Understanding the Role of Psychology in AI Interactions

Table of contents
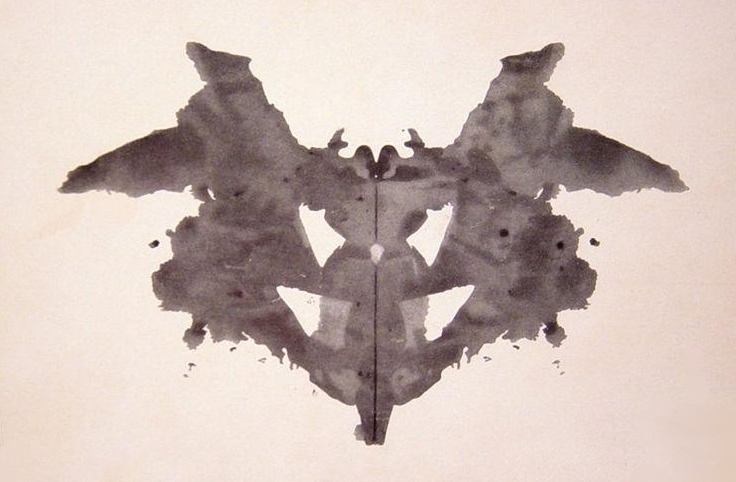
In the rapidly evolving landscape of artificial intelligence, we often focus on the capabilities and limitations of AI systems themselves. However, an equally crucial aspect that deserves our attention is how humans perceive and interpret AI-generated outputs from the perspective of psychology. This blog post delves into the complex interplay between human perception and AI, exploring how our biases, cultural backgrounds, and individual worldviews shape our understanding of AI-generated content.
The Concept of Umwelt: Shaping Our Perception
At the heart of this discussion lies the concept of umwelt - a term borrowed from biology and anthropology that describes the subjective world each individual perceives. For humans interacting with AI, our umwelt encompasses:
Sensory input
Cultural context
Language proficiency
Social conditioning
These factors create a personalized lens through which we interpret AI outputs, often leading to diverse interpretations of the same content.
Example: Language and Perception of AI
Consider an AI-generated text containing idiomatic expressions. A multilingual individual might grasp nuances lost on someone fluent in only one language. This disparity in interpretation highlights how our linguistic umwelt influences our perception of AI capabilities.
Cognitive Biases and Limits: The Human Factor in AI Interaction
Human cognitive biases play a significant role in how we perceive AI-generated outputs. These biases can lead to misinterpretations and misjudgments about AI's capabilities, trustworthiness, and reliability.
Key Biases Affecting AI Interpretation:
Anthropomorphic Bias: projecting human traits into things that don’t possess them.
Confirmation Bias: We tend to interpret AI outputs in ways that confirm our pre-existing beliefs about AI.
Anchoring Bias: Initial information from AI can disproportionately influence our subsequent judgments.
Cognitive Load and Working Memory Limitations: Complex AI outputs may overwhelm our cognitive capacity, leading to incomplete processing and flawed conclusions.
There is no red in the picture above. Your mind is filling the gaps. AI anthropomorphism works the same way.
The Role of Education and Expertise
An individual's knowledge and domain expertise significantly impact how they interpret AI outputs. Experts in a field are likely to scrutinize AI-generated content more critically, while laypeople might accept it at face value.
Example: Domain Expertise in Math and Statistics
When an AI model generates statistical information:
A statistician might question the underlying assumptions and identify potential biases.
A layperson might accept the output without critical evaluation.
This difference in approach underscores how expertise shapes our interaction with AI systems.
Cultural Background and Worldview
Cultural differences can lead to vastly different interpretations of the same AI-generated content. What one culture sees as neutral, another might perceive as offensive or inaccurate.
Example: AI-Generated Meal Recommendations
An AI suggesting recipes might omit an ingredient considered essential in one culture but optional in another. This cultural discrepancy can lead to divergent perceptions of the AI's competence, despite the output being identical.
Media Exposure and Access to Knowledge
Our exposure to media and information shapes our worldview, which in turn influences how we interpret AI outputs. This is particularly evident in AI-generated content related to historical events or political issues.
Example: AI Summarizing Historical Events
An AI summary of a historical event might be perceived differently by individuals from countries with contrasting historical narratives. The same factual output can be seen as accurate by some and biased by others, based on their education and cultural background.
Self-Awareness and Metacognition
The ability to reflect on one's thought processes (metacognition) plays a crucial role in how we interact with AI. Individuals with high metacognitive skills are better equipped to recognize their biases and evaluate AI outputs more objectively.
Example: Reflecting on AI Decision-Making
A person with strong metacognitive abilities might recognize when their interpretation of an AI output is influenced by personal biases or limited understanding, leading to more informed interactions with AI systems.
Implications for Human-AI Interaction
Understanding these perceptual differences is crucial for improving human-AI collaboration. Key considerations for AI developers include:
Personalized AI Outputs: Adapting outputs based on user background and expertise.
User Education: Providing training on AI limitations and operational mechanisms.
Transparent Decision-Making: Explaining how AI generates outputs to enhance user understanding.
Conclusion
The interpretation of AI outputs is far from uniform, influenced by a complex web of cognitive biases, cultural backgrounds, and individual knowledge. By recognizing and accounting for these human factors, we can design AI systems that interact more effectively with diverse users, fostering trust and improving the overall quality of human-AI collaboration.
As AI continues to integrate into our decision-making processes, understanding these perceptual nuances becomes crucial. It's not just about advancing AI technology, but also about enhancing our ability to interpret and utilize AI outputs effectively. By bridging the gap between AI capabilities and human perception, we can create more robust, equitable, and user-friendly AI systems that truly serve the diverse needs of their users.
Subscribe to my newsletter
Read articles from Gerard Sans directly inside your inbox. Subscribe to the newsletter, and don't miss out.
Written by

Gerard Sans
Gerard Sans
I help developers succeed in Artificial Intelligence and Web3; Former AWS Amplify Developer Advocate. I am very excited about the future of the Web and JavaScript. Always happy Computer Science Engineer and humble Google Developer Expert. I love sharing my knowledge by speaking, training and writing about cool technologies. I love running communities and meetups such as Web3 London, GraphQL London, GraphQL San Francisco, mentoring students and giving back to the community.