The AI-Driven Journey: Navigating the Future of Driverless Cars

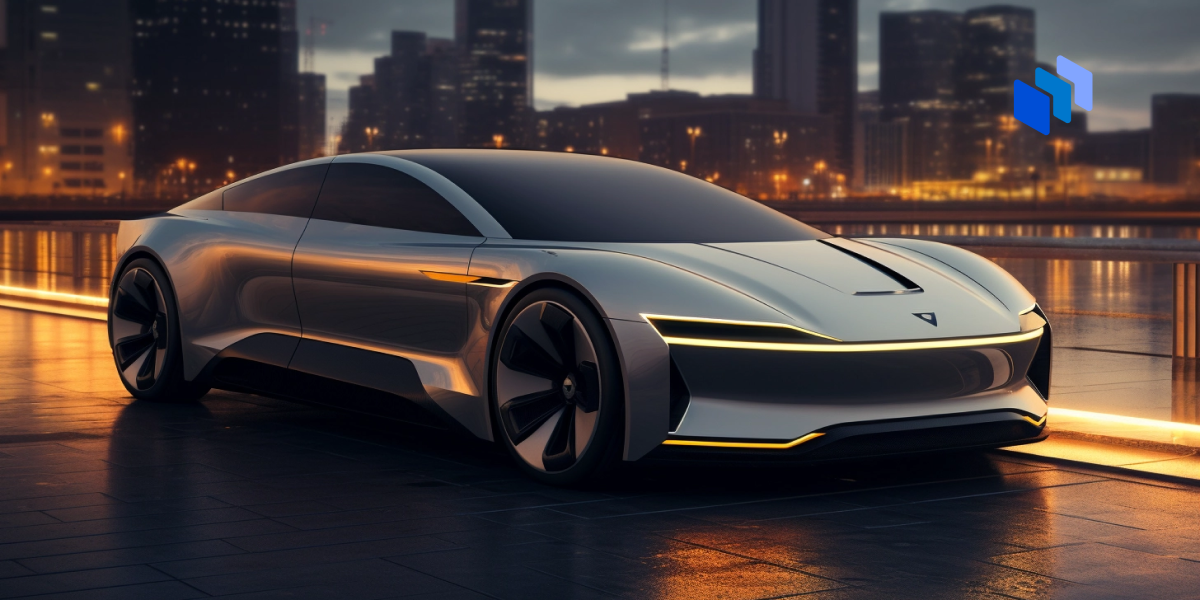
The Role of AI in Shaping the Future of Autonomous Vehicles
AI has, in recent decades, transformed how things are done globally across industries. But the most exciting use of this technology might just be an application within the autonomous vehicle. Said simply, AI is at the heart of the breakthrough technology - enabling vehicles to perceive their surroundings, make choices, and drive without human intervention. As we drift into a driverless car-dominated future, the AI will bring new revolutions in transport, safety, and urban mobility.
The Fundamentals of Understanding AI in Autonomous Vehicles
At its core, an autonomous vehicle is a robot on wheels. It must "sense," "think," and "act" like any other robot. AI plays a vital role in each of the three aforementioned stages, using ML, computer vision, and deep learning to make AVs perform well. Let's break this down and understand how AI influences every aspect of AV technology:
Sensing the Environment: Computer Vision and Sensor Fusion
Where the accumulation of real-time data from myriad sensors-LiDAR, cameras, radar, and ultrasonic sensors-infiltrates the sensorium of the vehicle, it is AI's computer vision algorithms that take this blank data and turn it into meaningful information; it allows the vehicle to "see" and interpret its surroundings, to determine that it is near a traffic sign, person, cyclist, or obstacle.
Another aspect that AI is powering is called sensor fusion and really refers to data extraction from multiple sensors in order to provide a holistic view of the environment, as accurate as possible. This kind of technology allows an autonomous vehicle to drive safely in complex dynamic conditions.
Thinking and Decision-Making: Machine Learning and Neural Networks
One of the key responsibilities of AI is decision-making. For example, self-driving cars have decisions to be made: whether to press the brakes, accelerate, change lanes, or sidestep something or someone on the road. Machine learning algorithms will take the insights in analyzing historical driving data to predict and make real-time decisions about the road.
Under a broader category of ML, deep learning uses neural networks to process large amounts of data so that the car learns from experience. These models learn over time and help the AV better understand rare or unusual driving scenarios-thereby, as a rule, the most complicated for self-driving cars.
Acting: Control Systems
Once the AI decision process is over, the control systems of a vehicle would then take control. They shall enforce the decisions made regarding steering, braking, and acceleration of the vehicle. With AI, a vehicle is sure to work in a manner that meets security standards and maximizes driving efficiency.
Impact of AI on Autonomous Vehicles
The influence of AI in AVs development only starts from navigation and decision-making. Some of the areas where AI impacts AVs include:
Safety: AI-controlled vehicles are designed to eliminate human mistake, the leading cause of accidents. Sophisticated algorithms allow AVs to scan dangers much faster than humans, respond faster, and prevent potential crashes.
Traffic Management: AI-autonomous vehicles can communicate with one another in a way that facilitates collaborative movements, minimizes the volume of congestion, and promotes smooth traffic flow. In the long run, autonomous cars may facilitate a smoother city shift towards intelligent transportation systems.
Personalized Driver Experience: This could be an area where AI would make the difference by making possible a personalized driving need from the speed to the route, tailored to the needs of the passenger. AVs become an extension of our digital lives, so we can integrate them into personal assistants or entertainment systems.
Recent Trends in AI for Autonomous Vehicles
The landscape of AI-powered autonomous vehicles is rapidly evolving, with several key trends emerging that will shape the future of the industry:
Edge AI and Onboard Processing Innovations
Edge AI is one of the hottest trends in autonomous vehicles today as companies are moving towards even more localized computing for data. This corresponds to processing data on the car (rather than in cloud servers, as needed for AVs). Because of this, high-performance onboard processors are being incorporated into vehicles that can deliver what is needed to function at the intersection in real time such as Sensor data capture and pre-processing like image recognition Path planning with advanced perception techniques And sensor fusion for complete situational awareness on board. By caching and distributing traffic management outward to the Venom modules, response times improved significantly with lower latency making AVs self-sustainable even in low coverage areas.
Enhanced AI Mapping and Localization
GPS is mainstream in traditional navigation while intelligent mapping is finding new applications that give accurate as well as dynamic navigation solutions. Recent developments are the SLAM approaches, which enable an AV to build up a precise map of the environment and localize itself in that map at the same time. AI related methods in the localization process are enabling AVs to be less susceptible to cities where GPS is known to be unreliable.
Autonomous Safety augmented by improved predictive AI techniques.
Reliability has not faded as a priority and AI is assisting by developing better forecasting models. These models calculate probable risks on the roads before they happen. For instance, it looks at how pedestrians are behaving and knows when a particular person is likely to cross into the road so that the car will slow down. Similarly, predictive AI systems can assess how other vehicles in the vicinity are likely to operate, and minutely correct its operation to reconcile with the like.
Autonomous Intelligent Vehicle-To-Everything technology
Vehicle to Everything (V2X) is another important trend of AVs where vehicles do not only interact with other vehicles and object but with traffic lights, pedestrians and road infrastructure also. The task which AI is used here to perform is the monitoring of shared data and the analysis of the interactions between the vehicle and the items within the vehicle’s environment. This aids AVs to determine appropriate traffic conditions, minimize incidence of accident, and select the best paths. Integrating V2X technology with Artificial intelligence, has been the platform for advanced intelligent traffic management system also on the way towards smart city formation.
The next process is the presence of laws and AI-driven standardization
As the technology of AVs is advancing, governments are paying considerable attention to creating guidelines for regulation. Currently, a clear trend is the cooperation of organizations, automotive companies, and AI developers to establish norms in the field. AI is being used by regulators to validate new environments and to experiment with different kinds of operational situations and safety measures. For instance, EU together with the US is likely to step up attempts to keep defining exactly the laws governing the application of artificial intelligence-driven driving systems in order to protect safety, privacy and culpability.
AI-Sim for Testing and Training
Real road tests of AVs are both time consuming and costly. To speed up enhancement, such companies are now deploying AL-based modeling platforms. These virtual environments approximate real life driving conditions and situations by which developers calibrate and evaluate their algorithms. Self-driving technology has the ability to drive millions of miles by simulation in a more limited number of hours, which enhances the reliability of the Lidar systems.
Challenges and Ethical Considerations
Despite the potential of AI in AVs, there are ethical and regulatory challenges. For example, in a collision incident, the AV needs to take a decision whether to save its passengers or not to injure people on the road. Such ethical dilemmas must be addressed so that the technology is well received by the public.
Further, even after so many advances by AI, so much is still left unrequited in building an almost flawless vehicle, particularly considering the uncertainties of road events. The human factor — fellow drivers and pedestrians and weather conditions — cannot be dependable enough in all cases for 100% reliability.
The Road Ahead…..
No doubt, AI will be one of the core enablers for the next horizon. Companies like Tesla, Waymo, or even Uber are putting some investment in AI to make fully autonomous cars a reality. When the model of AI goes very complex, it will enable the AVs to navigate challenging and complex driving environments that may portray towards a world where driverless cars dominate our roads.
Better means of transportation will be the norm with artificial intelligence in an autonomous vehicle. However, this may be possible only by surmounting the technological and social challenges associated with it. What is needed is a collaborative effort between the government and technology companies, as well as the general public, towards building such an environment where authentic and reliable, accessible AI-powered vehicles become the order of the day.
Conclusion
AI represents a transformative role in the self-driving car story of evolution. Safety is enhanced, and smooth navigation becomes well within the possibility of sophisticated programs that promise to act as the core of self-driving technology. Of course, with such great progression, the future holds much in store for us as regards how this could change the way we think of mobility and cities in the future.
Subscribe to my newsletter
Read articles from RIDDHI PATEL directly inside your inbox. Subscribe to the newsletter, and don't miss out.
Written by
