Understanding Data Bias: Types and Solutions for Fairer Analytics
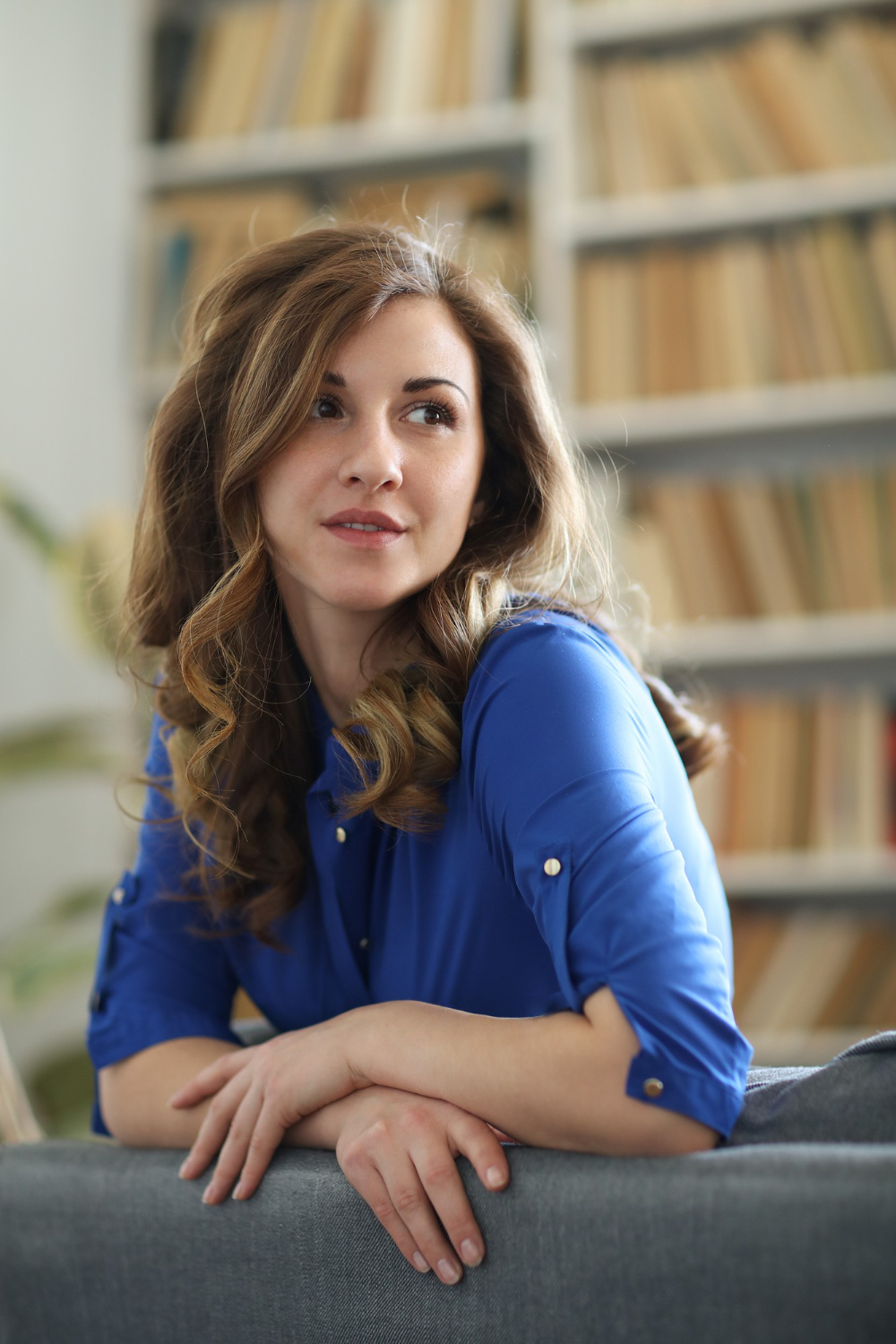
Data is a powerful asset in today’s digital landscape, driving business insights across industries. However, responsibly handling data is essential, as bias in data models can distort decisions and impact operations.
With companies heavily investing in AI, data ethics and bias have become critical challenges in ensuring fair and accurate outcomes. Bias can emerge at any stage of the data process — from defining questions to sampling and organizing datasets.
Addressing these biases is key to unlocking the true value of data, enabling businesses to make reliable, unbiased decisions that fuel growth and innovation.
What is Data Bias?
Human brains and senses have a natural trait to comprehend the surroundings and experiences instinctively seeking patterns in data. Like the human brain, AI/ML models rely on neural networks that comprehend the data they are trained with to analyze and deliver the outcomes. In simple words, data bias is a phenomenon occurring within AI/ML algorithms providing inaccurate or prejudiced results due to mistaken assumptions in the modeling process. This kind of data bias leads to incorrect, unfair, and discriminatory outcomes out of the data.
Different Types Of Data Biases and Their Solutions
Data bias can addressed by treating data ethically and practicing fair approaches to maintain the privacy and accuracy of the data. Having a good data set and treating it with an unbiased perspective is crucial for accurate outcomes. The AI/ML models can have different biases such as race, gender, replication, preference, and others. There are many ways to solve data bias challenges like model auditing and implementation of bias detection tools to make the data more accurate and reliable.
Here are common data biases that could happen during data processes:
1) Confirmation Bias
It happens when preference is given to information that aligns with the existing beliefs or opinions, often without realizing it. This bias leads to emphasizing data that supports the personal viewpoint, influencing the way data is gathered and analyzed unconsciously reinforcing the hypothesis.
How confirmation bias can be avoided:
To mitigate confirmation bias, start by clearly defining your research question, hypothesis, and the objectives of your data analysis before collecting any data. Actively challenge the data you’re working with by seeking evidence that contradicts your assumptions. Once the analysis is complete, carefully compare the results with your initial hypothesis to ensure an objective assessment.
2) Historical Bias
Historical bias arises when past cultural norms, prejudices, or societal beliefs shape the data that was collected, and it can continue to influence present-day data. This bias often reflects ingrained human biases, discrimination, or outdated beliefs, and it can hinder the development of accurate machine learning models by feeding them biased information.
How historical bias can be avoided:
Regularly audit your data sources to identify and correct for historical biases ensuring that underrepresented groups are considered and included in data frameworks. To prevent inaccuracy in future analysis recognize and address the bias in both historical and contemporary datasets.
3) Selection Bias
Selection bias occurs when the data sample does not properly represent the target population, leading to skewed insights. This error often arises from poor study design, such as selecting a non-random or too-small sample.
There are three common types of selection bias:
Sampling bias: When the data is not gathered randomly.
Convergence bias: When data is collected in a way that doesn’t accurately represent the population.
Participation bias: When participants self-select into groups, which distorts the results.
Subscribe to my newsletter
Read articles from Sarah R. Weiss directly inside your inbox. Subscribe to the newsletter, and don't miss out.
Written by
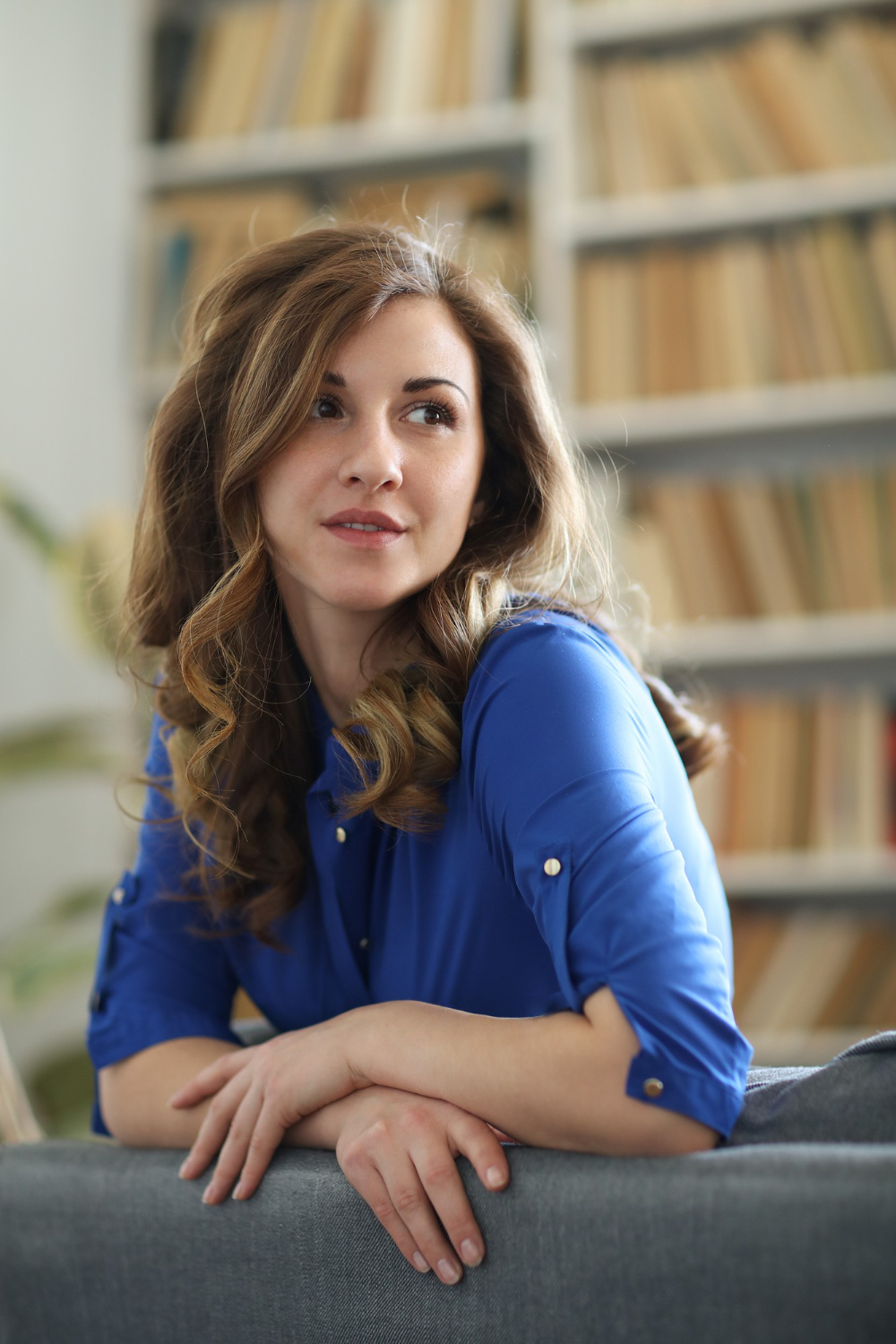
Sarah R. Weiss
Sarah R. Weiss
I share insights on Software Development, Data Science, and Machine Learning services. Let's explore technology together!