Data Science in Conflict Zones: Forecasting and Mitigation of Humanitarian Crisis
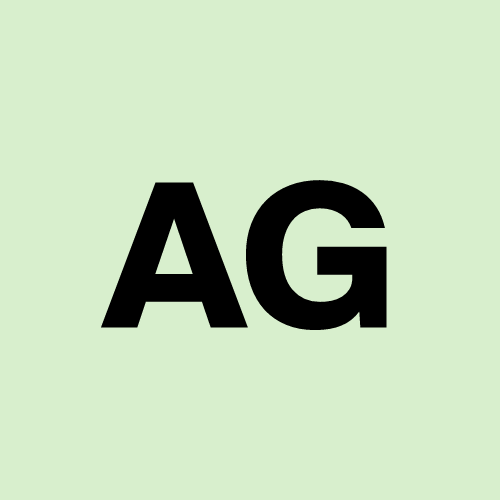
In a globalized society, early identification of and intervention in crises is essential in conflict-affected areas as they cause forced displacements, hunger, and disease outbreaks. These dynamic contexts imply complex problems, and with the ability to process a tremendous amount of information and the identification of concealed trends, data science has become an effective means of tackling these issues. If machine learning, big data analytics, and predictive modelling can be used to give stakeholders in conflict and humanitarian zones access to rich risk information or even predict crises and design interventions that reduce suffering.
Conflict areas – what a complex subject that is!
Conflict areas are unstable areas where the structures of law, physical infrastructure, and governance are destroyed. These populations are usually involved in violent acts, forced displacement, limited access to basic needs, and, most of the time, life-altering psychological trauma. The existing methods of responding to and mitigating such crises in these regions are also inadequate; they entail extensive overreliance on paper-based records, field-based surveillance, and crisis-based responses. However, these methods may be slow and inaccurate and not effective when used in rapidly changing environments. The advent of data science hence offers a better and preemptive way to deal with such challenges.
While information is easy to come by in conflict regions, it remains scattered and unconnected. That is, social media posts, satellite images, and even information gathered from individuals’ mobile phones, articles, and field surveys. The difficulty is in how to combine these various data inputs and how to gain knowledge from them effectively. In this case, data science justifies itself as a field that is capable of analyzing big data on the fly to flag attrition, highlight opportunities, and evaluate threats before turning into large-scale issues.
Predicting Crises: Machine Learning and AI
The specific aspect of data science that underpins its application in conflict zones is the ability to use machine learning (ML) and artificial intelligence (AI) models to forecast where and when conflicts will happen. Such tools are useful in detecting precursors of conflict and humanitarian disasters and enable organizations to act before a conflict gets out of hand.
For instance, an area where data science is making a huge difference is estimating forced displacement. Predictive models can approximate the possibility of massive movements after observing historical trends concerning conflict´s severity, economic climate, or population size. Other examples of specific applications of artificial intelligence include using them to learn about features visible by satellite, such as movements of troops, destruction of buildings or land usage shifts that may be signs of an impending conflict. This information can be integrated with geospatial data, social media analytics, and economic data to predict humanitarian requirements such as famine or epidemics.
These models are after adjusted as new data is obtained to make the predictions more accurate as time progresses. In addition, intervention strategies that can be implemented can be evaluated by the ML algorithms, information that can be used by humanitarian agencies to allocate their resources better and prepare contingency plans. In using these forecast tools, governments, NGOs, and international human rights organizations, including the United Nations, will be in a better position to determine where and how to allocate aid and resources.
Risk Prevention Based on Quantitative and Qualitative Evidence
To avoid humanitarian catastrophes in areas of conflict, it is necessary to forecast them and be capable of acting upon this forecast. Of course, intervention strategies here are, in essence, defined and informed by the data science approach. Using evidence-based approaches, humanitarian agencies can map out the hotspots of a crisis, focus their efforts, and evaluate the impact of their interventions in real time.
Satellite imagery is a good example of data use when it comes to ensuring that environmental factors that can precipitate a humanitarian crisis are adequately addressed. For example, in areas that are likely to face food shortages, data analysts can study the amount of moisture in the soil, rainfall, and quality of crops to determine when the next famine is likely to occur. With the integration of socioeconomic information like market prices and availability of water, predictive models can assist in the anticipatory deployment of foods and other necessities to spare the organization the agony of a full-blown humanitarian crisis.
Another example of a big data application is tracking the movements of internally displaced persons using data from mobile phones. CDRs from mobile phones also make it possible to identify areas of concentration of the displaced persons and access to basic amenities such as health, food, and shelter. It is thus very helpful in planning for responsive-selective healthcare solutions that may include mobile clinics or feeding stations, for example.
Let me briefly elaborate on how communication has been transformed by data science in areas of conflict. There are also simple things one could do to track tensions through social media and news sources, and the sentiment of the local population can be tracked through the use of natural language processing (NLP) algorithms. These insights help organizations handle complaints, which, if left unresolved, may exacerbate conflict, create culturally sensitive interventions, and facilitate negotiations between two groups in conflict.
Challenges in Implementation
As has been demonstrated, conflict regions can benefit significantly from the application of data science; however, there are constraints to its use. This means that data availability and data quality problems are commonplace in conflict zones as the infrastructure of such places is often developed, and obtaining good data is difficult. However, it has been recognized that in such environments certain ethical issues have to be taken into consideration while dealing with sensitive data. Personal data like mobile phone records are also subject to controversies regarding privacy and consent, especially when the data is being collected from sensitive groups of the population.
Also, worrying things could be possible if data science is overdone, as there is a likelihood that humanitarian work will be dehumanized due to predictions based on numbers. Data science should be used as an aid and in synergy with human rights organizations, field workers, and local communities in conflict zones.
Conclusion
Data science offers a transformative approach to predicting and preventing humanitarian crises in conflict zones. Through predictive modelling, real-time monitoring, and data-driven decision-making, it empowers organizations to anticipate challenges and respond swiftly to emerging threats. However, the ethical and practical difficulties of applying these technologies in conflict zones must be carefully managed. By combining the power of data science with the knowledge and expertise of humanitarian workers on the ground, we can move closer to a future where crises are not only predicted but prevented. For those interested in acquiring the necessary skills to contribute to such efforts, enrolling in a data science course in Chennai provides an excellent opportunity to gain hands-on experience and expertise in this vital field.
Subscribe to my newsletter
Read articles from adarsh gowda directly inside your inbox. Subscribe to the newsletter, and don't miss out.
Written by
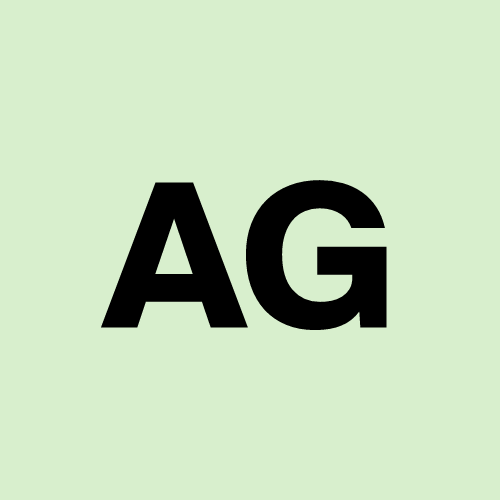