The Role of Artificial Intelligence and Machine Learning in Cybersecurity

Table of contents
- Introduction
- Current Challenges in Cybersecurity
- AI and ML in Cybersecurity: Key Use Cases
- Benefits of AI and ML in Cyber Security
- Limitations and Challenges of AI and ML in Cybersecurity
- Future Trends in AI and ML for Cybersecurity
- Recommended Steps for AI and ML Implementation in Cybersecurity
- Conclusion
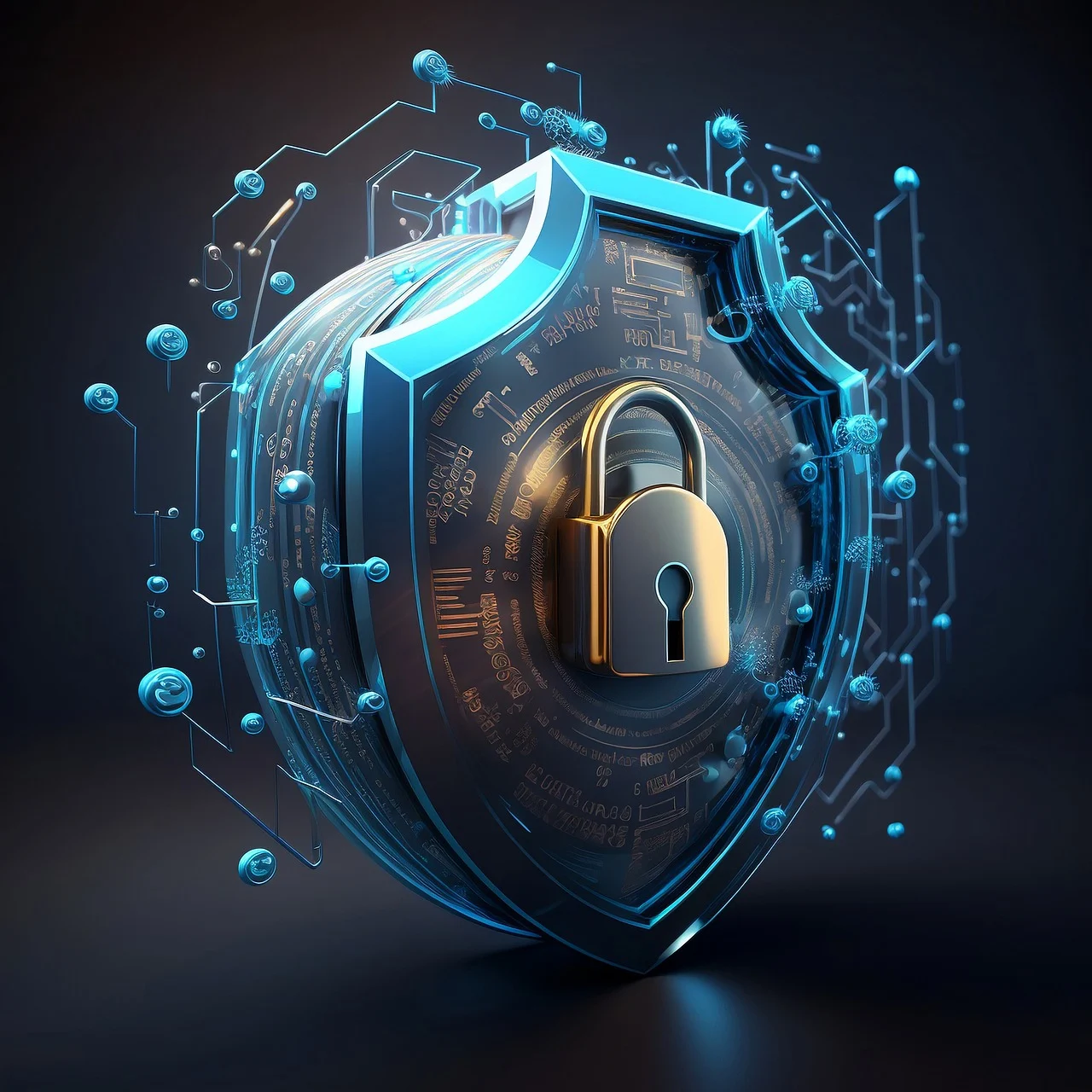
Introduction
As the scale and complexity of cyber threats are highly evolving, the urgency felt for coming up with sophisticated solutions has been becoming more pressing. Now, come two game-changing technologies, artificial intelligence and machine learning, to transform the whole landscape of cybersecurity. But, exactly what is AI, and how is it different from ML? While AI refers to the broader concept of machines performing tasks in a way that emulates human intelligence, ML is a subset of AI focused on enabling systems to learn and improve from experiences without explicit programming.
With cybersecurity, AI and ML hold great promise in countering the increasing sophistication of cyberattacks. The traditional security measures have tried to keep pace for some time now, but the demand is asking for more mechanisms that are both efficient and proactive. It is here that AI/ML come in with the capability to detect, predict, and respond to threats faster and with greater accuracy than ever before.
Current Challenges in Cybersecurity
Before the specific roles AI and ML have in cybersecurity are discussed, it is important briefly to understand the challenges that already are in play in the field.
Volume and Variety of Cyber Threats
Cyberattacks have now begun to become far more variegated and compound. From malware and phishing attacks to ransomware and APTs, cyberattackers use any variety of ways to exploit an array of vulnerabilities. It has reached a volume that organizations can no longer keep track of. Security teams are bombarded with millions of alerts and potential incidents every day. It is, in reality, impossible to address each of these with precision .
Limitations of Traditional Security Solutions
Most classic cybersecurity solutions are reactive by nature. For example, signature-based antivirus systems use known patterns or "signatures" of malware; hence, they are useless in providing protection from new or unknown threats, such as zero-day vulnerabilities. Rule-based systems require constant updates and manual supervision, which poses a significant challenge to teams that are already stretched to the limit.
The Need for Automated and Scalable Solutions
With the unparalleled scale and speed of its evolution, cyber threats already have outgrown traditional measures of security. It is time organizations required automated solutions that would be scalable—capable of processing big datasets to respond to incidents in real-time. And that's where AI and ML excel, offering tools capable of processing vast amounts of data while minimizing loads on human analysts.
AI and ML in Cybersecurity: Key Use Cases
AI and ML have become the most powerful technologies that can change how cybersecurity professionals defend against threats. Following are some of the key use cases where AI/ML has started to make a big impact:
Threat Detection and Response
One of the most critical roles AI plays in cybersecurity involves threat detection. While traditional systems detect malware based on predefined signatures, AI-powered systems can make further analysis by finding a pattern in network traffic and user behavior. This is where machine learning models tend to get really good at identifying unusual activity that could suggest a threat might be present, such as a new strain of malware or an insider attack.
These solutions monitor network traffic for abnormal usage patterns that deviate from the normal use pattern to flag any potential security incident. This allows organizations to respond swiftly and more precisely, reducing the mean time to detect and neutralize threats.
Automating Incident Response
Besides detection, AI can automate responses to cybersecurity incidents. Without needing human analysts to investigate threats and perform remediation, the AI-driven tools automatically classify the alerts in order of severity. Therefore, this makes sure the most critical ones are addressed first and, as a result, reduces the time required for containing breaches.
Also, machine learning models trained on past incidents can recommend remediation steps to take, further streamlining the response. It is this level of automation that accelerates threat resolution and frees human analysts up to deal with more complex tasks.
AI-Powered Security Operations Centers - SOCs
The traditional SOC is usually overwhelmed by the barrage of data and alerts thrown up by security tools, for which AI and ML come in to alleviate. AI and ML help alleviate this by enhancing SOC capabilities. With an AI-driven SOC, it would be able to analyze big datasets in near real time, sifting through the thousands of logs and alerts in search of meaning. They can minimize false positives so that analysts will not have to spend their precious time on non-threatening activities.
Fraud Detection
AI and ML have proved to be particularly effective in finding fraudulent activities, particularly in industries such as finance and e-commerce. Machine learning models can analyze large volumes of transaction data in real time for suspicious patterns or behaviors that could suggest fraud. For instance, an AI system may identify something out of the ordinary, such as a login from a location it does not recognize or an out-of-pattern transaction. It then flags the activity for further investigation or even automatically blocks the activity.
Phishing Detection
Phishing remains one of the most widely used types of cyberattacks, and AI will be of great use in such detection and prevention. AI systems can also go through emails to detect any phishing attempt by analyzing sender behavior, email content, or even minor structural anomalies in language. Unlike classic filters that depend on preset rules, solutions using AI may adapt to new methods of phishing once they are spotted.
Benefits of AI and ML in Cyber Security
The integration of AI and ML within cybersecurity provides a set of key benefits that are transforming how organizations protect themselves against attack.
Speed and Accuracy Increase
AI-driven systems process large volumes of information at a speed that no human analyst can. They scan logs, network traffic, and user activity in real time while detecting threats and anomalies with a high degree of accuracy. Machine learning models will be improving their accuracy continuously over time by learning from new data and adjusting to emerging threats.
Proactive Threat Prevention
AI's predictive analysis empowers organizations to move from a reactive to a proactive stance in cybersecurity. Predictive analytics, with the use of machine learning, will contribute to locating the possible attack points before they are attacked. For example, AI can flag outdated software that may be an easy target or even detect subtle signs of a breach before it happens.
Reduced Human Error
Human error is one of the leading causes of security breaches and most often results from misconfigurations, oversight, or slow response times. AI has the capability to automate routine activities that are prone to errors. But that's not all: AI systems also provide actionable insights that enable a security team to make better, data-driven decisions.
Scalability
AI systems are built to scale with modern IT infrastructures. Whether an enterprise cloud environment or an IoT network, AI-driven solutions support more volumes of data as they adapt to the scaling of infrastructure complexity.
Limitations and Challenges of AI and ML in Cybersecurity
While AI and ML have immense benefits, all is not well in this garden either.
Data Quality and Availability
For machine learning models to learn effectively, they need high-quality data for training. Inaccurate or non-diverse datasets that are not updated can be deficient in the detection of new or sophisticated threats by ML models. Thus, many organizations are faced with the challenge of acquiring real-world threat data, which consequently reduces the effectiveness of AI-driven systems.
Adversarial Attacks on AI Systems
Attackers are shifting their target to the AI systems themselves: Feeding the AI system false or manipulated data, cybercriminals can trick the machine learning models into bad decisions—a tactic known as adversarial attacks. An attacker could intentionally feed an AI system bad data in order to make it miss or misclassify a threat.
Over-Reliance on Automation
While AI and ML can automate most cybersecurity tasks, the biggest risk here is dependency. An automated system that uses fully automated systems might lack the capability to address such complex, nuanced threats that call for human judgment. That is why striking a balance between AI-driven automation and human judgment is crucial in cybersecurity operations.
Ethical Considerations
AI also presents ethical dilemmas, mainly regarding bias and privacy. For example, AI models, while trained using biased data, tend to make faulty or unfair decisions. Also, most AI-driven security systems require massive volumes of data, which again is a concern for user privacy and data protection.
Future Trends in AI and ML for Cybersecurity
While AI and machine learning continue to advance, their role in cybersecurity will be all the more critical. The following is the set of emergent trends going to shape the future of AI and ML in this field.
The Role of Deep Learning
Deep learning is a subset of machine learning that deals with neural networks to model and analyze data for various complex patterns. The field of deep learning has already covered most of the works in image and speech recognition; however, it is seeing very fast growth in cybersecurity. Deep learning is useful in recognizing minute and sophisticated cyber threats in the likes of zero-day attacks or APTs.
However, deep learning can analyze large volumes of data created by network traffic to find hidden patterns or anomalies that would elude traditional systems. It also allows the detection of hitherto unknown strains of malware in real time, based on their behavior rather than their signature.
AI-Driven Autonomous Security Systems
Perhaps one of the most exciting developments in the world of AI and cybersecurity relates to autonomous security systems. Beyond threat detection and response, these can operate independently of, and without, human interference to repel cyberattacks. Fully autonomous security systems are still in their infancy today, but the potential hidden in these technologies really could be limitless.
For example, AI-driven systems can automatically detect, investigate, and neutralize threats across an entire network without human intervention. Autonomous systems also do live learning from newly emerging threats so that each new attack serves to make the systems stronger.
Automation and AI-powered solutions are immensely promising, but the future of cybersecurity will irrefragably require a cooperation model in which AI supplements human experience. Instead of replacing human analysts, AI will collaborate with them to offer insights and recommendations for better decision-making.
For instance, AI can handle the routine and repetitive tasks, such as scanning logs or filtering through large volumes of alerts, leaving the more strategic and complex areas of cybersecurity to humans. This combination of humans and AI will surely promote efficiency and further equip organizations with combat skills to deal with threats that are more sophisticated and beyond AI's reach.
AI in Offensive Cybersecurity
AI will also play a part in offensive cybersecurity, especially in ethical hacking and pen testing. AI-driven automated pen-testing tools can simulate attacks that unveil weaknesses in systems much faster than traditional methods of manual testing. Ethical hackers—so-called "red teams"—can utilize AI in finding hidden weaknesses that human testers may miss.
On the other hand, there's the fear that AI might be used by cybercriminals as a new method of intelligence in carrying out more valuable attacks. As AI becomes increasingly available, the attackers may use AI-powered tools when finding vulnerabilities, passing defenses, or even performing phishing attacks whose content has been generated by AI. The reason this duality with AI, because it can be an attack and a mechanism for defense, calls for the implementation of strong defense mechanisms against AI-powered attacks along with traditional cyberattacks.
Recommended Steps for AI and ML Implementation in Cybersecurity
While AI and ML will go a long way in strengthening an organization's security posture, proper implementation holds the key to derive maximum benefit out of each. Here is a look at some of the best practices when including AI and ML into your cybersecurity strategy.
Building an AI-Driven Security Strategy
AI has to be integrated into one's security infrastructure very thoughtfully. First, identify the most value-added areas that would best be served by AI/ML, such as threat detection, incident response, or network monitoring. It is also important to make sure that the AI systems will be compatible with the existing security tools and processes.
Another critical factor is data. Artificially intelligent cybersecurity solutions require huge amounts of data for training the machine learning models. Access to diverse, high-quality data that is up-to-date is essential in making effective AI.
Human and AI Collaboration
While AI is powerful in automation, in cybersecurity, human expertise cannot be replaced. Organizations should strive to create an environment that fosters collaboration between human analysts and the AI system. For example, AI can do mundane tasks such as log analysis and alert filtering, while the human analyst makes high-level decisions about strategy and response.
Equally important will be training your cybersecurity team to work alongside AI. Analysts will need to know how AI systems work, their strengths and limitations, and how to interpret insights from AI. A right mix of humans and AI will allow the accuracy and efficiency of threat detection and response to become very effective.
Continuous Model Training and Adaptation
These threats continue to change with time, and so must the machine learning models. Organizations should continuously update the AI models with new data, including information on emerging threats, to keep them effective. Similarly, training and adaptation of the AI systems regularly will help them cope with new types of attack techniques and vulnerabilities.
Furthermore, it is helpful for an organization to track performance over time, knowing when and where to make improvements to the models. This will be an iterative process to ensure the accuracy and reliability of the AI systems continue, given the ever-evolving threat landscape.
Conclusion
As cyber threats are getting more sophisticated and occurring more often, AI, along with machine learning, has emerged to become an integral part of today's cybersecurity defense mechanisms. Artificial intelligence and machine learning were changing the way organizations defend against cyberattacks through superior threat detection at accelerated rates, automating incident responses, and providing predictive insights into those threats.
These technologies do come with their own challenges, though—data quality, adversarial attacks, and overdependence on automation being some of the issues to be most judiciously handled. Besides, AI is not a replacement for human expertise; rather, it is a strong tool that complements the skills of cybersecurity professionals.
Going forward, integrating AI-powered solutions into human-orchestrated strategies will increasingly be the hallmark of the next phase of cybersecurity. Since AI is continuously evolving, its role to defend against increasingly sophisticated threats will also increase, which makes it a part not to be separated from any type of security strategy.
Subscribe to my newsletter
Read articles from Victor Uzoagba directly inside your inbox. Subscribe to the newsletter, and don't miss out.
Written by

Victor Uzoagba
Victor Uzoagba
I'm a technical writer specializing in cybersecurity, with expertise in crafting in-depth, informative content on topics such as cloud security, threat detection, data privacy, and regulatory compliance. With a passion for simplifying complex security concepts, I help organizations and professionals stay informed about the latest trends, tools, and best practices in the cybersecurity landscape.