Introduction to A/B Testing for Data-Driven Decisions

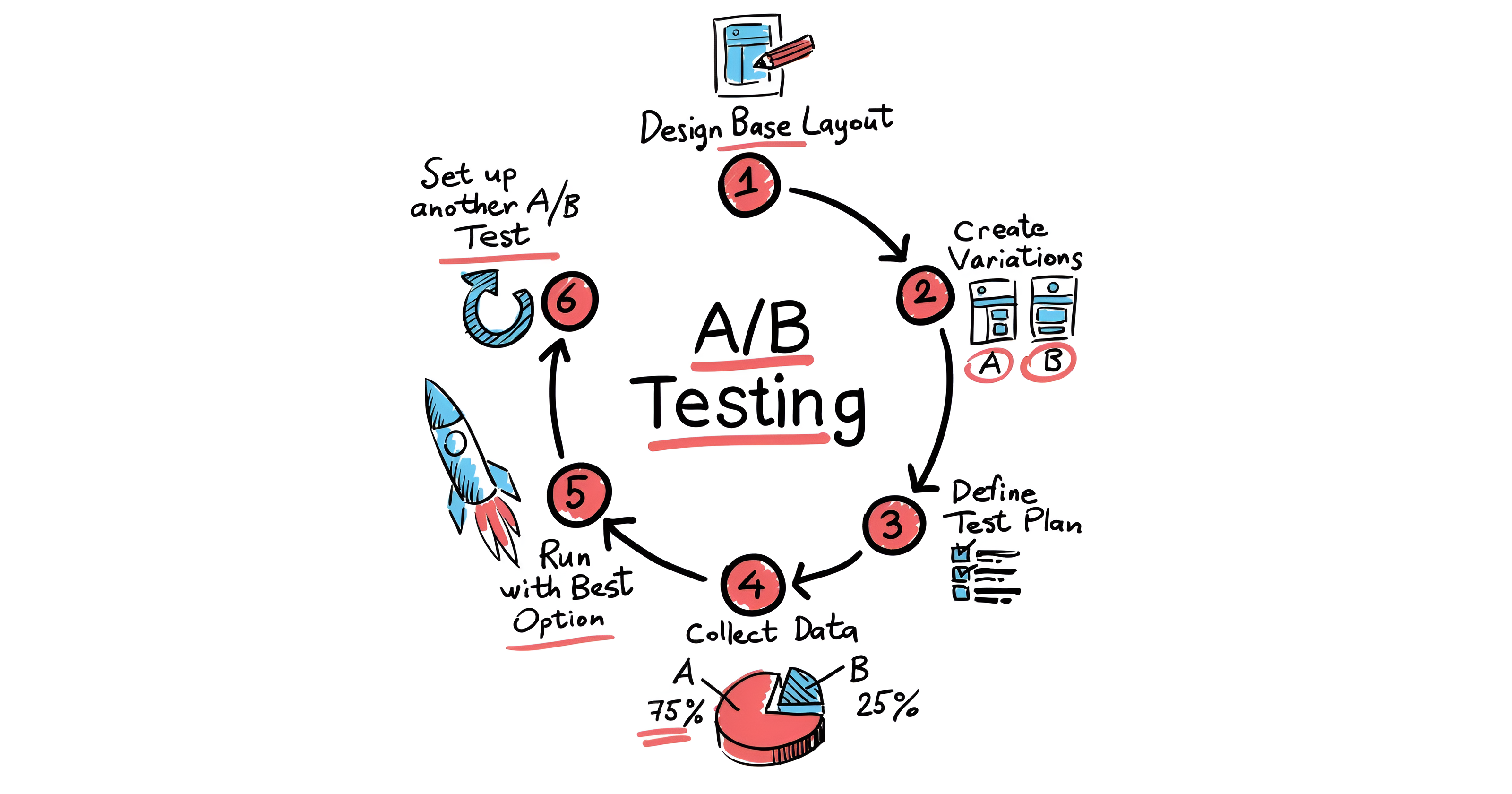
In today’s data-driven world, businesses rely on making informed decisions to stay competitive. One of the most popular methods used to assess the effectiveness of new features, strategies, or designs is A/B testing. This simple, yet powerful method enables data analysts and business teams to compare two versions of a variable to determine which performs better in achieving a specific goal.
In this article, we’ll dive deep into what A/B testing is, when to use it, how to set it up, and how to interpret the results to make data-driven decisions. By the end, you’ll have a solid understanding of how A/B testing can help refine business strategies, improve customer experiences, and drive growth.
What is A/B Testing?
A/B testing, also known as split testing, is an experiment where two or more variants (often referred to as A and B) of a variable are tested against each other. The goal is to determine which version yields the best results based on a key performance metric (e.g., conversion rate, click-through rate, engagement).
In an A/B test:
A represents the control (the original version).
B represents the variant (the modified version). The users or participants are randomly divided into two groups, with one group exposed to Version A and the other group exposed to Version B. The performance of both versions is then tracked to determine which one performs better.
Example: Suppose an e-commerce website wants to test whether changing the color of a call-to-action button from blue to green improves click-through rates. An A/B test would show Version A with the blue button to one group of visitors and Version B with the green button to another group. The performance of each button (click-through rate) would then be analyzed to determine the more effective version.
When to Use A/B Testing
A/B testing is incredibly versatile and can be used in various scenarios across industries. Here are some common use cases:
Website Optimization:
- Test different landing page designs, headlines, or images to determine which version converts visitors into customers more effectively.
Email Marketing:
- Compare two email subject lines to see which generates higher open rates or click-through rates.
Product Features:
- Test new features or designs in a mobile app or website to see how users engage with them (e.g., testing the placement of navigation buttons).
Pricing Strategies:
- Run A/B tests on different pricing models or discount offers to determine which drives more sales or customer acquisition.
Advertising Campaigns:
- Test different ad copy, visuals, or targeting criteria in paid advertising campaigns to optimize engagement and ROI.
In any of these scenarios, A/B testing allows businesses to base their decisions on data rather than guesswork, leading to more informed, evidence-based strategies.
How to Set Up an A/B Test
To ensure you conduct a valid and reliable A/B test, follow these essential steps:
1. Define Your Hypothesis
Before starting any test, you need to define what you’re testing and why. This requires creating a clear hypothesis that specifies what you’re changing (the independent variable) and what you expect to happen (the dependent variable).
Example: "Changing the color of the call-to-action button from blue to green will increase click-through rates by 10%."
2. Identify Key Metrics
Determine the specific metric(s) you’ll use to measure success. These are often known as Key Performance Indicators (KPIs). For example, if you’re running an email marketing A/B test, your key metrics might be open rates or click-through rates.
3. Segment Your Audience
Divide your audience randomly into two or more groups. Ensure that each group is representative of your overall audience so that the results can be generalized. Randomization helps eliminate bias and ensures that the observed effects are due to the variation being tested and not external factors.
4. Run the Test for an Adequate Duration
One common mistake is running an A/B test for too short a period. You need to gather enough data to ensure statistical significance (more on this later). The test duration should allow for seasonal or temporal variations in user behavior, such as weekdays vs. weekends or morning vs. evening traffic.
5. Analyze the Results
Once the test has run its course, it’s time to analyze the data. You’ll want to look at your key metrics for both versions and compare the results. Use statistical analysis to determine whether the difference between the two groups is significant or just due to random chance.
Interpreting A/B Test Results
After running the test, you’ll need to interpret the results using statistical methods to ensure they’re valid and meaningful. The two key concepts you’ll encounter are statistical significance and p-value.
1. Statistical Significance
Statistical significance refers to the likelihood that the difference in performance between Version A and Version B is not due to random chance. This ensures that your results are reliable and can be acted upon with confidence.
A common threshold for statistical significance is 95%, meaning you can be 95% confident that the observed differences are real.
2. P-value
The p-value is a metric used to determine statistical significance. It represents the probability that the observed difference could have occurred by chance. A p-value below 0.05 typically indicates that the difference is statistically significant.
For example, if you’re testing two landing page designs and find that Version B has a p-value of 0.03 compared to Version A, this suggests a significant difference, meaning you can confidently conclude that Version B performs better.
3. Effect Size
Effect size measures the magnitude of the difference between the two versions. While statistical significance tells you that there is a difference, the effect size tells you how meaningful or large that difference is. A large effect size can justify implementing the change across your platform or business, while a small effect size might prompt further investigation before taking action.
Best Practices for A/B Testing
To get the most out of your A/B testing efforts, consider the following best practices:
Test One Variable at a Time:
- To understand the impact of a specific change, test only one variable (e.g., button color or headline) at a time. Testing multiple variables simultaneously can make it difficult to determine which change influenced the outcome.
Be Patient with Results:
- Don’t rush to conclusions. Let the test run for the full duration to gather enough data and ensure that your results are statistically significant.
Consider External Factors:
- Take external factors (e.g., seasonality, marketing campaigns, or technical issues) into account when analyzing results, as they can influence the test’s outcome.
Iterate and Test Continuously:
- A/B testing is not a one-time task. As user behaviors and market conditions change, continuously test and optimize your product or service. Regular testing allows you to keep up with evolving trends and preferences.
The Power of A/B Testing for Data-Driven Decisions
A/B testing is a powerful tool for making data-driven decisions, as it provides direct evidence of what works and what doesn’t. Rather than relying on intuition or guesswork, businesses can use A/B testing to systematically evaluate changes and optimize for better performance. By testing one variable at a time, running tests for an adequate duration, and interpreting the results with statistical rigor, you can make decisions that lead to real improvements in user experience, marketing campaigns, and overall business success.
The beauty of A/B testing lies in its simplicity and versatility. Whether you’re refining a product feature, tweaking a marketing strategy, or optimizing a website design, A/B testing empowers you to learn from your data and drive meaningful, measurable results.
End
In conclusion, A/B testing is a critical tool for any data analyst or business professional looking to optimize performance and improve decision-making. By following a structured approach, understanding statistical concepts, and adhering to best practices, you can make informed, data-driven decisions that propel your business forward. As the business landscape becomes increasingly competitive, A/B testing offers a reliable way to experiment, learn, and adapt, ensuring that you stay ahead of the curve.
If you haven't started using A/B testing yet, now is the time to begin!
Subscribe to my newsletter
Read articles from Sai Sravanthi directly inside your inbox. Subscribe to the newsletter, and don't miss out.
Written by

Sai Sravanthi
Sai Sravanthi
A driven thinker on a mission to merge data insights with real-world impact.