Introduction to Decision Science
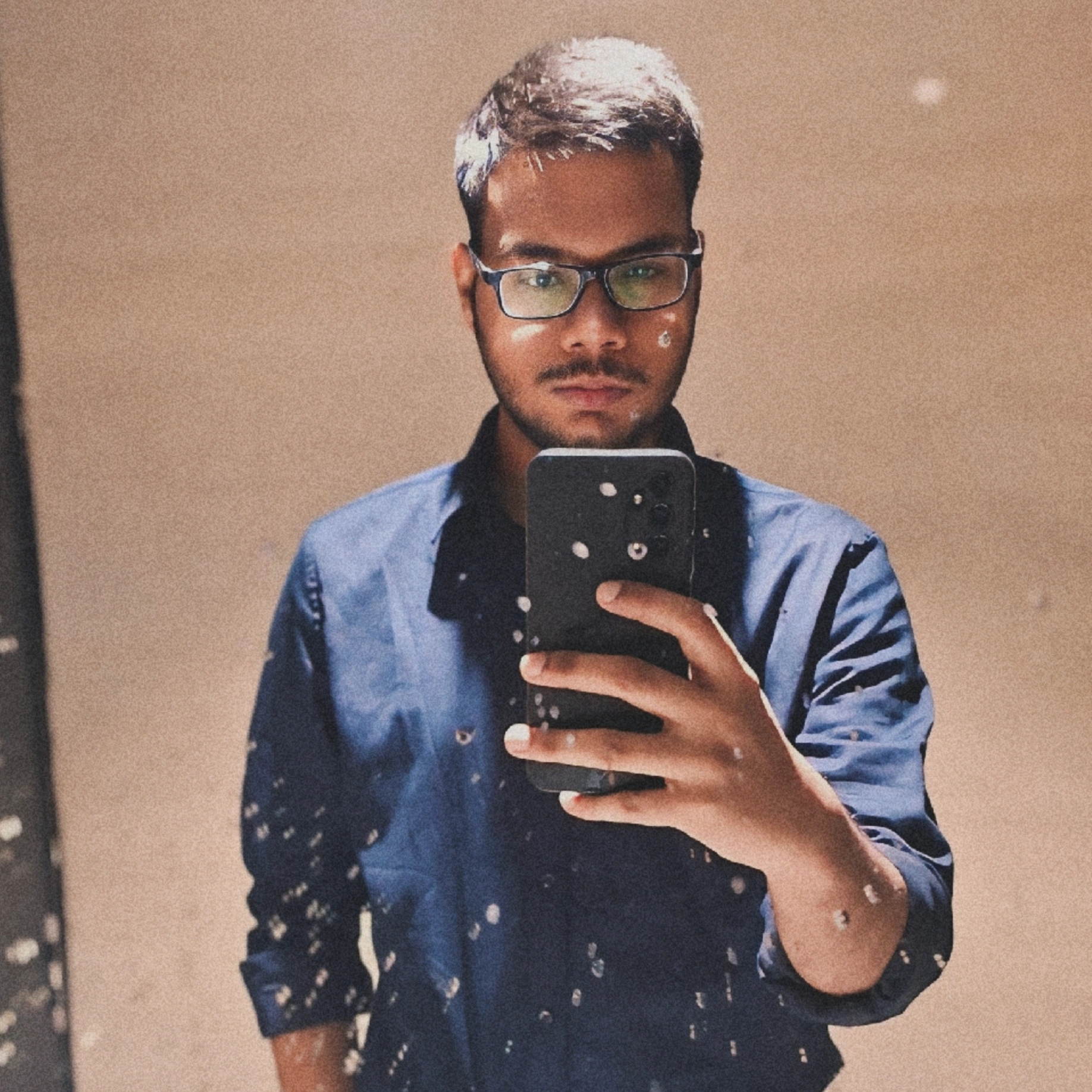
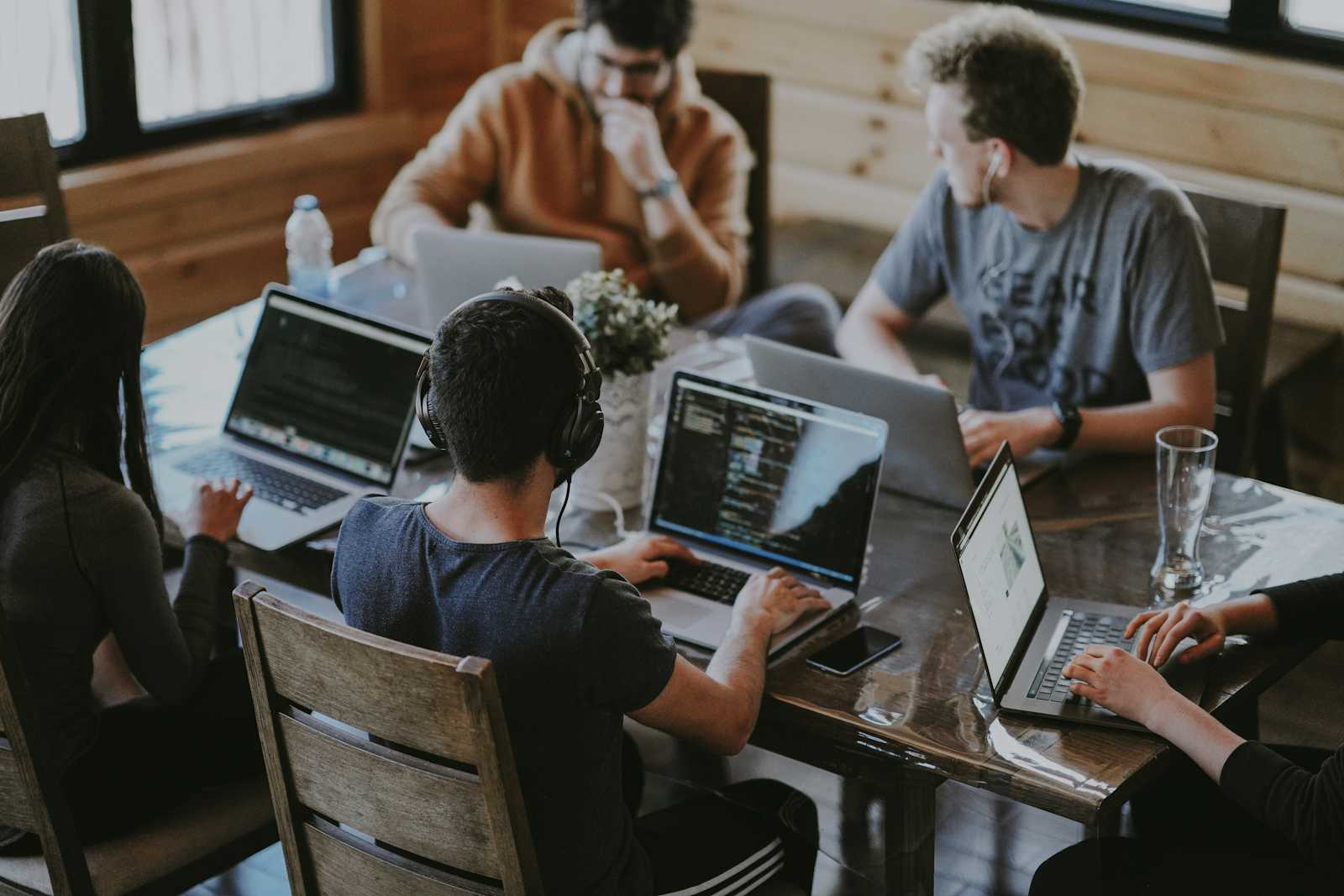
Decision Science is an interdisciplinary field focused on utilizing data, quantitative models, and scientific principles to make well-informed decisions that address complex challenges across various domains. In today's highly interconnected world, where organizations face diverse and multifaceted challenges, the demand for robust decision-making frameworks has surged. Decision Science meets this need by drawing insights from fields like statistics, mathematics, data science, psychology, and computer science. At its core, Decision Science empowers organizations and individuals to analyze alternatives, evaluate potential outcomes, and make decisions based on empirical evidence, thus enhancing the likelihood of achieving optimal results. The methodology behind Decision Science involves systematically examining all available options, evaluating the associated risks and rewards, and determining the most effective course of action to accomplish the intended objective.
A key element of Decision Science lies in its reliance on data. In our information-driven world, data has become an invaluable asset, offering insights into consumer behavior, market trends, operational efficiencies, and more. Decision scientists utilize sophisticated tools and techniques to process and analyze massive datasets, identifying patterns, correlations, and causal relationships that are often invisible to the naked eye. With these insights, decision scientists can help organizations better understand their current circumstances, forecast future trends, and make proactive decisions. Machine learning and artificial intelligence (AI) are two pivotal technologies within Decision Science, enabling the creation of predictive models that learn from historical data to improve their accuracy over time. These predictive models are indispensable in industries like finance, healthcare, retail, and supply chain management, where anticipating future trends can mean the difference between success and failure.
Beyond the computational aspects, Decision Science also incorporates a deep understanding of human behavior. Behavioral Decision Science, a subfield, studies how individuals and groups make decisions, often influenced by biases, heuristics, and psychological factors. For instance, cognitive biases—such as confirmation bias, where individuals favor information that confirms their preexisting beliefs—can distort the decision-making process, leading to suboptimal outcomes. Decision scientists aim to identify and mitigate these biases by introducing evidence-based methods and objective analysis into the decision-making process. This approach acknowledges that while human intuition and judgment are valuable, they are also fallible. By incorporating psychological insights, Decision Science seeks to refine decision-making frameworks that balance human intuition with empirical rigor, resulting in choices that are both logical and aligned with real-world constraints.
In business, Decision Science plays an essential role in shaping strategies, optimizing operations, and enhancing customer experiences. From small startups to multinational corporations, companies face a myriad of decisions that can significantly impact their success. Decision scientists apply their expertise in data analysis, modeling, and optimization to support strategic planning, market entry decisions, resource allocation, and risk management. For example, in the context of marketing, Decision Science enables companies to segment customers based on purchasing behavior, preferences, and demographics. This segmentation allows businesses to create targeted marketing campaigns that resonate with specific audiences, thus maximizing their marketing ROI. Additionally, decision scientists can help organizations optimize pricing strategies, ensuring that prices are competitive while still generating profit. In the age of e-commerce, where companies have access to vast amounts of customer data, Decision Science is instrumental in creating personalized recommendations and enhancing user engagement, ultimately leading to increased sales and customer satisfaction.
Another area where Decision Science has made a significant impact is in the financial industry. Investment firms, banks, and insurance companies rely heavily on data to make informed decisions about asset allocation, risk assessment, and portfolio management. Decision Science facilitates the creation of complex financial models that can predict market movements, evaluate credit risk, and optimize investment strategies. In algorithmic trading, for instance, decision scientists develop algorithms that analyze real-time data to identify profitable trading opportunities. These algorithms can execute trades within milliseconds, capitalizing on market fluctuations before human traders have a chance to react. Decision Science also plays a crucial role in risk management, as financial institutions must evaluate the potential risks associated with loans, investments, and other financial transactions. By utilizing probabilistic models, decision scientists can estimate the likelihood of various scenarios, enabling firms to make risk-adjusted decisions that protect their assets while still pursuing growth opportunities.
Healthcare is yet another domain where Decision Science has transformed the landscape, particularly in the areas of diagnostics, treatment planning, and healthcare management. With the advent of electronic health records, medical institutions now have access to vast amounts of patient data, including medical history, genetic information, and lifestyle factors. Decision scientists work alongside healthcare professionals to analyze this data, identifying patterns that can improve diagnostic accuracy and personalize treatment plans. For example, predictive models can assess a patient's risk of developing certain conditions, allowing for early interventions that could prevent or mitigate the disease. In hospitals, Decision Science helps optimize resource allocation by forecasting patient demand, ensuring that medical staff and resources are available when and where they are needed most. Additionally, in the realm of public health, decision scientists analyze epidemiological data to track the spread of diseases, assess the effectiveness of interventions, and inform policy decisions aimed at improving population health outcomes.
Supply chain and logistics is another sector where Decision Science has proven invaluable, particularly in the context of globalized trade and complex distribution networks. Companies face constant pressure to deliver goods efficiently while minimizing costs, and decision scientists use advanced models to optimize supply chain operations. Predictive analytics plays a crucial role in demand forecasting, allowing companies to anticipate customer demand and adjust inventory levels accordingly. This proactive approach helps prevent stockouts and overstock situations, both of which can be costly for businesses. Furthermore, Decision Science supports route optimization, ensuring that goods are transported along the most efficient paths to reduce delivery times and fuel costs. By analyzing historical data on factors such as shipping routes, traffic patterns, and weather conditions, decision scientists can create models that improve delivery accuracy and customer satisfaction. In times of crisis, such as natural disasters or global pandemics, Decision Science becomes even more critical, as companies must adapt quickly to disruptions in supply chains. With the right decision-making frameworks, organizations can respond effectively to these challenges, maintaining resilience in the face of uncertainty.
The intersection of technology and Decision Science has given rise to intelligent systems capable of making autonomous decisions. Autonomous vehicles, for example, rely on a combination of Decision Science and AI to navigate complex environments, respond to obstacles, and make split-second decisions that ensure passenger safety. These systems use data from sensors, cameras, and GPS to build a real-time understanding of their surroundings, enabling them to make decisions based on a constantly evolving set of inputs. Similarly, smart cities utilize Decision Science to manage resources such as energy, water, and transportation systems. By analyzing data from various sensors and IoT devices, city planners can optimize traffic flow, reduce energy consumption, and improve waste management, all of which contribute to a more sustainable urban environment. However, as autonomous systems become more prevalent, ethical considerations become increasingly important. Decision scientists must ensure that these systems make fair and transparent decisions, particularly in situations where human lives are at stake. Issues such as bias in AI algorithms, transparency, and accountability are critical areas of concern that must be addressed to build public trust in these technologies.
Despite its many advantages, Decision Science faces several challenges, particularly in the areas of data privacy and security. As decision scientists gather and analyze large datasets, they must adhere to strict data protection regulations to ensure that individuals' privacy is respected. This is especially relevant in sectors like healthcare and finance, where sensitive information is often involved. Additionally, the risk of data breaches and cyberattacks poses a significant threat, as unauthorized access to data could lead to misuse or exploitation. Decision scientists must work closely with cybersecurity experts to implement robust security measures that protect data integrity and confidentiality. Another challenge is the potential for bias in decision-making models, particularly when the data used to train these models is itself biased. For instance, if a hiring algorithm is trained on historical data that reflects past discriminatory practices, it may inadvertently perpetuate these biases. Decision scientists must be vigilant in identifying and mitigating bias to ensure that their models are fair and inclusive.
Transparency and interpretability are also crucial considerations in Decision Science, particularly when models are used to make decisions that impact people's lives. Complex models, such as deep neural networks, often operate as "black boxes," meaning that their decision-making processes are not easily understood by humans. This lack of transparency can be problematic, especially in high-stakes domains like healthcare and criminal justice, where stakeholders need to understand the rationale behind certain decisions. To address this issue, decision scientists are exploring methods to make models more interpretable, such as feature importance analysis and surrogate modeling. By enhancing transparency, decision scientists can build trust in their models and ensure that decisions are made in an ethical and accountable manner.
Looking to the future, Decision Science is poised to become even more integral to the decision-making processes of organizations and individuals alike. Advances in AI, machine learning, and quantum computing hold the potential to revolutionize Decision Science, enabling more accurate and efficient models that can tackle increasingly complex problems. For example, quantum computing could enable decision scientists to solve optimization problems that are currently infeasible with classical computers, opening up new possibilities in fields like logistics, finance, and materials science. Furthermore, as Decision Science continues to evolve, it will likely play a central role in addressing global challenges such as climate change, poverty, and public health. By harnessing the power of data and advanced modeling techniques, decision scientists can develop solutions that are both innovative and impactful, contributing to a more sustainable and equitable world.
In conclusion, Decision Science is a dynamic and multifaceted field that combines the strengths of various disciplines to improve decision-making in an increasingly complex world. By leveraging data, quantitative models, and insights into human behavior, decision scientists empower organizations to make informed choices that drive success. From business and finance to healthcare and public policy, the applications of Decision Science are vast and varied, underscoring its importance in modern society. As technology continues to advance, the role of Decision Science will only grow, providing new opportunities to solve pressing problems and improve the quality of life for people around the world. However, as we embrace the benefits of Decision Science, we must also remain mindful of the ethical and practical challenges it presents,
striving to create decision-making frameworks that are fair, transparent, and respectful of individual rights. In doing so, Decision Science can truly fulfill its potential as a force for positive change in the world.
Subscribe to my newsletter
Read articles from Anshuman Sinha directly inside your inbox. Subscribe to the newsletter, and don't miss out.
Written by
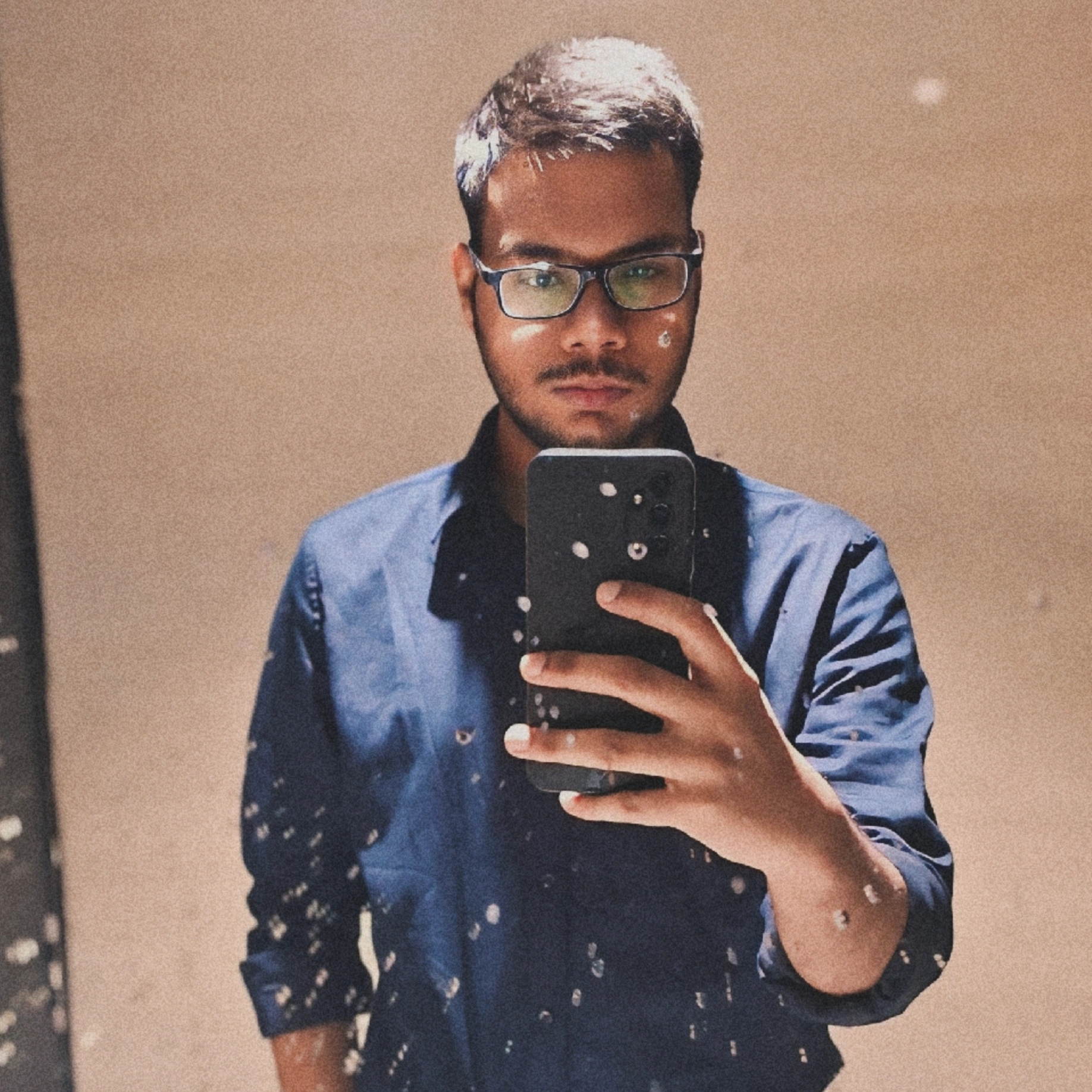
Anshuman Sinha
Anshuman Sinha
Software Developer who previously worked as an SDE Intern at a consulting firm and as a Data Science intern at an IT Firm. Currently pursuing BCA from Amity University Patna.