Using Data Science To Combat Fraud: Techniques And Tools For The Modern Age
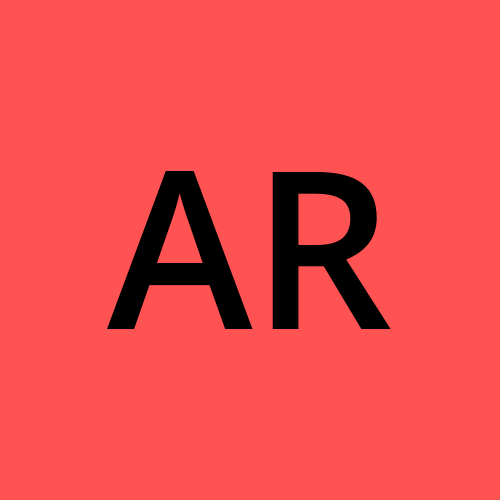
Fraud has been a longstanding issue that has affected both business and personal areas throughout the years. This can be understood in the radical increase of advanced and complex forms of fraud as the world goes digital. Thankfully, data science has become an effective weapon in the fight against fraud, thus providing businesses with a way of defending themselves. Now, we turn to how data science is applied at present to the identification and prevention of fraud, with the techniques and tools that underpin it.
The Role Of Data Science In Fraud Detection
Data science is also known as data analytics, which is the process of modeling large datasets to reveal helpful information. In fraud detection, it is one capability that is universally useful. Almost all fraudulent activities do not produce any blatant clues; however, identifying them may entail hunting for a needle in a haystack. That’s where data science comes to the rescue. Algorithm and machine learning models allow the data scientist to look for similar scenarios in millions of transactions or records on a real-time basis.
One of the skills that are exceptionally useful in the war against fraud is the ability of data science to identify outliers. An example of what might be an anomaly could be a considerable transaction amount compared to the norm, a purchase made in arguably an odd location, or several transactions that could follow one another in a very short time. They may be the first sign of fraud and help businesses act before they experience much more harm.
Fraud Detection Techniques
Of course, data scientists utilize numerous methods to identify fraud. All are important in building practical systems to prevent fraudulent transactions that are likely to occur in organizations. Here are a few of the most important ones:
Anomaly Detection: This technique is at the heart of most fraud detection systems. Through the definition of baseline behavior for users, anomaly detection identifies an irregular activity, such as large purchases or multiple logins from different IPs.
Predictive Modeling: Fraud detection is done by data scientists who develop models that you choose to base their outcomes on past actions. The machine learning algorithms when learned with the historical data can predict the new fraud cases to be prevented in the future. It allows them to always be in a position to dupe the fraudsters.
Network Analysis: Most fraud schemes are connected since the perpetrator uses the same account and modus operandi to carry out the schemes. Such relations are revealed through network analysis that connects the corresponding points within the incident database, discovering negative connections between disparate fraud episodes.
Natural Language Processing (NLP): NLP is particularly helpful when dealing with volumes of unformatted datasets, as are usually encountered in email archives or customer feedback. The con artists always use text messages, whether it is in the employment of fake e-mail addresses or fake claims. NLP can process and detect problems within such data types and, moreover, draw attention to suspicious material.
Tools Used In Fraud Detection
Indeed, most of these techniques require the utilization of multiple tools and technologies among data scientists. Below are some of the most popular tools that have proven essential in the fight against fraud:
Python and R: These programming languages are among the foundational components of data science applications such as fraud detection. For instance, the popular language known as Python has many application libraries to make machine learning models such as Scikit-learn, and to analyze data as illustrated by the Pandas library.
SAS Fraud Management: This tool is actually under development for detecting and monitoring fraud paying activities, especially in commerce, banks, and insurance industries. The huge volumes of data are processed so that results can be available in real-time via machine learning algorithms to flag cases of fraud in SAS.
IBM’s Watson: Watson, famous for its artificial intelligence side, is applied to fraud in industries such as healthcare and finance. It enables organizations to create outstanding data models and detect fraud in real-time but due to the application of artificial intelligence and machine learning.
Tableau: Tableau is often known as a reporting tool although in the process of detecting fraud, it enables businesses to look at patterns that indicate fraud in a graphical way.
Fraud Prevention: Effect Of Data Science
Analyzing fraud is a great idea, but stopping it is even better. Data science enables organizations not only to determine when fraud has taken place, but where and when, it is likely to happen again. Business owners need to know exactly what the fraud perpetrators want to achieve, in order to be ensured that their companies do not fall victim to these scams and manipulations.
For example, risk models can qualify transactions or a particular user action with risk values. Given the strong correlation between certain actions and fraudulent activities, the system can recommend actions based on the identified threats or reject the action altogether. Such preventive measures of fraud have proven helpful in preventing the loss of millions of dollars for various businesses.
Furthermore, with the advancement of technology, there is an advancement in the techniques that data scientist applies. The other way is flexibility; machine learning models become appreciably accurate with each experience they make in the field of fraud detection. The more samples the systems are fed with, the improved their capacity to identify the early clues of fraud.
Why Learning Data Science Is Essential
Like any other social evil, fraud is changing gradually, and so must be the strategies used to counter it. This makes data science one of the most coveted skills that any professional wants to have to work in the field of fraud detection. No matter whether it is a mastering of complicated learning algorithms or the use of data analysis, data science provides the kind of knowledge that is necessary to build the technology that can fight modern fraud effectively.
Prospective learners wishing to advance themselves in this sector benefit from signing up for a data science course. One good opportunity, therefore, would be to take a data science course in Chandigarh, which will help you understand all the fundamental aspects of data science frameworks and methods used in combating fraud. After the completion of the course, you are in a perfect position to fight fraud in the modern world.
Final Thoughts
Fraud is always lurking around, and with the help of data science, we now know how to fight it to our advantage better than before. Some of the methods that can help alleviate fraud include anomalous pattern analysis, assessment, and network approaches. In the midst of continued technological progression, more sophisticated strategies for deterring fraud efforts will emerge, which would make data science an effective weapon against fraud.
Subscribe to my newsletter
Read articles from Arthur directly inside your inbox. Subscribe to the newsletter, and don't miss out.
Written by
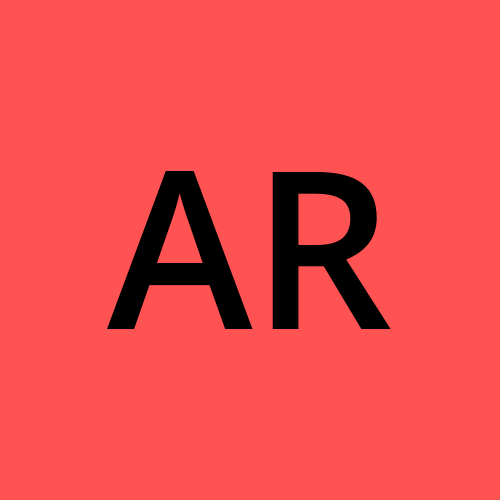