AI Ethics

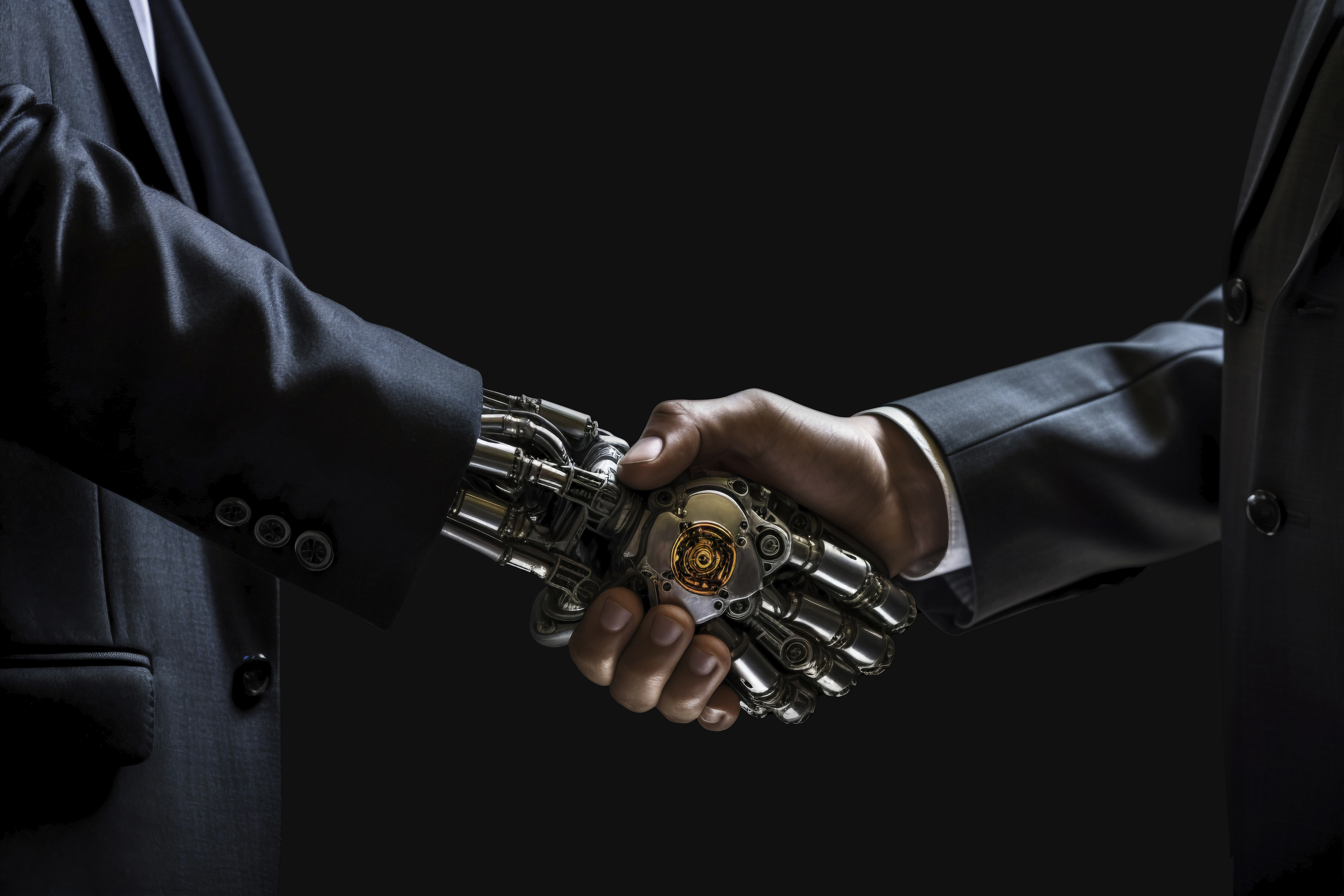
Understanding AI Ethics: What It Means and Why It Matters
AI ethics refers to the moral principles and guidelines that govern the development and use of artificial intelligence technologies. As AI systems become more prevalent in various aspects of life—such as healthcare, criminal justice, and finance—the need for ethical considerations becomes increasingly important. This section will define AI ethics and explore its significance.
What Does AI Ethics Involve?
At its core, AI ethics encompasses a wide range of issues, including fairness, accountability, transparency, and the impact of AI on society. It involves critical discussions about how AI systems should be designed, implemented, and monitored to ensure they do not cause harm.
Fairness: This involves ensuring that AI systems operate impartially and do not reinforce existing biases. Fairness is a crucial consideration in areas like hiring and lending, where biased algorithms can lead to discriminatory practices.
Accountability: As AI systems make more autonomous decisions, determining who is responsible for those decisions becomes complex. Accountability in AI requires clear definitions of liability and oversight mechanisms to address potential harms.
Transparency: The algorithms behind AI systems often operate as "black boxes," making it challenging to understand how decisions are made. Transparency involves making AI processes more understandable, allowing users to see how outcomes are determined.
Societal Impact: AI ethics also encompasses the broader implications of AI on society, including its potential to exacerbate inequalities or disrupt traditional job markets. Ethical considerations must guide the development of AI to ensure it contributes positively to society.
Why Does AI Ethics Matter?
AI ethics is not just an abstract concept; it has real-world implications. Here are several reasons why AI ethics is critical:
Preventing Harm: AI systems can have significant consequences on individuals' lives. Ethical considerations help mitigate potential harm caused by biased or poorly designed algorithms.
Building Trust: When people believe that AI systems are developed ethically, they are more likely to accept and use these technologies. Trust is essential for the successful integration of AI into society.
Fostering Innovation: Ethical frameworks can drive innovation by encouraging developers to create AI systems that address social issues. This proactive approach can lead to the development of technologies that benefit everyone.
Regulatory Compliance: As governments worldwide begin to regulate AI, understanding and implementing ethical guidelines will be crucial for organizations to comply with legal standards and avoid penalties.
The Importance of AI Ethics in Our Technology-Driven World
AI technologies are becoming increasingly integrated into various facets of our lives. Their influence can be seen in areas such as healthcare diagnostics, credit scoring, law enforcement, and social media algorithms. With such widespread application, the importance of AI ethics cannot be overstated.
Building Trust in AI Systems
Trust is a cornerstone of successful AI adoption. When consumers feel confident that AI technologies are safe and reliable, they are more likely to embrace them. To build this trust, organizations must prioritize ethical practices in AI development.
Clear Communication: Companies should communicate openly about their AI systems, explaining how they work and what data they use. This transparency helps alleviate concerns and fosters user confidence.
User Empowerment: Providing users with control over their data and insights into how AI systems make decisions can enhance trust. When individuals feel they have agency in their interactions with AI, they are more likely to accept its use.
Addressing Societal Inequities
AI has the potential to address long-standing social issues, but it can also exacerbate existing inequities if not handled properly. Ethical AI development aims to create technologies that actively work to reduce inequalities rather than reinforce them.
Promoting Inclusion: Ethical considerations in AI development should include the voices of marginalized communities. Engaging these groups can ensure that AI technologies meet diverse needs and do not perpetuate harmful stereotypes.
Mitigating Discrimination: By prioritizing fairness and accountability, organizations can create AI systems that minimize the risk of discrimination in areas like hiring, lending, and law enforcement.
Long-Term Sustainability of AI Technologies
Sustainable AI development hinges on ethical considerations. As public awareness of ethical issues grows, consumers will demand more responsible practices from organizations.
Consumer Expectations: Consumers are becoming increasingly aware of ethical concerns related to AI. Organizations that prioritize ethical practices will be better positioned to meet these expectations and retain customer loyalty.
Future-Proofing Technologies: By developing ethical AI systems now, organizations can future-proof their technologies against potential backlash or regulatory challenges. This proactive approach can save time and resources in the long run.
What is AI Bias? A Deep Dive into the Concept and Its Implications
AI bias occurs when an AI system produces results that are systematically prejudiced due to flawed assumptions in the machine learning process. This section will define AI bias, explore its different types, and discuss its implications.
Types of AI Bias: Understanding Different Forms of Prejudice in AI
Understanding the different forms of AI bias is crucial to addressing the underlying issues. Here are the primary types of bias that can occur in AI systems:
Prejudice Bias: This form of bias arises when the training data reflects existing social prejudices. For instance, if a hiring algorithm is trained on resumes submitted to a company with a history of discriminatory hiring practices, it may perpetuate those biases in its recommendations.
Measurement Bias: Measurement bias occurs when data collection methods are flawed, leading to skewed results. An example could be facial recognition algorithms trained predominantly on images of light-skinned individuals, which can lead to poor performance on darker-skinned faces.
Algorithmic Bias: This bias stems from the algorithms themselves, which may favor certain outcomes over others based on how they weigh different features in the data. If an algorithm is optimized for speed over accuracy, it may make unfair decisions in critical situations.
Sample Bias: Sample bias happens when the dataset used to train the AI model is not representative of the broader population. For instance, if a healthcare AI is trained only on data from urban populations, it may not perform well for rural patients.
Real-World Examples of AI Bias: Case Studies in Unintended Consequences
Several high-profile incidents illustrate the real-world implications of AI bias. Here are two notable case studies:
Case Study: The Amazon Hiring Algorithm
In 2018, Amazon scrapped an AI recruiting tool that showed bias against women. The algorithm, trained on resumes submitted to the company over ten years, learned that male candidates were preferred based on historical hiring data. This incident highlights the critical need for bias detection and correction in AI systems, especially in hiring practices that can shape careers and livelihoods.
Case Study: COMPAS Risk Assessment
The Correctional Offender Management Profiling for Alternative Sanctions (COMPAS) is an AI tool used to assess the risk of reoffending among criminal defendants. Investigations revealed that the system was biased against Black defendants, falsely labeling them as higher risk than white defendants. This case underscores the severe implications of biased AI in the criminal justice system, where flawed assessments can lead to unfair sentencing and perpetuate systemic inequalities.
Combating AI Bias: Strategies for Developing Fair AI Systems
Combating AI bias requires intentional strategies and practices. This section will explore effective approaches to creating fair AI systems.
Diversity in Data: Why It’s Crucial for Fair AI
One of the most effective ways to combat AI bias is by ensuring diversity in training data.
Representative Datasets: Organizations should prioritize creating datasets that reflect the diversity of the population. This includes accounting for race, gender, age, and other demographic factors to ensure that AI systems are trained on a balanced representation of the user base.
Continuous Monitoring: It’s essential to continually monitor datasets for changes and updates. As society evolves, so too should the data that informs AI systems. Regular audits of training data can help identify and rectify biases over time.
Transparency in Algorithms: Building Trust Through Openness
Transparency is key to fostering trust in AI systems. Here’s how organizations can promote transparency:
Open Source Algorithms: By making algorithms open source, organizations can allow external parties to review and assess the fairness of AI systems. This collaborative approach can lead to more ethical practices and increased accountability.
Explainable AI: Developing AI systems that provide clear explanations for their decisions can enhance user understanding and trust. Explainable AI frameworks aim to make the decision-making processes of AI systems more interpretable to non-experts.
Regular Audits and Assessments: Ensuring Fairness in AI Systems
Regular audits of AI systems can help identify biases and ensure they operate fairly.
Bias Audits: Organizations should conduct bias audits at various stages of AI development, including pre-deployment and post-deployment. These audits can help detect and correct biases before they lead to harmful outcomes.
Stakeholder Engagement: Involving a diverse range of stakeholders in the auditing process can provide valuable insights into potential biases and encourage a more comprehensive understanding of the issues at hand.
AI Accountability: Who Bears the Responsibility?
As AI systems take on more autonomous roles, questions of accountability become paramount. This section explores who is responsible for AI decisions and the role of regulations in ensuring accountability.
Who is Responsible for AI Decisions? A Look at Stakeholders
Determining accountability in AI systems involves various stakeholders:
Developers: AI developers play a crucial role in ensuring that the systems they create adhere to ethical guidelines. This includes implementing best practices for data collection, model training, and bias mitigation.
Organizations: Companies that deploy AI systems must take responsibility for their outcomes. This involves establishing ethical guidelines and fostering a culture of accountability within the organization.
Policymakers: Governments and regulatory bodies have a vital role in creating laws and regulations that promote accountability in AI technologies. Establishing clear guidelines for liability in cases of AI-related harm is essential for protecting individuals and society.
The Role of Regulations in Ensuring AI Accountability
As AI technologies become more pervasive, regulations governing their use are increasingly necessary. Here’s how regulations can promote accountability:
Establishing Standards: Regulatory bodies can develop ethical standards for AI development and usage. These standards can guide organizations in creating responsible technologies that prioritize fairness and accountability.
Monitoring Compliance: Regulations should include mechanisms for monitoring compliance. Regular assessments can ensure organizations adhere to ethical guidelines and take corrective action when necessary.
Public Oversight: Encouraging public oversight of AI technologies can help hold organizations accountable. Transparency initiatives, such as public reporting of AI system outcomes, can foster a culture of accountability and trust.
AI Privacy Concerns: Protecting Personal Data in the Age of AI
With AI systems often relying on vast amounts of personal data, privacy concerns are paramount. This section delves into how AI collects data and the importance of user consent.
Data Collection and Usage: Understanding How AI Gathers Information
AI systems rely on data to function effectively, but improper handling of this data can lead to privacy violations.
Data Minimization: Organizations should adopt a principle of data minimization, collecting only the data necessary for the AI system to operate. This reduces the risk of privacy violations and builds user trust.
Secure Data Storage: Ensuring the secure storage of personal data is essential. Organizations must invest in robust cybersecurity measures to protect user data from unauthorized access and breaches.
Transparency in Data Usage: Organizations should clearly communicate with users about how their data is collected, used, and stored. This transparency fosters trust and encourages responsible data practices.
User Consent and Control: Empowering Individuals Over Their Data
User consent is critical in protecting privacy. Here’s how organizations can empower individuals:
Informed Consent: Organizations should ensure that users provide informed consent before their data is collected. This includes clear explanations of what data will be collected and how it will be used.
User Control Over Data: Providing users with the ability to manage their data—such as options to delete their data or withdraw consent—empowers individuals and fosters trust in AI systems.
Regular Privacy Audits: Conducting regular audits of data privacy practices can help organizations identify potential issues and make necessary adjustments to protect user privacy.
The Future of AI Ethics: Emerging Trends and Potential Solutions
The future of AI ethics is likely to be shaped by ongoing technological advancements, societal expectations, and regulatory developments. This section explores potential trends and solutions.
Collaborative Approaches: Engaging Multiple Stakeholders
Engaging diverse stakeholders can lead to the development of comprehensive ethical guidelines for AI technologies.
Inclusive Discussions: Involving ethicists, developers, policymakers, and the public in discussions about AI ethics can ensure that multiple perspectives are considered. This collaborative approach can lead to more robust ethical frameworks.
Cross-Industry Partnerships: Organizations can benefit from partnering with others in different industries to share best practices and insights. These partnerships can promote a culture of ethical AI development across sectors.
Educational Initiatives: Promoting Awareness of AI Ethics
Educating developers and the public about AI ethics is essential for creating a culture of ethical AI development.
Training Programs: Organizations should invest in training programs that emphasize ethical considerations in AI design. This education can help developers recognize the importance of ethics in their work.
Public Awareness Campaigns: Raising public awareness about AI ethics can empower consumers to make informed decisions about the technologies they use. Informative campaigns can promote discussions about responsible AI practices.
Advancements in Ethical AI Research: Supporting Innovative Solutions
Ongoing research in ethical AI is critical for identifying new challenges and solutions.
Funding Research Initiatives: Supporting research focused on ethical AI can drive innovation in responsible practices. Funding initiatives that prioritize ethical considerations can lead to the development of technologies that benefit society.
Collaboration with Academia: Partnerships between organizations and academic institutions can foster interdisciplinary research on AI ethics. This collaboration can lead to new insights and strategies for ethical AI development.
Conclusion
AI ethics is not just a buzzword; it’s a critical field that impacts our daily lives. By addressing issues like AI bias, accountability, and privacy, we can ensure that AI technologies develop in ways that are fair, transparent, and respectful of individual rights. As we move forward, fostering a culture of ethical AI development will be essential for building trust and ensuring a positive future for technology.
FAQs
1. What is AI bias?
AI bias refers to systematic prejudice in AI systems, often due to flawed training data or algorithms. It can lead to unfair outcomes in critical areas like hiring, lending, and law enforcement.
2. How can we combat AI bias?
By ensuring diversity in training data, promoting transparency in algorithms, and conducting regular audits, we can work toward reducing AI bias and creating fairer systems.
3. Who is responsible for AI accountability?
Accountability can involve developers, companies, and regulatory bodies, depending on the context of the AI system. Establishing clear lines of responsibility is crucial for ethical AI usage.
4. Why is AI privacy important?
AI systems often collect vast amounts of personal data, making privacy protection crucial to maintaining user trust. Ethical practices in data collection and usage are essential for fostering confidence in AI technologies.
5. What is the future of AI ethics?
The future will involve evolving technological standards and regulatory frameworks that prioritize fairness, accountability, and privacy in AI development. Engaging a diverse group of stakeholders will be key to shaping responsible AI practices.
…
Join Weskill’s Newsletter for the latest career tips, industry trends, and skill-boosting insights! Subscribe now:https://weskill.beehiiv.com/
Subscribe to my newsletter
Read articles from Weskill directly inside your inbox. Subscribe to the newsletter, and don't miss out.
Written by

Weskill
Weskill
WeSkill.org is an innovative online learning platform dedicated to empowering individuals through accessible, high-quality education. We provide a wide range of courses covering various fields such as technology, business, health, and personal development, aimed at helping learners acquire new skills and advance their careers. With a focus on practical knowledge and real-world applications, WeSkill.org offers interactive content, expert-led instruction, and community support to ensure that every student achieves their educational goals. Whether you’re a beginner looking to start your journey or a professional seeking to enhance your expertise, WeSkill.org is your partner in lifelong learning.