Why Traditional Data Solutions Fail and How Data Product Approach Can Save the Day
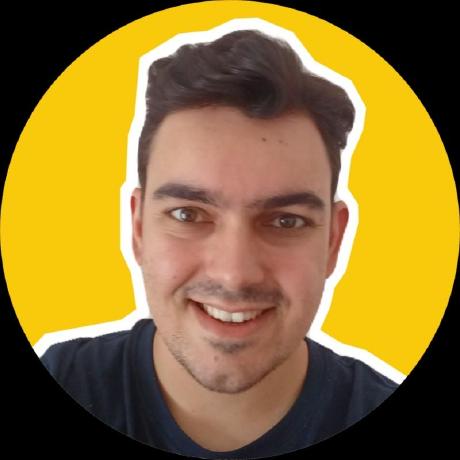
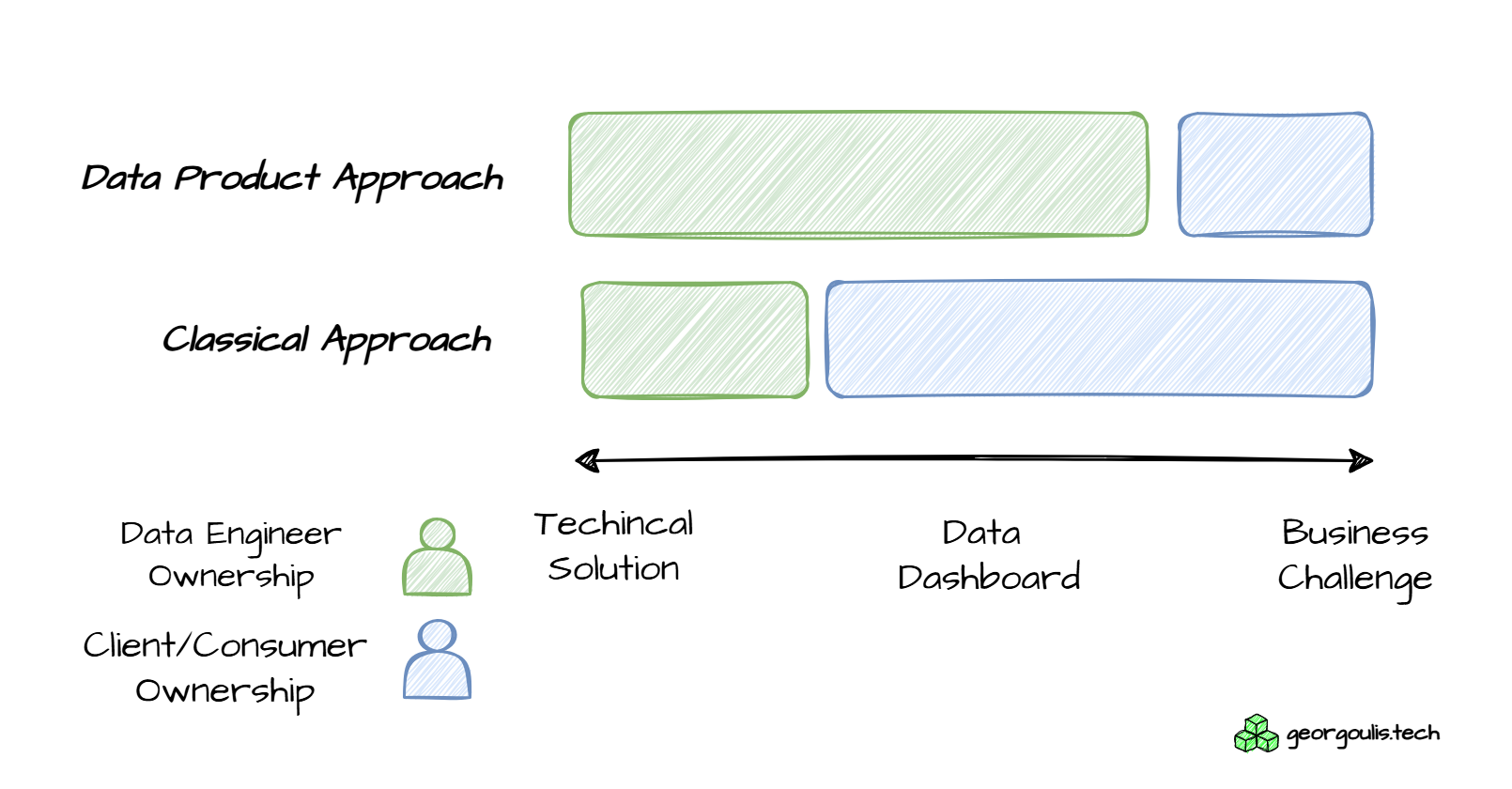
Introduction
I have spoken about it before, but I would like to write a post regarding the importance of building Data Products. Instead of simply delivering platforms, reports, or dashboards, we should focus on building Data Products. A Data Product doesn’t just provide information; it solves a specific business problem and continues to deliver value over time. This shift means the responsibility for identifying and solving the problem moves from the customer to the product development team, requiring a deeper understanding of their needs
The Why
The problem
In many organizations, data teams are tasked with creating dashboards, reports, or tables that, in the end, go largely unused. Despite fulfilling the initial requirements, these deliverables often fail to provide meaningful value to end users. What’s more interesting is that these products are exactly what the customer asked for—so, why do they fail to make an impact?
The core of the problem lies in how we approach building these data apps. Often, we focus on creating individual tools or dashboards to address specific issues that a team faces. Over time, this results in a chaotic sea of reports and dashboards that users must sift through to find the information they need. This overload of data can block teams from making quick decisions and actually increases complexity rather than reducing it.
Why this keeps happening?
So, why this keeps happening is a good question to focus. Several factors contribute to this recurring problem, each of which reflects deeper structural issues in how organizations approach data product development:
1. Tool-Centric Approach
One of the main reasons data products fail is a focus on tools rather than problems. In many cases, each problem is addressed by building a new dashboard or report. As a result, users end up with multiple disconnected tools, each serving a narrow purpose. Instead of streamlining decision-making, this tool-centric approach creates a fragmented data environment where users struggle to find the right information at the right time. The end result? Information overload and slower decision-making.
2. The Self-Service BI Trap
Self-service BI has been touted as the solution to this problem, allowing users to access the data they need without relying on the data team. However, self-service BI often shifts the responsibility for solving data problems onto the users themselves. Rather than focusing on user-specific pain points, this approach emphasizes providing access to raw data. While data access is important, this model often leads to a lack of clear processes, no standardization, and more work for users as they have to figure out how to derive insights on their own.
To be clear, full access to data is valuable, but it should be reserved for research and exploration, not as part of the organization’s standard processes. Without standardized solutions, it becomes difficult to evaluate performance, automate processes, and truly save work hours.
3. Lack of User Involvement
Another common issue is that data product requirements are often gathered from indirect stakeholders—like managers or directors—without involving the actual end users. This results in dashboards and reports that users are forced to interact with but don’t enjoy or find helpful. When users aren’t given the opportunity to provide input during the development process, the final product often misses the mark, creating more frustration than value.
The Result: Data Products That Miss the Mark
When organizations follow this pattern—focusing on tools rather than user needs and relying too heavily on self-service BI, or failing to involve actual users in the development process—they end up with data products that no one truly cares about. These products are cumbersome, difficult to use, and ultimately ineffective in helping teams make better decisions.
Why the Transition to Data Products is a Game-Changer
Having looked at the challenges of traditional data solutions, let's dive into why transitioning to a Data Product mindset offers a far better approach. This shift brings tangible benefits that enhance user experience, increase the effectiveness of data solutions, and streamline operations. Here’s why:
1. Make Your Customer’s Life Easier
At the core of any successful data product is the idea of making the user's life easier. A Data Product is not just about delivering information; it’s about delivering actionable insights that solve real problems.
When data products are well-designed, they integrate seamlessly into the customer’s workflow. Instead of providing a sea of dashboards that users must navigate, a good data product identifies what’s important, offers clear recommendations, and ultimately helps users make faster, more informed decisions. This eliminates the “dashboard fatigue” that users often experience when they are overwhelmed with too many tools that provide little guidance on what to do next.
Example: Rather than creating ten separate dashboards for different reports, a data product could unify key metrics and use intelligent alerts to notify the user when a critical decision is needed. This proactive approach makes their job easier and increases their reliance on the data product.
2. Customers Are Usually Not Data Experts
Many teams and organizations mistakenly assume that their end users—whether they are managers, analysts, or front-line employees—are equipped to make sense of complex data. The truth is, most customers are not data experts, nor should they need to be. The role of a data product is to simplify the complexity and provide insights in an easy-to-understand format.
The typical user is not looking to interpret data, but rather to understand what the data is telling them and how they can act on it. By transitioning to a Data Product model, you remove the burden of data interpretation from the user. You focus instead on presenting key insights, offering solutions, and making recommendations that are directly actionable.
Example: Instead of showing a series of complex graphs and tables, a well-designed data product might present a simple narrative: “Sales in the west region are down by 10% this month.” This clarity drives action without requiring deep technical knowledge, but clear domain context which end users already have.
3. Concentrate Ownership (Domain + Technical)
In traditional approaches, ownership of data solutions is often scattered across various departments—data teams provide the tools, but business teams are expected to use them effectively. This split responsibility often leads to misunderstandings, misaligned expectations, and ultimately, unused products.
In contrast, a Data Product approach centralizes ownership within a dedicated team that understands both the domain expertise (business problems) and the technical requirements (data solutions). This team takes full responsibility for ensuring that the data product is not just technically sound but also valuable to the business.
This concentration of ownership streamlines communication, reduces misalignment, and ensures that the team is fully invested in delivering a solution that works for the end user.
Example: In a Data Product framework, a cross-functional team—comprising data engineers, data scientists, and domain experts—works together from the start to identify the problem and craft a product that solves it. This team owns the entire process, from problem discovery to solution delivery, ensuring that the end product is both relevant and technically sound.
4. Foster Continuous Improvement
A Data Product approach encourages iterative development, allowing for regular updates based on user feedback and evolving business requirements. This not only ensures long-term relevance and adaptability but also helps avoid the need to build entirely new tools or dashboards when requirements change. Instead, you continuously refine the existing product, making it more efficient and valuable as the organization grows.
The Bottom Line: A Better Approach for All
Transitioning to a Data Product approach shifts the focus from merely fulfilling customer requests to proactively solving their problems. You make users’ lives easier by reducing complexity, you simplify insights for non-data experts, and you ensure success by concentrating ownership in a dedicated team.
Ultimately, this approach creates data products that continue to provide meaningful value over time. So, the question is: How will you start building Data Products that matter? [to be continued …]
Subscribe to my newsletter
Read articles from Christos Georgoulis directly inside your inbox. Subscribe to the newsletter, and don't miss out.
Written by
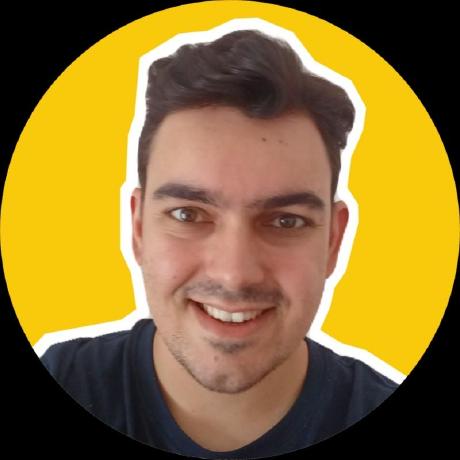