why Terminology Matters
Clarity and Understanding
Using terms like "hallucination" can confuse users about the nature of AI outputs. It blurs the line between human cognition and machine processing, leading to misconceptions about how AI functions. Clear, accurate terminology helps set appropriate expectations and fosters a better understanding of AI capabilities and limitations.
Focus on Technical Issues
Describing AI inaccuracies with precise terms directs attention to the underlying technical problems. Recognizing outputs as "errors" or "fabrications" highlights issues such as data quality, model architecture, or training methodologies that need to be addressed to improve performance.
Avoiding Anthropomorphism
Anthropomorphizing AI can lead to unrealistic expectations or unwarranted fears about technology. By refraining from using human-centric terms, we maintain a clear distinction between human and machine intelligence, which is crucial for ethical considerations and responsible AI development.
Enhancing Communication
Accurate terminology facilitates better communication among researchers, developers, and users. It ensures that discussions about AI are grounded in reality, which is essential for collaboration, education, and policy-making.
Alternative Terminology
To better describe AI outputs that don't align with reality, consider using terms rooted in technical accuracy:
Fabrication: Indicates that the AI has generated information without a factual basis. It emphasizes the creation of unsupported content.
Error: A straightforward term denoting that the output is incorrect or flawed.
Inaccuracy: Focuses on the lack of correctness in the information provided by the AI.
Misalignment: Suggests a deviation from the intended or accurate output, highlighting a discrepancy between the AI's response and factual information.
Some have proposed the term "confabulation," but it also originates from human cognitive processes related to memory errors and may carry similar anthropomorphic implications.
Moving Forward with Precision
By adopting terminology that accurately reflects AI processes, we can:
Improve Model Development: Address specific issues within AI models by identifying errors without anthropomorphic bias.
Educate Users: Provide clearer explanations to users, helping them understand AI limitations and reducing the spread of misinformation.
Promote Ethical AI: Encourage responsible use and development of AI technologies by avoiding language that misrepresents AI capabilities.
Conclusion
The language we use shapes our understanding. As AI continues to evolve, it's crucial to describe its behaviors accurately. Moving away from terms like "hallucination" in favor of "fabrication," "error," or "misalignment" helps demystify AI outputs and fosters a more productive dialogue about improving these systems.
Let's continue the conversation about AI with clarity and precision, ensuring that we build and use these technologies responsibly.
Subscribe to my newsletter
Read articles from William Stetar directly inside your inbox. Subscribe to the newsletter, and don't miss out.
Written by
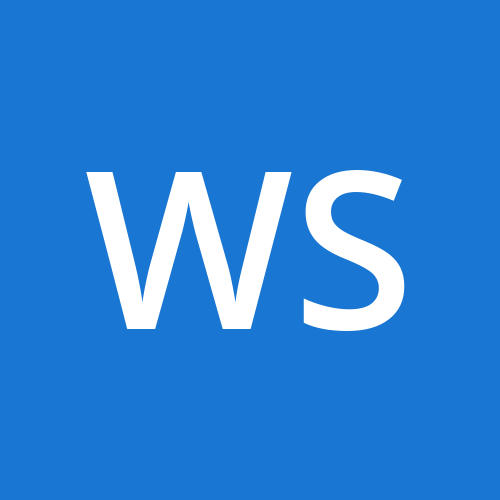