Data Science In The Development Of Public Health Surveillance

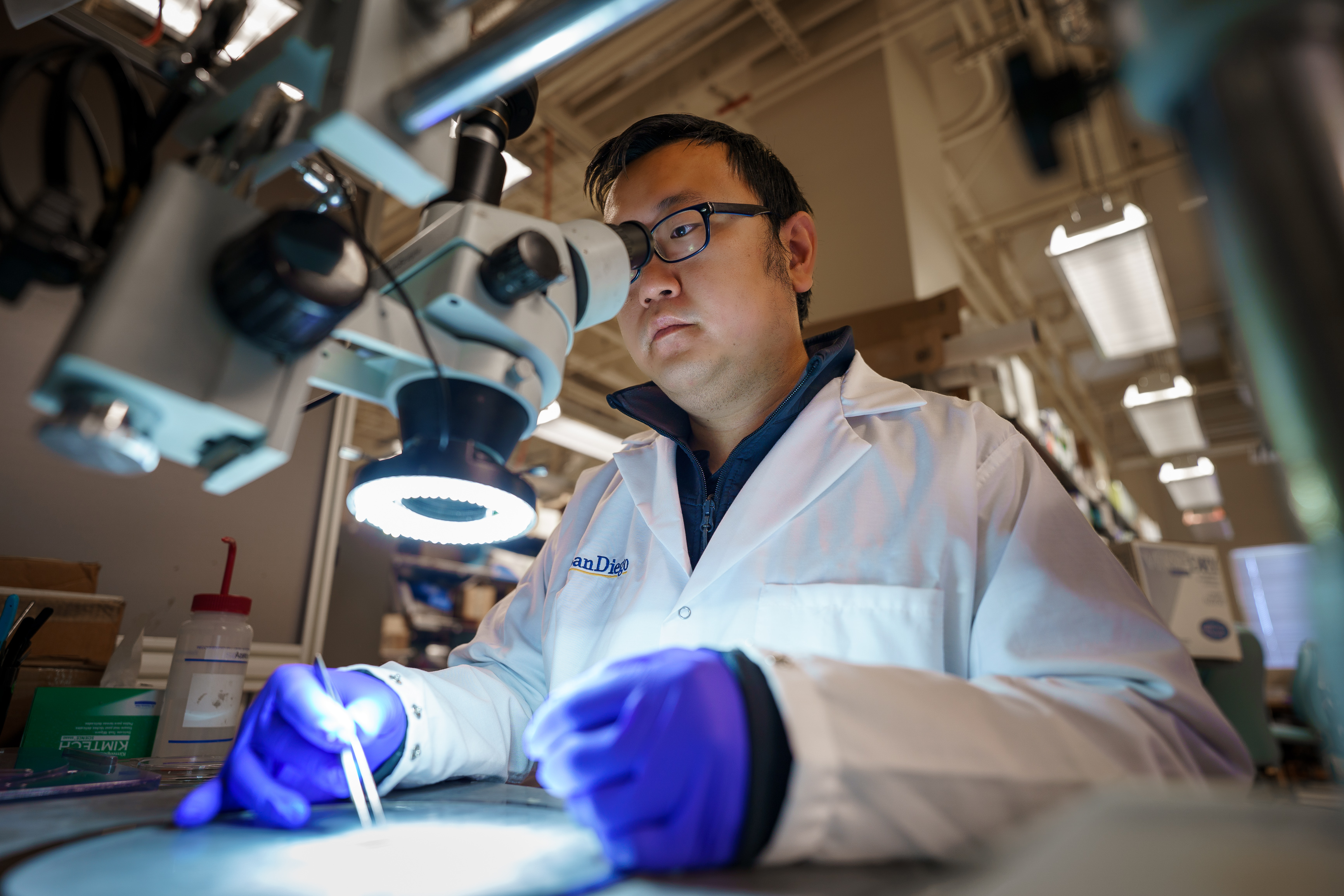
In this environment, big data science also becomes the driver of change in the constantly developing field of public health. With increasing rates of global disease and morbidity from simple infectious diseases to chronic disease and non-communicable diseases, data science enables coherent public health intelligence and surveillance. This blog explores one of the multiple aspects of data science in improving public health surveillance, its methods and uses, and future developments.
This work aims at providing the reader with a clear understanding of public health surveillance.
Public health surveillance is health-related information. It is used to provide trends within the healthcare system, including policies and approval of interventions in the healthcare delivery systems. Indeed, traditional surveillance systems can include practices such as manually entering data into a computer, and time intervals for reporting, which can slow down intervention during public health emergencies. Specifically, integrating data science advances these processes by proposing distinctive and effective approaches to their execution.
1. Data Integration and Management is the first level of the five-tiered DMG process.
One of the most significant capabilities of data science in support of public health surveillance is its help when working with ‘big’ data. From records of clinical consultations, laboratory findings, social media, and even some environmental monitoring devices, public health data can be obtained. These different sources use data management frameworks such as data warehousing and data lakes to amalgamate into large data sets.
To achieve this, data scientists apply programming languages such as Python and R to data analytics besides database technologies like Structured Query Language (SQL) and No-SQL for extracting, formatting, and storing health data. This is so that it is not only credible but also easily retrievable. This reliable data structure is conducive to real-time analysis; thus, responding to new diseases with proximity.
2. Risk Assessment for Early Interference
Data analytics involves using past data to come up with an assortment of potential health risks. This capability is helpful, especially in public health where assessing the origins of certain health events can significantly influence the allocation of resources and interventions.
For instance, epidemiologic models, machine learning, or artificial intelligence models can estimate the risk factors that determine the spread of diseases including vaccination rate, mobility, and population density. In the COVID-19 pandemic, predictive modeling was used in estimating the infection rates and the effectiveness of control measures including the cessation of human interaction.
The use of predictive models is one-way public health organizations can move from performing merely reactive roles to proactive roles, which is very important, especially within public health centers.
3. Real Time Surveillance and Monitoring Technologies
Advanced technologies help pioneers of data science monitor occurrences in real-time. Mobile health applications, wearable devices, and sensors from IoT promote health asymptomatic check-ups. These technologies enable the fast capture and analysis of data together with cloud computing.
NLP can also be used in health informatics to discover health-relevant information from social media and forums and to identify real-time health problems in a community. For instance, examining the Twitter content during the flu season would allow identifying a need to interact with the public much more on this topic.
In addition to improving the agility of the public health response, this capacity to track health trends in real time is also shown to increase response speeds.
4. Geospatial Analysis for Finding Need-Specific Coverage
Spatial statistics is a vital data analysis technique for understanding geographical locations of health concerns. This way, using Geographic Information Systems (GIS), maps can represent particular types of data, such as disease abundance or possible risks.
For instance, the geocoding of diseases against socioeconomic factors may identify relationships that can after that be addressed. This means it is easier to prioritize where resources will be used, for example, in high-risk areas for vaccination or using mobile clinics in areas that rarely benefit from clinics.
Spatial analysis goes a long way in explaining health inequalities to inform better public health interventions that are targeted to specific groups of people.
5. TyingDown Effective Communication and visualization
Since the implementation of the programs mostly involves communities, the use of communication is critical to their success. Data visualization methods enable intricate information in the field of health to be displayed in a way that can be understood and acted upon. Web dashboards and other interactive tools are useful when providing officials and the public with an understanding of essential points.
For instance, using a graphic display of the development of an epidemic to explain why some measures are required—including wearing face masks during the Covid-19 pandemic—, can increase the levels of public compliance. So, data scientists can use tools like Tableau or Power BI to create reports that provide stakeholders with fresh information.
Conclusion
Data science is revolutionizing public health surveillance since today’s health issues require analytical approaches. By combining multiple data types, using predictive analysis, allowing for constant surveillance, using geographic data, and improving reporting, data science strengthens the capacity of health authorities to provide strategic choices.
In the future, engagement with data science superiority will remain paramount in delivering robust public health frameworks. It is now possible to bring data scientists, public health practitioners, and policymakers to an optimal level that ensures the use of data for Community health safety, and wellness and to mitigate any forthcoming threat to public health. In today’s era where a decision cannot be made without the support of data, it is useful, no, crucial to have a Data Science and AI Course in public health surveillance.
Subscribe to my newsletter
Read articles from nibedita nibedita directly inside your inbox. Subscribe to the newsletter, and don't miss out.
Written by
