Advanced Machine Learning with Scikit-learn: A Deep Dive
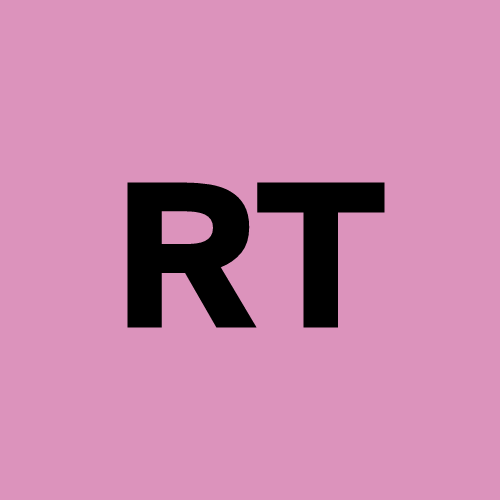
Table of contents
- What Makes Scikit-learn So Powerful?
- Why Use Scikit-learn in Advanced Machine Learning Projects?
- Advanced Concepts in Scikit-learn
- 1. Cross-Validation: Ensuring Generalization
- 2. Hyperparameter Tuning: Optimizing Your Models
- 3. Pipelines: Simplifying Workflows
- 4. Ensemble Learning: Boosting Performance with Multiple Models
- Model Evaluation and Metrics
- Conclusion
- Key Takeaways:
Machine learning (ML) is transforming industries by allowing companies to uncover patterns, make predictions, and improve decision-making. While there are numerous ML libraries, Scikit-learn stands out due to its simplicity and power. Whether you’re a beginner or an experienced data scientist, Scikit-learn has something to offer.
In this post, we’ll explore the more advanced aspects of Scikit-learn, including model selection, hyperparameter tuning, pipelines, and ensemble methods. By the end, you’ll be well-equipped to leverage Scikit-learn for building efficient and complex ML models.
What Makes Scikit-learn So Powerful?
Scikit-learn is often the go-to library for machine learning because it strikes a perfect balance between simplicity and depth. It includes:
Wide Range of Algorithms: From classic models like linear regression to advanced ensemble methods like Random Forest and Gradient Boosting.
Preprocessing: Tools for feature scaling, encoding categorical variables, and dimensionality reduction.
Model Evaluation: Cross-validation, metrics, and model selection techniques to help assess performance.
Comprehensive Documentation: An extensive and well-maintained user guide makes learning smooth.
Why Use Scikit-learn in Advanced Machine Learning Projects?
Consistency: The API remains the same whether you are working with linear regression, support vector machines (SVM), or ensemble methods.
Integration: It integrates effortlessly with libraries like Pandas for data manipulation and Matplotlib/Seaborn for visualization.
Custom Pipelines: Easily create workflows that streamline the entire machine learning process, from preprocessing to model evaluation.
Advanced Concepts in Scikit-learn
1. Cross-Validation: Ensuring Generalization
One of the fundamental concepts in building robust machine learning models is cross-validation. It helps ensure that the model generalizes well to unseen data. The most common form is k-fold cross-validation, where the data is split into k
subsets. The model is trained on k-1
subsets and validated on the remaining one. This process is repeated k
times, and the performance metrics are averaged across the folds.
Example:
from sklearn.model_selection import cross_val_score
from sklearn.ensemble import RandomForestClassifier
from sklearn.datasets import load_iris
# Load dataset
data = load_iris()
X, y = data.data, data.target
# Create model
model = RandomForestClassifier()
# Perform 5-fold cross-validation
cv_scores = cross_val_score(model, X, y, cv=5)
print(f"Cross-validation scores: {cv_scores}")
print(f"Mean accuracy: {cv_scores.mean():.2f}")
Cross-validation is especially important when working with small datasets, ensuring that the model does not overfit and performs well on unseen data.
2. Hyperparameter Tuning: Optimizing Your Models
Every machine learning algorithm has parameters that can be adjusted to fine-tune model performance. These are called hyperparameters. For example, in a Random Forest, you can adjust the number of trees, the maximum depth of each tree, and the minimum samples required for splitting a node.
Scikit-learn offers two primary methods for hyperparameter tuning:
Grid Search (
GridSearchCV
): Exhaustively searches for the best combination of hyperparameters from a grid of predefined options.Random Search (
RandomizedSearchCV
): Samples a fixed number of random combinations from a range of hyperparameters.
Example: Hyperparameter tuning with GridSearchCV for a RandomForestClassifier.
from sklearn.model_selection import GridSearchCV
from sklearn.ensemble import RandomForestClassifier
# Define model
model = RandomForestClassifier()
# Define hyperparameter grid
param_grid = {
'n_estimators': [50, 100, 200],
'max_depth': [None, 10, 20, 30],
'min_samples_split': [2, 5, 10]
}
# Grid Search
grid_search = GridSearchCV(estimator=model, param_grid=param_grid, cv=5, n_jobs=-1, verbose=2)
grid_search.fit(X, y)
print(f"Best Hyperparameters: {grid_search.best_params_}")
Hyperparameter tuning is crucial to improving your model’s accuracy, precision, and robustness, especially when working with high-dimensional or complex data.
3. Pipelines: Simplifying Workflows
As machine learning projects become more complex, managing the different steps of the workflow (data preprocessing, model training, and evaluation) can become cumbersome. Scikit-learn’s Pipelines allow you to string together multiple steps into a single process.
A typical workflow includes:
Preprocessing: Scaling or encoding the data.
Feature Selection: Choosing the most relevant features.
Model Training: Fitting a model.
Evaluation: Cross-validation and performance assessment.
Example: Creating a pipeline with a scaler and a classifier.
from sklearn.pipeline import Pipeline
from sklearn.preprocessing import StandardScaler
from sklearn.ensemble import RandomForestClassifier
from sklearn.model_selection import train_test_split
# Define pipeline
pipeline = Pipeline([
('scaler', StandardScaler()), # Preprocessing step
('classifier', RandomForestClassifier()) # Model training step
])
# Split the data
X_train, X_test, y_train, y_test = train_test_split(X, y, test_size=0.2)
# Train the model within the pipeline
pipeline.fit(X_train, y_train)
# Evaluate the model
score = pipeline.score(X_test, y_test)
print(f"Accuracy: {score * 100:.2f}%")
With pipelines, your code becomes cleaner, easier to debug, and more robust to changes in the workflow.
4. Ensemble Learning: Boosting Performance with Multiple Models
Ensemble learning combines the predictions of multiple models to improve accuracy and robustness. Scikit-learn supports two types of ensemble methods:
Bagging: Combines the predictions of multiple independent models (e.g., Random Forest).
Boosting: Sequentially trains models, where each model focuses on the mistakes of the previous one (e.g., Gradient Boosting, AdaBoost).
Random Forest is an example of a bagging method that builds multiple decision trees and averages their predictions.
Gradient Boosting, on the other hand, is a boosting method that focuses on improving the errors made by the previous models.
Example: Gradient Boosting Classifier
from sklearn.ensemble import GradientBoostingClassifier
# Gradient Boosting model
model = GradientBoostingClassifier(n_estimators=100, learning_rate=0.1, max_depth=3)
# Train and evaluate
model.fit(X_train, y_train)
score = model.score(X_test, y_test)
print(f"Gradient Boosting Accuracy: {score * 100:.2f}%")
Ensemble methods can significantly improve model performance, especially when dealing with complex datasets.
Model Evaluation and Metrics
While accuracy is a common metric, it is often insufficient for imbalanced datasets. Scikit-learn provides a range of evaluation metrics to assess your models more holistically:
Precision, Recall, F1-Score: Useful for classification tasks, especially in the case of imbalanced data.
ROC-AUC Score: Measures the performance of classification models at various threshold settings.
Mean Absolute Error (MAE) and Mean Squared Error (MSE): Commonly used for regression models.
Example:
from sklearn.metrics import classification_report, roc_auc_score
# Classification report
y_pred = model.predict(X_test)
print(classification_report(y_test, y_pred))
# ROC-AUC score
roc_auc = roc_auc_score(y_test, model.predict_proba(X_test)[:, 1])
print(f"ROC-AUC Score: {roc_auc:.2f}")
Choosing the right metric is critical, as different tasks may require different evaluation criteria.
Conclusion
Scikit-learn is a versatile and powerful tool for machine learning, making it suitable for everything from basic classification tasks to complex ensemble models. In this post, we covered more advanced topics like cross-validation, hyperparameter tuning, pipelines, and ensemble methods, all of which are essential to building robust and accurate models.
Key Takeaways:
Cross-validation ensures your model generalizes well to unseen data.
Hyperparameter tuning allows you to fine-tune your models for optimal performance.
Pipelines streamline workflows and improve code readability.
Ensemble methods like Random Forest and Gradient Boosting enhance prediction accuracy.
By mastering these advanced Scikit-learn techniques, you can take your machine learning projects to the next level, ensuring that your models are both powerful and reliable.
Happy modeling!
Subscribe to my newsletter
Read articles from Rahul Tiwari directly inside your inbox. Subscribe to the newsletter, and don't miss out.
Written by
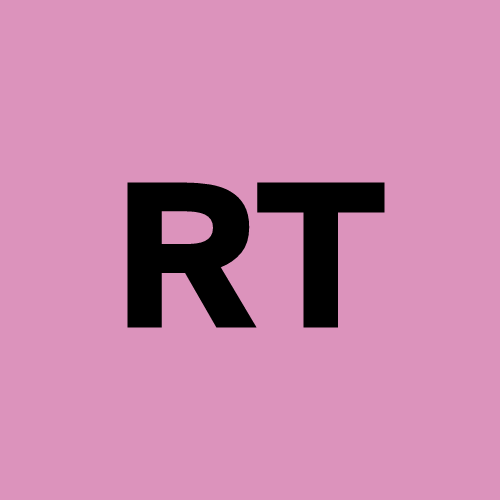