Financial Engineering in Retail

Table of contents
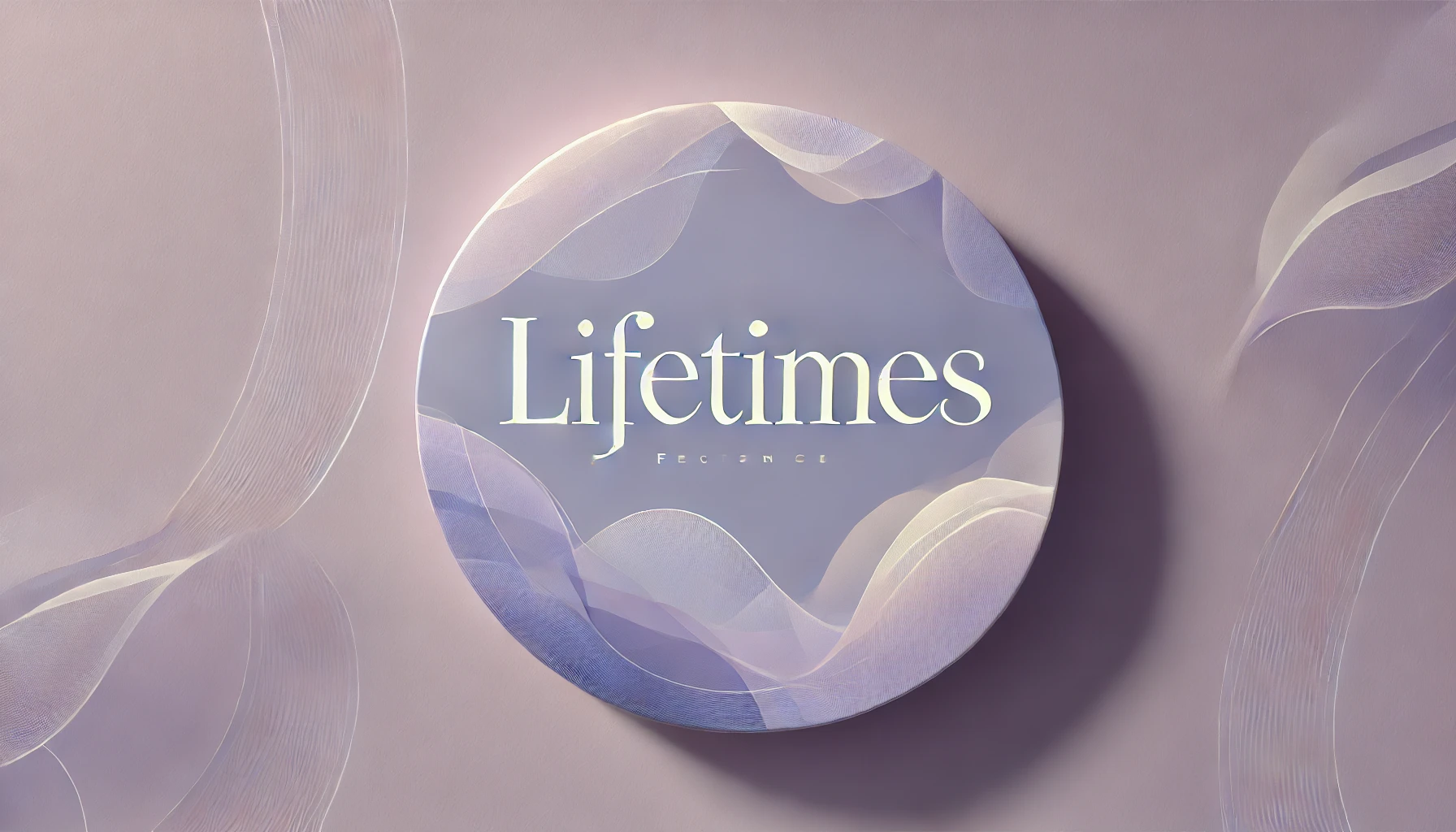
If you’re interested in customer behavior analysis or lifetime value predictions, Lifetimes is an essential tool in your toolkit!
The Lifetimes Python library is used for customer lifetime value (CLV) analysis and related tasks. It’s particularly popular for modeling customer retention and purchase behavior, especially in ecommerce and subscription-based businesses. It helps in understanding how often customers purchase, predicting how likely they are to return, and estimating how long they will remain customers.
Key Features of Lifetimes:
BG/NBD Model: Predicts the number of purchases a customer will make over time.
Gamma-Gamma Model: Estimates the monetary value of each customer.
Pareto/NBD Model: Another model for predicting customer purchase behavior.
Customer Segmentation: Helps segment customers based on their buying patterns.
CLV (Customer Lifetime Value) Prediction: Calculates the lifetime value of customers based on their purchasing behavior.
Use Cases:
Ecommerce: Predict future purchases of customers and identify high-value customers.
Subscription Models: Estimate customer retention and calculate customer lifetime value.
Marketing Campaigns: Use CLV to decide which customer segments to target for marketing efforts.
Advanced Features:
Customer Segmentation: Based on frequency, recency, and monetary value, businesses can create customer segments.
Churn Prediction: Estimate the probability that a customer will stop making purchases.
Revenue Forecasting: Predict future revenue based on customer purchase behavior.
Subscribe to my newsletter
Read articles from Yugal Nandurkar directly inside your inbox. Subscribe to the newsletter, and don't miss out.
Written by
