Hyperautomation: Driving Large-Scale Adoption in the Digital Era
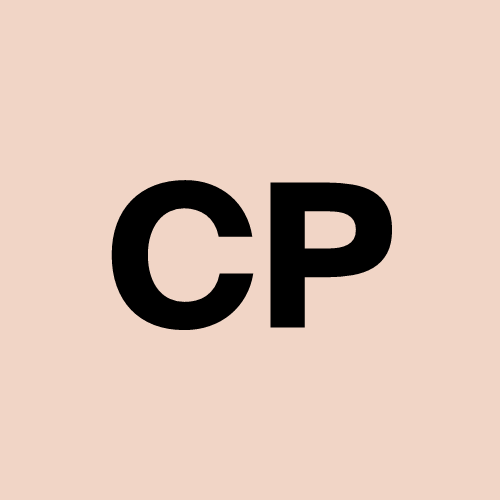
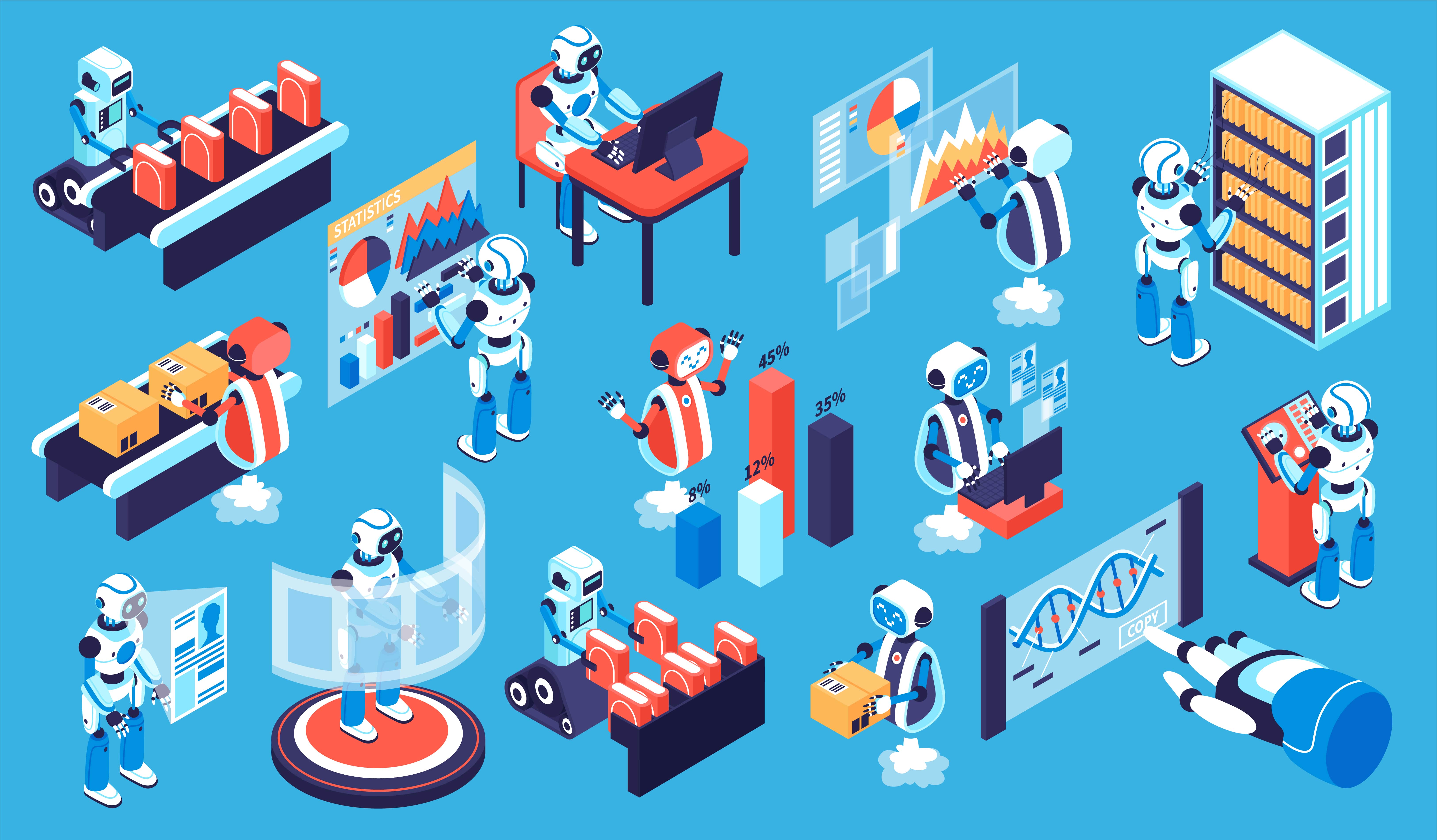
About the author
This article is authored by Chaitanya Pathak. He is a seasoned technology leader with extensive expertise in product management and enterprise software, boasting over a decade of experience at the nexus of product innovation and technology. As the Chief Product and Technology Officer at LEAPS by Analyttica, he spearheads the development and execution of advanced technological solutions that drive innovation in dynamic pricing and machine learning. Chaitanya's strategic vision and deep technical acumen consistently deliver impactful solutions, enhancing the company's product offerings and positioning it at the forefront of industry advancements.
Introduction
Organizations are constantly seeking ways to streamline operations, enhance efficiency, and drive innovation. Hyperautomation presents a sophisticated approach that combines multiple technologies to automate complex business activities at an unprecedented scale. This article delves into the concept of hyperautomation, its impact on various industries, and its practical applications in driving large-scale adoption of data literacy and advanced analytics.
Understanding Hyperautomation
From RPA to Intelligent Automation
To fully grasp the concept of hyperautomation, it’s essential to understand its evolution:
Robotic Process Automation (RPA): The foundation of modern automation, RPA focuses on automating repetitive, rule-based tasks using software bots. Example: Automating data entry from invoices into accounting systems.
Intelligent Automation: Building upon RPA, this approach incorporates machine learning and artificial intelligence to handle more complex tasks. Example: Using natural language processing to categorize and route customer support tickets.
Hyperautomation: The next frontier, combining multiple advanced technologies to automate end-to-end processes and workflows across entire organizations.
Defining Hyperautomation
Hyperautomation is the synchronized and integrated use of multiple technologies, including:
Artificial Intelligence (AI)
Machine Learning (ML)
Robotic Process Automation (RPA)
Business Process Management (BPM)
Natural Language Processing (NLP)
Optical Character Recognition (OCR)
Advanced Analytics
Example: A hyperautomation solution in healthcare might combine RPA for data entry, AI for diagnosis assistance, ML for predictive analytics, and NLP for processing patient records – all working together seamlessly to improve patient care and operational efficiency.
Key Components of Hyperautomation
Process Discovery: AI-powered tools that analyze existing workflows to identify automation opportunities.
Advanced Analytics: Utilizing big data and ML algorithms to derive insights and drive decision-making.
Intelligent Document Processing: Combining OCR and NLP to extract and process information from unstructured documents.
Low-Code/No-Code Platforms: Enabling citizen developers to create and deploy automation solutions.
AI-Powered Decision Making: Leveraging advanced algorithms to automate complex decision processes.
The Impact of Hyperautomation
Empowering Citizen Users
Hyperautomation brings sophisticated technologies within reach of non-technical users, fostering bottom-up innovation. For example, drag-and-drop AI-powered analytics tools allow marketing professionals to perform complex customer segmentation without extensive coding knowledge.
Intelligent Decision Making
By combining NLP, advanced analytics, and machine learning, hyperautomation enables autonomous decision-making systems. For instance, in financial services, these systems can analyze market trends, company reports, and news articles to make real-time investment recommendations.
Adaptive Workflows
Unlike traditional automation, hyperautomation creates dynamic workflows that can adapt to changing conditions. In manufacturing, for example, an AI-powered system can adjust production lines in real-time based on supply chain disruptions, demand fluctuations, and equipment performance.
Enhanced Knowledge Management
Hyperautomation excels at capturing and codifying tacit knowledge within organizations. For example, an AI-powered system can analyze patterns in how experienced employees solve complex problems, creating a knowledge base that can be used to train new staff or automate future problem-solving.
Scaling Adoption: A Case Study of LEAPS
LEAPS, a SaaS product focused on data literacy, is leveraging hyperautomation to enhance its offerings and drive adoption at scale. Here’s how:
Challenges in Data Literacy
Many organizations struggle with:
Understanding data in context
Performing "what-if" scenario analyses
Moving from explanatory to experimental data use
LEAPS’ Hyperautomation Solution
To address these challenges, LEAPS has developed an integrated hyperautomation architecture that includes:
Graph RAG (Retrieval-Augmented Generation): For understanding user context based on historical data and domain rules.
Natural Language Interfaces: Allowing users to interact with data using conversational prompts.
Action-based Recommendation Engines: Suggesting next steps and analyses based on user behavior and data patterns.
Automated ML Pipeline Generation: Creating and executing machine learning models based on user requirements.
Automated Dashboard Creation: Generating visualizations and reports tailored to user needs.
This hyperautomation approach allows LEAPS to:
Automate end-to-end workflows for scenario identification
Generate synthetic data for testing and training
Create and deploy ML models with minimal user intervention
Produce automated, context-aware dashboards and reports
The Future of Hyperautomation
As organizations continue to embrace digital transformation, hyperautomation will play an increasingly crucial role. Key trends to watch include:
Integration of Generative AI: Incorporating large language models to enhance natural language interactions and content generation within automated workflows.
Edge Computing in Hyperautomation: Bringing automation closer to data sources for real-time processing and reduced latency.
Ethical AI and Governance: Developing frameworks to ensure responsible and transparent use of AI in automated decision-making processes.
Cross-functional Process Optimization: Breaking down silos to create seamless, organization-wide automated workflows.
Conclusion
Hyperautomation represents a paradigm shift in how organizations approach digital transformation. By combining RPA, AI, ML, and other advanced technologies, it offers unprecedented opportunities for efficiency, innovation, and growth. As demonstrated by LEAPS’ implementation in the field of data literacy, hyperautomation has the power to democratize complex technologies, enabling citizens within organizations to participate in high-value activities that drive core competencies.
As we move forward, the focus will be on creating human-centric hyperautomation solutions that augment rather than replace human capabilities, fostering a collaborative environment where technology and human expertise work hand in hand to tackle the challenges of the digital age.
Subscribe to my newsletter
Read articles from Chaitanya Pathak directly inside your inbox. Subscribe to the newsletter, and don't miss out.
Written by
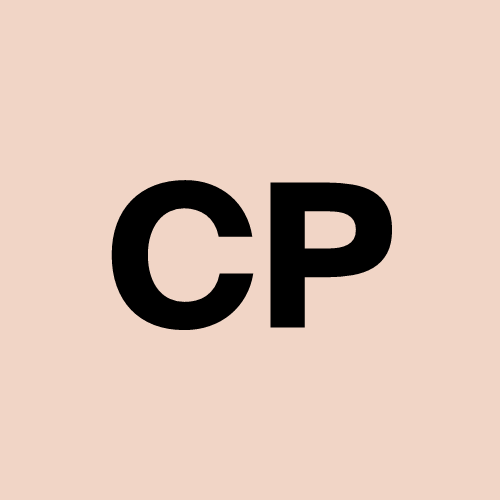