Why should we care about AI Agents instead of a single prompted LLM?

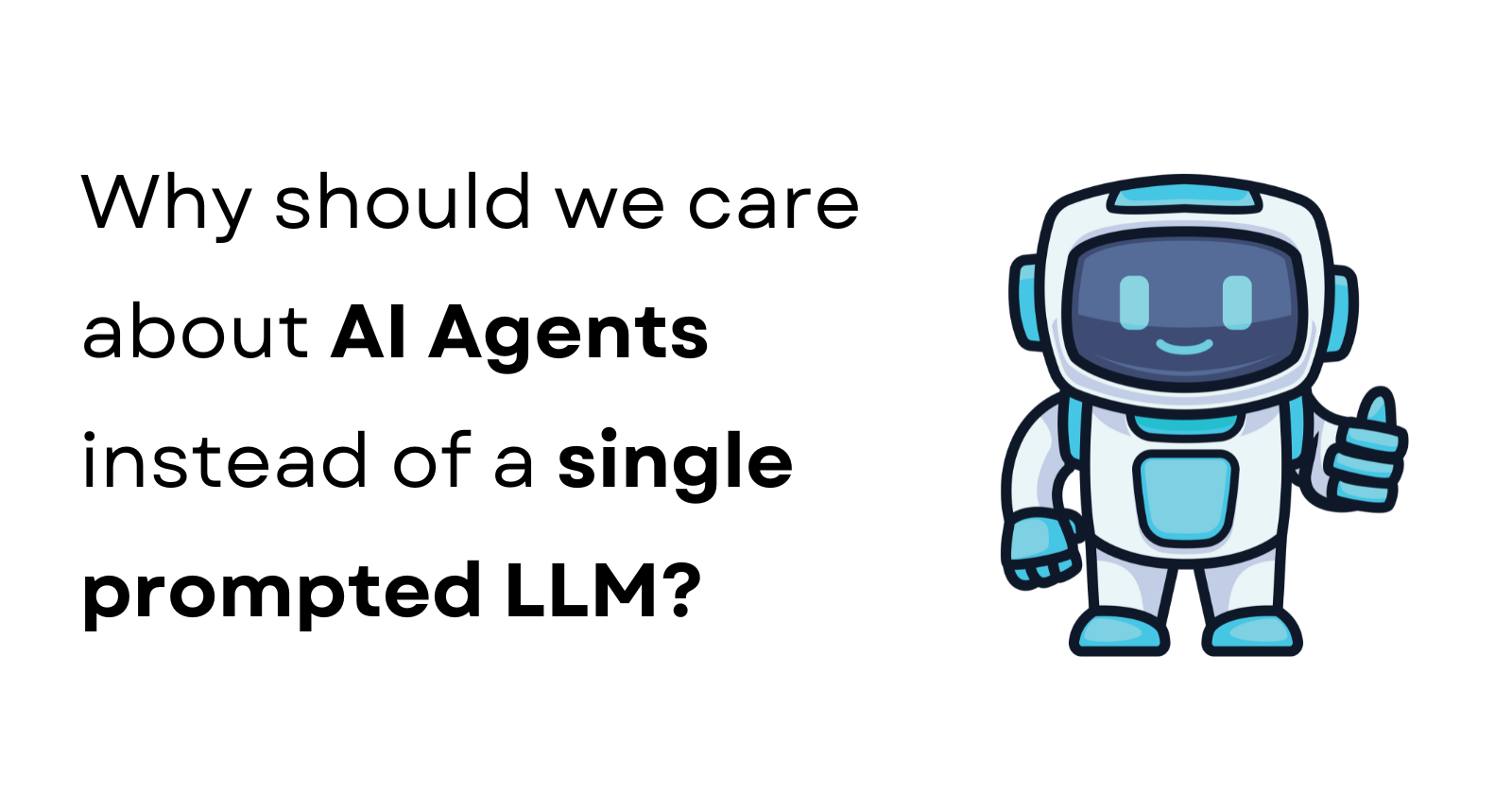
Artificial intelligence (AI) is now changing industries at an incredible rate. Two technologies contributing to this shift are single-prompted LLMs and AI agents. Such true LLMs like GPT-4 have become famous due to their ability to generate human-like text. Although LLMs are proficient in dealing with single queries, they are weak in dealing with multiple-stage problems without interacting judiciously with the users.
AI agents, unlike simple AI systems, are more sophisticated. Most importantly, they can work independently and even learn during interactions with an individual or different objects. Unlike LLMs, which require a prompt for each action to be performed next, AI agents can perform one operation after another; more agents can be coordinated to work together and make efforts to make the operations more effective in the coming time. This makes them highly suitable for industries such as finance, healthcare, and decentralised computing industries.
In this blog, I’ll dissect why AI agents are better than LLMs trained with a single prompt and how they can be leveraged with decentralised and scalable AI platforms like Spheron Network to open up the doors to advanced, yet efficient AI.
Understanding Single-Prompted LLMs.
Single-prompted LLMs, like GPT-4, are AI models that take a text prompt and emit human-like text output. Many of these models have been useful in generating the content, responding to enquiries, and providing solutions to particular tasks depending on the data fed into them. For instance, the GPT-4 can write an essay, prepare an email, or even write lines of code depending on the given input.
However, there are some disadvantages that you need to consider when opting for LLMs to enhance your qualifications. Plain and simple, they act stupid after they have accomplished a task and claim that they have forgotten what they just did. This means they require a new prompt for each new task, which is less effective if the process needs to be continuously ongoing or involves detailed analysis of results. Consequently, LLMs do perform well in generating content and addressing single queries, although they do not offer the level of self-governance that organisations with continual, large-scale AI operations require.
What Makes AI Agents Different?
In this sense, AI agents take a fundamentally different approach. However, depending on obtained LLMs, AI agents will be able to complete tasks on their own rather than constantly interacting with users. You would wish to have a helper who responds to your emails, sets appointments, reminds clients of agreements, and manages the day’s activities on your behalf. This is where AI agents come into play. When implemented in the real world, this is the power of AI agents.
The main reason for the distinction between LLMs and AI agents is that they are fully autonomous. Once a task is delegated to an AI agent, it can subdivide the work into several operational procedures that can be performed and then reinvented if required. For example, if given a travel task, an AI is able to find a location, book flights and a hotel, select tours, and more, all autonomously.
Also, AI agents have the memory and the contextual knowledge to use their previous experiences to train on and complete subsequent tasks. They also can cooperate with other agents and share the workload, each doing a part of the more comprehensive task. That’s why AI agents are much stronger than LLMs, including their autonomy, memory, and the ability to work in a team, especially in cases that presuppose several steps or constant operation.
Key Advantages of AI Agents
Autonomy in Action: AutoGPT, BabyAGI, and Sophia the Robot
AutoGPT and BabyAGI are two familiar AI agents that demonstrate the capabilities of automatic systems. For example, AutoGPT can be programmed to come up with a business strategy, gather information on trends, and even launch marketing strategies without having to input distinct instructions for each process. Also like AGI Baby, it can handle and accomplish sequences of tasks and learns in the process from outcome feedback.
Perhaps the most complex of all existing AI agents is Sophia the Robot, built by Hanson Robotics, showing how these AI agents are used. Sophia can actually talk and interact with others, calculate feelings, and even perform certain social roles, such as interviews or providing some advice according to the prior information, on her own. While LLMs require a set of new circumstances for each action, AIs such as AutoGPT & Sophia are already able to decompose tasks, adapt to feedback on the model, and perform multi-step tasks at different levels without asking for help.
Meaningful Contextual Sense and Synergy
AI agents are capable of remembering what they did before, which makes them useful in a given task. This capability makes AI agents suitable for those activities that are continuous or involve an interaction with the environment. For instance, in a large project, several AI agents may cooperate to solve parts of the project at different levels and in a distributed fashion.
The Position of Decentralisation
For the AI agents to implement a greater strategy towards the actualisation of efficiencies, they would need an existing technological environment to operate. This is where decentralised Computing and Scalable Infrastructure come into the picture.
Decentralised Computing
In decentralised computing, the work is split up into various nodes and not centrally with a server. Such arrangements make the system more reliable and, to a certain extent, more efficient across the various partitions of the whole system. This is in the event that one node develops a problem, and then other nodes take over just in case there are issues with the tasks.
Decentralised computing is actually quite beneficial for AI agents because the structures commonly manage vast quantities of data. For instance, decentralised systems are safer than centralised systems since there is always focus. This still makes them fit for use by AI agents that process vast amounts of data and complex processes, for example, in dApps or financial markets.
Scalable Infrastructure
The term flexible means that it is possible for a firm to expand or contract depending on the performance of the company. For example, if artificial intelligence has been applied in data processing at a large scale, then scalability is understood as the ability of the system to perform the same at a larger scale. Whereas during low activity the system might be able to reduce the use of the resources and thereby reduce the cost.
AI agents need decentralised and scalable solutions from Spheron Network, which is why this platform is suitable for its construction. That is why, thanks to Spheron Network, AI agents can safely take up large-scale workloads because, in this way, businesses can grow without worrying about infrastructure.
AI Agents and Spheron Networks: A Case Study
To illustrate the potential and applicability of using AI agents and decentralised system layout, let's explore the Spheron Network case.
Case Study: Optimised Data Management in Decentralised Finance (DeFi)
If a DeFi platform is dealing with up to a thousand transactions on a daily basis, it therefore requires a solution unit to track its AI workloads. The platform sets up its AI agents to facilitate the online monitoring of blocks and the execution of contracts. However, the platform has high operation costs, and the idea of expanding the community and targeting many people proved difficult.
Through connection with Spheron Network, the platform provides an opportunity to distribute AI agents across the decentralised nodes, which reduces the risk of a system failure and a security threat. The capability of the architecture to support the access of high traffic flows without additional investment in resources led to the optimisation of the operation costs by a third, demonstrating cost-efficient compute capabilities.
This tries to explain how the AI agents, when backed by a decentralised and global structure, can enhance the functionality and extend the affordability, security, and scalability of business solutions.
Conclusion
All in all, AI agents have better features over single-prompted LLMs because of their abilities to be self-controlled, have memory, dislike being alone, and can team with other agents. But for single, specific tasks, LLMs like GPT-4 are ideal, unlike AI agents we’ve seen that can carry out numerous, interdependent tasks as one, hence suitable for big operations in financial services, healthcare, and decentralised computing industries.
When integrated with decentralised and scalable structures like the Spheron Network, AI agents work better and cost less, or, in other words, they offer businesses what they need - efficient tools for the company’s growth. Different perspectives are going to carry AI forward; certainly, the cooperation between AI agents and decentralised— computing will present us with new ways of being intelligent in the digital age.
Subscribe to my newsletter
Read articles from Sheefa Jalali directly inside your inbox. Subscribe to the newsletter, and don't miss out.
Written by

Sheefa Jalali
Sheefa Jalali
Social Media Executive at Bandit Network | Web3 Writer