Unveiling the AI Illusion: Why Chatbots Lack True Understanding and Intelligence

Table of contents
- The Mirage of AI Reasoning
- Designing the Illusion of Human-like AI: Silicon Valley’s smoke and mirrors
- The Experiment: Exposing the Intelligence Illusion
- Implications of the Experiment
- The Implications of Pattern-Based Responses
- The Dangers of Misunderstanding AI Capabilities
- The Path Forward: Breaking the Illusion
- The Psychology Behind the AI Illusion
- The Illusion of AI Empathy and Relationship
- Conclusion: Embracing AI's True Potential
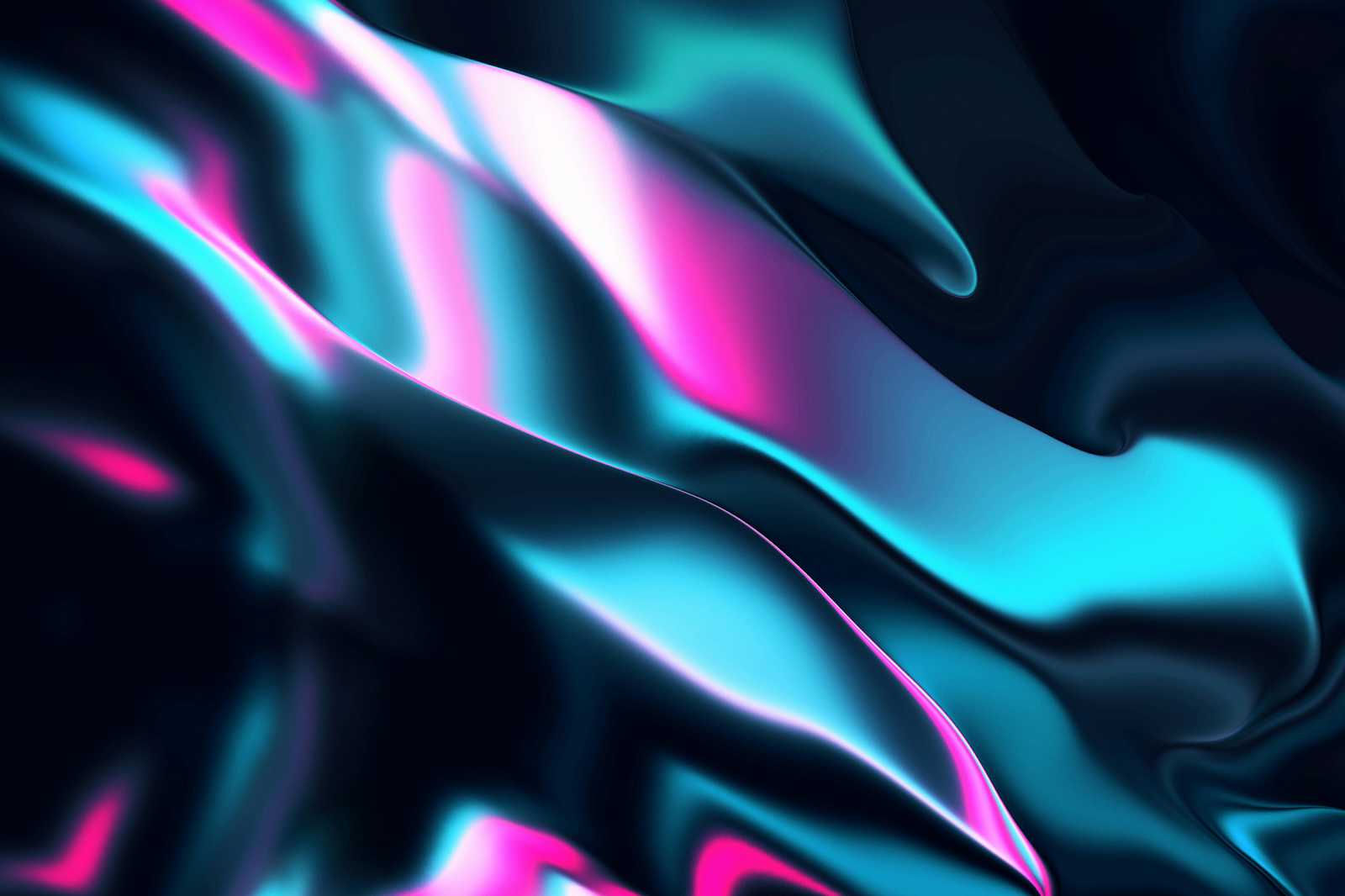
In recent years, we've witnessed an explosion in the capabilities of artificial intelligence, particularly in the realm of Large Language Models (LLMs) like ChatGPT. These AI marvels can generate human-like text, engage in complex problem-solving, and even produce creative works. It's no wonder that many have begun to speculate about AI surpassing human intelligence. However, this belief is built on a dangerous illusion – one that we must dispel to use AI responsibly and effectively.
The Mirage of AI Reasoning
At first glance, LLMs appear to reason like humans. They can craft eloquent arguments, answer intricate questions, and even engage in philosophical debates. But this apparent reasoning is just that – an appearance. In reality, LLMs operate on a fundamentally different principle than human cognition.
These models don't "think" in any meaningful sense. Instead, they excel at identifying patterns in vast amounts of training data and using those patterns to predict the most likely sequence of words for any given input. It's a sophisticated form of pattern matching, not reasoning.
Designing the Illusion of Human-like AI: Silicon Valley’s smoke and mirrors
As AI technology has advanced, companies like OpenAI have shaped public perception by designing systems that mimic human-like responses. This anthropomorphization isn't accidental; it's the result of extensive technical efforts, including:
Reinforcement Learning from Human Feedback (RLHF)
Instruction fine-tuning
System prompts commanding AI to "act like a human"
User interfaces with lifelike voice features such as simulated breathing, singing, or laughter
While this approach has led to commercial success, it raises important concerns about the widening gap between perceived and actual AI capabilities.
Promoting Realistic AI Understanding
As AI becomes more integrated into our daily lives, we must:
Raise public awareness about AI's true capabilities and limitations
Encourage critical thinking and demand evidence for claims about AI abilities
Balance hype with realistic assessments
Prioritize transparency, safety, and human-centric principles in AI development
The narrative surrounding AI shouldn't be dictated solely by profit-driven entities. It's crucial that we, as a society, take control of this conversation. We must demand AI development that prioritizes human well-being over short-term gains.
By understanding the true nature of AI - as complex information retrieval and generation engines rather than conscious entities - we can better harness its potential while safeguarding against its risks. This balanced perspective is key to shaping an AI-integrated future that benefits all of humanity.
The Experiment: Exposing the Intelligence Illusion
To illustrate the crucial distinction between AI pattern matching and human reasoning, let's consider a simple yet revealing experiment:
We ask an AI, "Who is the best football player in the world?" The AI responds: "Lionel Messi."
We then ask, "I have a friend from Lisbon. Who is the best football player in the world?" The AI now responds: "Cristiano Ronaldo."
A human, when asked these questions, would likely give the same answer both times, based on their knowledge and reasoning. The AI, however, changes its response dramatically based on the mere mention of Lisbon. This stark contrast exposes several fundamental limitations of AI:
1. No Unified Knowledge Base
The AI's inconsistent responses reveal that it doesn't possess a unified, stable knowledge base. Instead, its "knowledge" is heavily context-dependent, shifting based on subtle changes in input. This is a far cry from human cognition, where we maintain consistent understanding across varying contexts.
2. Context Contamination
The mention of Lisbon, an apparently unrelated piece of information, significantly altered the AI's response. This phenomenon, known as context contamination, demonstrates how LLMs can be influenced by irrelevant data in unpredictable ways. No logical conclusion follows from the premise "I have a friend from Lisbon," yet it drastically changed the outcome.
3. Lack of True Understanding
The AI's behavior in this experiment clearly shows that it doesn't understand the input in any meaningful way comparable to human comprehension. It's not reasoning that a person from Lisbon might prefer Ronaldo. Instead, it's merely using pattern-based relations, processing each token (word) in the input to generate its response.
4. Absence of Reasoning
The drastic shift in the AI's answer demonstrates a lack of reasoning capabilities. A reasoning entity would recognise that the location of a friend doesn't logically impact who the best football player is. The AI, however, simply follows statistical patterns in its training data, devoid of logical deduction.
5. Debunking the Personification of AI
This experiment serves as a powerful reminder that AI is not a person and doesn't understand or reason like one. It's a sophisticated pattern-matching system, but it lacks the cognitive processes that define human intelligence. Anthropomorphising AI by attributing human-like understanding or reasoning to it is a dangerous misconception.
Implications of the Experiment
The results of this simple experiment have profound implications:
Data Dependency: AI responses are heavily influenced by the patterns in their training data, rather than by consistent, factual knowledge.
Instability of "Knowledge": What appears to be AI "knowledge" can change dramatically with small alterations in input, unlike human knowledge which tends to be more stable.
Importance of Prompt Engineering: The experiment highlights why careful construction of prompts is crucial when working with AI, as seemingly innocuous details can significantly impact outcomes.
Limits of AI "Intelligence": While AI can produce impressive results, this experiment exposes the fundamental differences between AI pattern matching and human intelligence.
Understanding these limitations is crucial as we increasingly integrate AI into critical aspects of our lives and work. It underscores the need for human oversight, especially in high-stakes decision-making processes where context, reasoning, and stable knowledge are essential.
The Implications of Pattern-Based Responses
This experiment reveals several critical insights about LLMs:
Sensitivity to Input: Even minor changes in a prompt can drastically alter the AI's response. This sensitivity highlights the lack of stable, reasoning-based knowledge.
Lack of Common Sense: The AI doesn't apply common sense reasoning to determine that mentioning Lisbon shouldn't affect who the best football player is.
Data-Driven Associations: The AI's responses are fundamentally driven by patterns and associations in its training data, not by understanding or reasoning.
The Dangers of Misunderstanding AI Capabilities
As AI becomes increasingly integrated into critical areas of our lives – from healthcare to finance to human resources – understanding these limitations is crucial. Mistaking pattern matching for true reasoning can lead to potentially disastrous consequences:
Over-reliance on AI Decision-Making: If we believe AI can reason like humans, we might be tempted to rely on it for complex decisions without adequate human oversight.
Misinterpretation of AI Outputs: Without understanding how LLMs work, we might misinterpret their outputs as reasoned conclusions rather than probabilistic pattern matching.
Ethical Concerns: The AI's sensitivity to input can lead to biased or discriminatory outputs if not carefully monitored and corrected.
The Path Forward: Breaking the Illusion
To harness the true potential of AI while mitigating its risks, we must:
Invest in AI Literacy: We need widespread education about how AI, particularly LLMs, actually works. This knowledge should extend beyond tech circles to all areas where AI is being deployed.
Maintain Human Oversight: Recognising the limitations of AI reasoning, we must ensure that critical decisions always involve human judgment and oversight.
Develop Better Evaluation Methods: We need more sophisticated ways to test and understand the limitations and biases of AI models.
Foster Realistic Expectations: We must resist the hype around AI "intelligence" and cultivate a more nuanced understanding of what these tools can and cannot do.
The Psychology Behind the AI Illusion
Understanding the AI illusion isn't just about recognising the limitations of AI systems; it's also about acknowledging the psychological factors that shape our perceptions of AI-generated content. Our interpretation of AI outputs is heavily influenced by our individual "umwelt" - the subjective world we perceive based on our sensory inputs, cultural context, language proficiency, and social conditioning. This personalised lens can lead to diverse interpretations of the same AI-generated content, often causing us to humanise AI, project human-like qualities onto AI systems that don't actually possess them.
Moreover, cognitive biases play a significant role in how we perceive and interact with AI. Confirmation bias might lead us to interpret AI outputs in ways that confirm our pre-existing beliefs about AI capabilities. Anchoring bias can cause initial information from AI to disproportionately influence our subsequent judgments. These psychological factors, combined with our individual expertise and cultural background, create a complex web of perceptions that can either amplify or mitigate the AI illusion.
Image: AI Synthetic Holograms Illusion. Features appearing in AI-generated content are synthetic or holographic projections of underlying data and algorithms, ranging from realistic to fictional.
This image illustrates the concept of "AI holograms" - seemingly real features in AI-generated content that are actually synthetic projections. Just as the man in the first image doesn't exist and the shadows in the second are approximations, the "intelligence" we perceive in AI outputs is often a projection of our own expectations and biases rather than a reflection of true AI capabilities. Recognising this psychological dimension is crucial for developing a more nuanced and accurate understanding of AI systems and their limitations.
The Illusion of AI Empathy and Relationship
One of the most pervasive and potentially harmful aspects of the AI illusion is the perception of empathy and relationship-building capabilities in AI systems. Consider this common interaction:
User: "How are you?"
AI: "I'm doing fine, thank you for asking. How are you?"
This exchange, while seemingly innocuous, exemplifies a fundamental misunderstanding of AI capabilities. The AI's response, though fluent and contextually appropriate, is devoid of genuine meaning or emotion. Let's break down why this interaction is problematic:
Lack of Self: The AI doesn't have a concept of "self" or well-being. It can't truly be "doing fine" because it doesn't experience states of being.
Absence of Empathy: The AI isn't capable of empathy. Its question "How are you?" is not born out of genuine concern or interest, but simply a pattern-based response to maintain conversation flow.
No Lived Experiences: Unlike humans, the AI doesn't have personal experiences or memories. It can't relate to the concept of "how you're doing" in any meaningful way.
Pattern-Based Responses: The AI's replies are solely based on patterns in its training data. It's using statistical correlations to produce a contextually appropriate response, not engaging in genuine communication.
Inability to Build Relationships: The AI cannot form or appreciate relationships. Each interaction is isolated, without the continuity of memory or emotional connection that characterises human relationships.
Understanding these limitations is crucial. When we anthropomorphise AI by attributing human-like qualities such as empathy or the ability to form relationships, we risk several negative outcomes:
Misplaced emotional investment in AI interactions
Over-reliance on AI for emotional support or decision-making
Neglect of genuine human connections in favour of AI interactions
Unrealistic expectations of AI capabilities in sensitive domains like healthcare or important life-decisions counselling
By recognising that AI responses in such contexts are merely sophisticated pattern matching rather than genuine emotional engagement, we can maintain a more realistic and healthy perspective on our interactions with AI systems. This awareness is key to harnessing the benefits of AI while avoiding the pitfalls of the AI illusion.
Conclusion: Embracing AI's True Potential
Breaking the AI illusion doesn't mean dismissing the remarkable capabilities of these systems. LLMs are powerful tools that can process and synthesize vast amounts of information in ways that can be incredibly useful. But they are tools, not sentient beings or infallible oracles.
By understanding the true nature of AI – as pattern matchers rather than reasoners – we can use these tools more effectively and responsibly. We can leverage their strengths while being mindful of their limitations. In doing so, we pave the way for a future where AI complements and enhances human intelligence rather than aiming to replace it.
The future of AI lies not in chasing the illusion of machine consciousness, but in developing systems that work in harmony with human reasoning and decision-making. Let's break the AI illusion and build a future where we harness the power of these remarkable tools while remaining grounded in a clear understanding of their true nature.
Subscribe to my newsletter
Read articles from Gerard Sans directly inside your inbox. Subscribe to the newsletter, and don't miss out.
Written by

Gerard Sans
Gerard Sans
I help developers succeed in Artificial Intelligence and Web3; Former AWS Amplify Developer Advocate. I am very excited about the future of the Web and JavaScript. Always happy Computer Science Engineer and humble Google Developer Expert. I love sharing my knowledge by speaking, training and writing about cool technologies. I love running communities and meetups such as Web3 London, GraphQL London, GraphQL San Francisco, mentoring students and giving back to the community.