Crafting a Clear Value Proposition for Your Machine Learning Solution: Geoffrey Moore's Template

Table of contents
- What is Geoffrey Moore's Value Positioning Statement?
- Applying the Value Positioning Statement to a Machine Learning Project
- For telecom companies who want to reduce customer churn,
- The churn prediction model is a machine learning-based system
- That predicts which customers are most likely to churn, allowing proactive retention strategies.
- Unlike traditional rule-based systems,
- Our product uses advanced predictive analytics to accurately identify at-risk customers, leading to improved retention rates.
- Refining Your Own Value Proposition for Machine Learning Projects
- Key Takeaways
- Conclusion
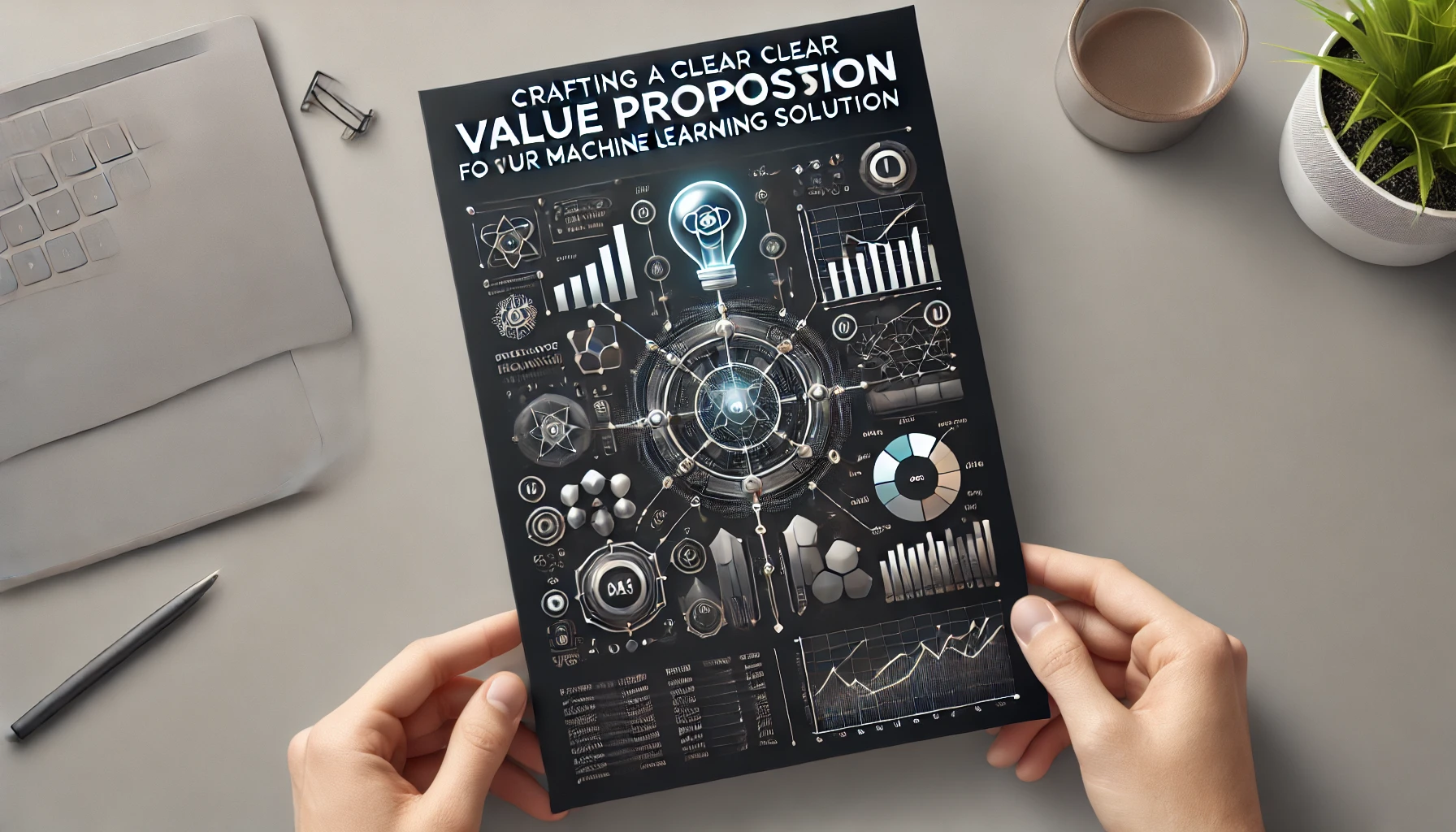
In the world of machine learning, one of the biggest challenges isn’t just building the model—it’s communicating the value your solution brings to your business, users, or clients. That's where Geoffrey Moore's Value Positioning Statement comes in. This simple yet powerful template helps you clarify who your solution is for, what problem it solves, and why it's better than the competition.
In this blog post, we'll walk through Geoffrey Moore's Value Positioning Statement template and apply it to a machine learning project, helping you articulate the true value of your solution.
What is Geoffrey Moore's Value Positioning Statement?
Geoffrey Moore, author of "Crossing the Chasm," created this template to help businesses clearly define the value of their product or service in a competitive market. The template goes like this:
For (target customer)
Who (statement of the need or opportunity)
The (product name)
Is a (product category)
That (key benefit or compelling reason to use the product)
Unlike (primary competitive alternative)
Our product (primary differentiation)
This simple format forces you to define exactly who your target user is, what problem you're solving, and how your solution stands out from the competition.
Why is this important for Machine Learning?
In machine learning projects, especially those that are data-heavy and technically complex, it’s easy to get lost in the algorithms and technicalities. The Value Positioning Statement helps you zoom out and focus on the bigger picture: What value is your solution delivering?
Whether you're building a fraud detection model, a recommendation system, or a customer churn predictor, clearly stating your value proposition can help you communicate with stakeholders, clients, and business teams in a way they understand.
Applying the Value Positioning Statement to a Machine Learning Project
Let’s walk through an example. Imagine you’re building a machine learning model that predicts customer churn for a telecom company. This model helps the company retain customers by identifying which ones are likely to leave, allowing them to take preemptive actions.
Here’s how we would apply Geoffrey Moore’s template:
For telecom companies who want to reduce customer churn,
Telecom companies are constantly dealing with customer attrition. Keeping customers longer directly impacts revenue. A machine learning model that predicts churn can significantly help by identifying at-risk customers, allowing proactive action before they leave.
The churn prediction model is a machine learning-based system
This solution is a machine learning system that uses historical customer data (such as usage patterns, billing information, customer complaints, etc.) to predict future behavior. It helps decision-makers pinpoint potential churn risks.
That predicts which customers are most likely to churn, allowing proactive retention strategies.
The key benefit of the churn prediction model is that it provides actionable insights. By predicting the likelihood of churn, the company can reach out to at-risk customers with personalized offers or retention strategies, potentially saving millions in lost revenue.
Unlike traditional rule-based systems,
Traditional customer churn prevention methods often involve manually setting rules, like flagging customers based on certain behaviors. These methods tend to be reactive, and not all customers who exhibit churn-like behavior actually leave.
Our product uses advanced predictive analytics to accurately identify at-risk customers, leading to improved retention rates.
The machine learning model uses advanced techniques such as classification algorithms, feature engineering, and real-time data inputs to predict churn with much greater accuracy. This allows the business to retain customers they might otherwise have lost.
Refining Your Own Value Proposition for Machine Learning Projects
Now that we’ve broken down Geoffrey Moore’s template, you can apply it to your own machine learning projects. Let’s say you’re working on a recommendation system for an e-commerce platform. You might frame it like this:
For e-commerce platforms who want to increase customer engagement and sales,
The recommendation engine is a machine learning-powered recommendation system
That provides personalized product recommendations to users, leading to higher conversion rates.
Unlike generic recommendation systems,
Our product tailors recommendations based on real-time user behavior, preferences, and past purchases, resulting in a more personalized shopping experience and increased sales.
Key Takeaways
Creating a value proposition for machine learning solutions using Geoffrey Moore’s template forces you to think critically about your product's impact. It helps you define your target audience, communicate the core benefit, and highlight what makes your solution unique compared to others.
Remember, the goal isn’t just to build a great model—it’s to build a solution that delivers real value to your users or business.
By filling out each section of this template, you’ll be better prepared to explain the value of your project to stakeholders, making it easier to gain support, funding, or buy-in for your machine learning initiatives.
Conclusion
Machine learning is all about solving problems using data. But without a clear value proposition, even the most sophisticated model can fail to resonate with decision-makers. Geoffrey Moore’s Value Positioning Statement simplifies the process of communicating the why behind your project, helping you cut through the technical jargon and deliver a compelling message.
Next Steps
Want to see more examples of how to apply this template to different machine learning use cases? Stay tuned for upcoming blog posts where we dive deeper into the specifics of various ML applications.
Try applying this template to your next machine learning project, and watch how much clearer your communication becomes!
Subscribe to my newsletter
Read articles from Riya Bose directly inside your inbox. Subscribe to the newsletter, and don't miss out.
Written by
