How Can We Ensure Fairness and Eliminate Bias in AI Systems?
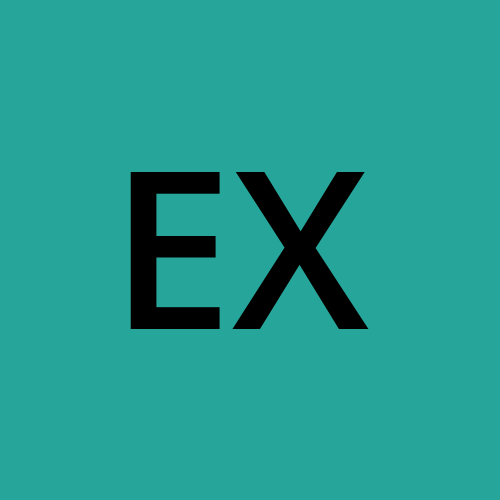
How Can We Ensure Fairness and Eliminate Bias in AI Systems?
As artificial intelligence (AI) continues to evolve and integrate into more aspects of our daily lives, ensuring the fairness and lack of bias in these systems is becoming increasingly important. AI systems are now used to make decisions in areas like healthcare, finance, education, and criminal justice. These decisions have significant impacts on individuals and communities, which raises ethical concerns about bias, discrimination, and fairness. Addressing these challenges is crucial for building trust in AI technology.
In this article, we will explore the sources of bias in AI, discuss the challenges in ensuring fairness, and suggest steps that can be taken to reduce bias in AI systems. For individuals pursuing artificial intelligence training, understanding these aspects is essential to developing responsible and ethical AI technologies.
1. Understanding Bias in AI Systems
To ensure AI systems are fair and unbiased, we must first understand where bias comes from. Bias in AI can stem from two primary sources:
Data Bias: AI systems learn from data. If the training data is biased, the AI system will inherit those biases. For example, if an AI algorithm for hiring is trained on resumes that historically favored men, it may learn to favor male candidates over equally qualified women. This issue of biased data is a significant challenge, as many historical datasets reflect societal inequalities.
Algorithmic Bias: Even when data is relatively unbiased, the algorithms themselves may introduce bias. Some algorithms can prioritize certain variables over others in ways that unintentionally produce unfair outcomes. For example, a credit scoring algorithm might disproportionately favor applicants from wealthier neighborhoods if it weighs certain socio-economic factors too heavily.
Bias in AI systems is a critical topic covered in artificial intelligence training programs, as AI developers must learn to recognize, mitigate, and avoid perpetuating existing societal biases in their designs.
2. Challenges in Ensuring Fairness in AI
Ensuring fairness in AI is not straightforward due to several challenges:
Lack of Diverse Data: Many AI models are trained on data that lacks diversity. For instance, facial recognition systems trained on datasets that contain mostly light-skinned individuals often perform poorly on people with darker skin tones. This can lead to discriminatory outcomes in real-world applications, such as law enforcement or security.
Complexity of Fairness: Fairness is a complex concept that varies depending on the context. What may be considered fair in one domain may be perceived as unfair in another. For example, an AI system that assigns equal weight to every student’s test score may seem fair, but it could overlook socio-economic disparities that affect access to education and learning resources.
Trade-Offs Between Accuracy and Fairness: Developers often face trade-offs between the accuracy of AI systems and their fairness. Improving fairness might sometimes come at the cost of reducing the system's overall accuracy. For example, in predictive policing, if efforts are made to remove bias against specific demographic groups, the predictive power of the model might decrease.
Addressing these challenges requires deep technical understanding and ethical considerations, both of which are emphasized in modern artificial intelligence training programs.
3. Steps to Reduce Bias and Ensure Fairness in AI
Despite these challenges, there are concrete steps that can be taken to ensure AI systems are more fair and unbiased. Here are some strategies:
Use of Diverse and Representative Data: To reduce bias, AI systems must be trained on diverse datasets that reflect a wide range of demographic groups and experiences. This ensures the AI model does not favor one group over another. Data should also be regularly audited for any imbalances or hidden biases before it is used in training.
Bias Detection Tools: Researchers and AI developers are creating bias detection tools that automatically identify and flag biased decisions made by AI systems. These tools can help AI practitioners monitor models and make real-time adjustments to correct for any unfair patterns.
Transparent AI Models: Making AI models more transparent can improve fairness. When the inner workings of an AI system are open for review, it becomes easier to detect bias and hold developers accountable. Explainable AI (XAI) is an emerging field that aims to make AI systems more understandable and interpretable, allowing stakeholders to better assess how decisions are made.
Regular Audits and Testing: Conducting regular audits of AI systems is a critical step in maintaining fairness. AI models should be tested not just during the initial development phase but continuously as they are used in real-world applications. This ensures that models adapt to changing environments without introducing new biases over time.
Ethical AI Development Training: AI developers should receive training in ethical AI development to better understand the social and cultural implications of their work. As part of their artificial intelligence training, developers can learn techniques for bias mitigation, fairness metrics, and the importance of incorporating diverse perspectives during the AI development process.
Conclusion
Ensuring fairness and removing bias in AI systems is a complex but essential goal for the responsible development of artificial intelligence. With AI playing an increasingly significant role in decision-making processes, from hiring to policing, it is crucial that these systems be designed in ways that are fair and equitable.
Understanding the sources of bias, the challenges in achieving fairness, and the strategies to reduce bias is critical for AI developers. By focusing on diverse datasets, transparent algorithms, and regular audits, we can make strides toward building AI systems that serve everyone equally.
For those pursuing artificial intelligence training, the importance of developing ethical, fair, and unbiased AI cannot be overstated. Learning these practices ensures that future AI developers contribute to creating technology that benefits all members of society, without perpetuating existing inequalities.
Subscribe to my newsletter
Read articles from excelrsolution directly inside your inbox. Subscribe to the newsletter, and don't miss out.
Written by
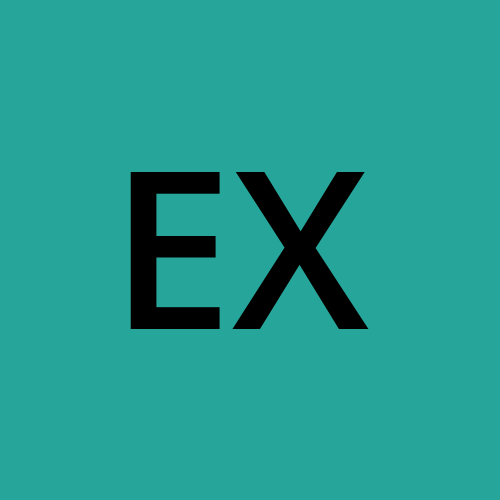