The Ethical Implications of Machine Learning

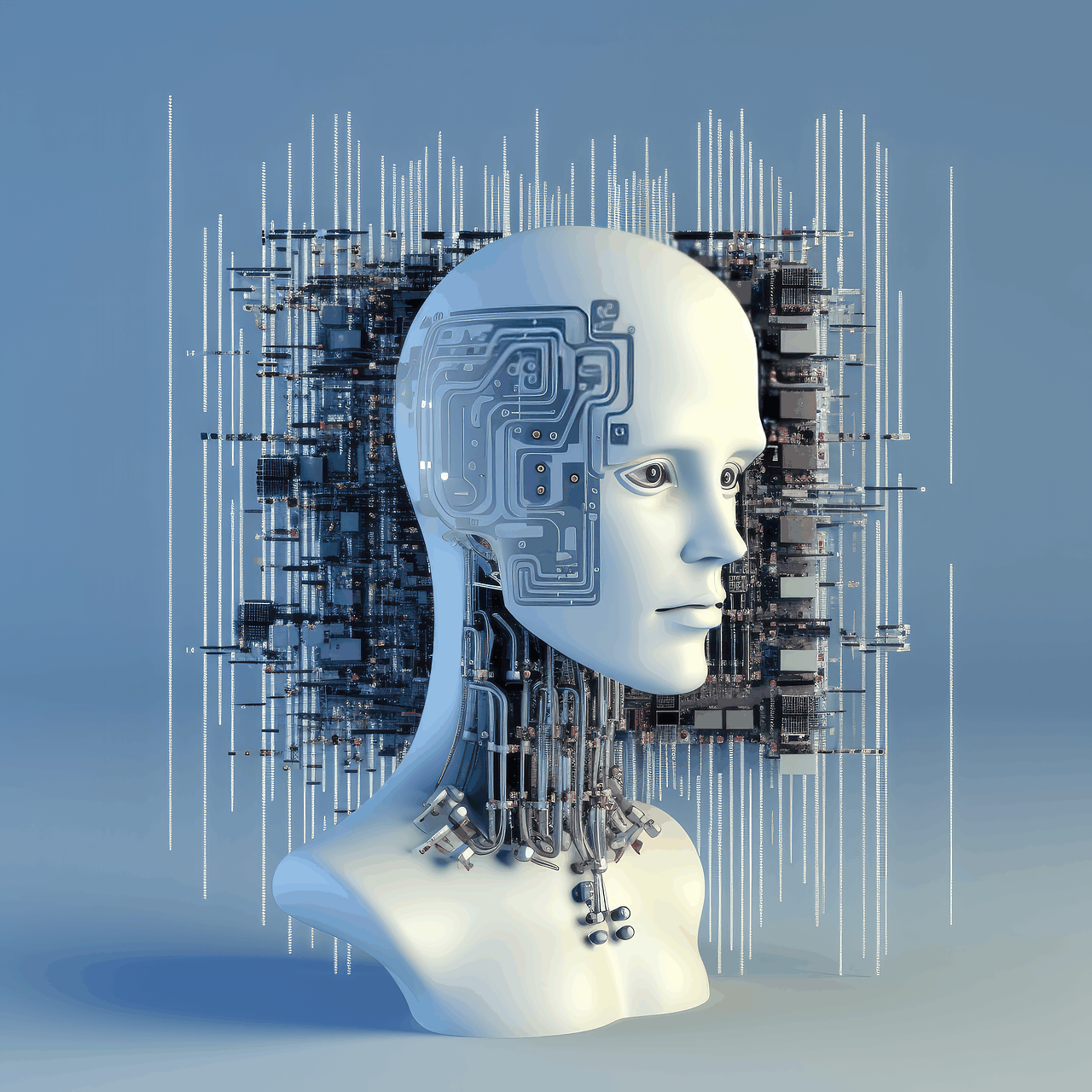
Introduction
Machine learning (ML) has ushered in a new era of technological advancement, influencing countless aspects of daily life. From self-driving cars to personalized online shopping experiences, the power of machine learning lies in its ability to analyze vast datasets, identify patterns, and make predictions. However, as we integrate machine learning into more areas of our lives, it raises critical ethical questions that we must confront.
The ethical implications of machine learning are multifaceted and complex. Issues surrounding bias in AI, fairness in algorithms, accountability for decisions made by machines, and the need for transparency in how these systems operate are paramount. These concerns are not merely theoretical; they manifest in real-world consequences affecting individuals and communities. For instance, biased algorithms can lead to discriminatory practices in hiring, lending, and law enforcement.
As machine learning continues to evolve, understanding its ethical implications becomes increasingly crucial. This article will explore these key themes, providing a comprehensive overview of the ethical landscape in machine learning. We'll discuss how bias creeps into AI, the importance of fairness, mechanisms for accountability, and strategies for ensuring transparency. By navigating these ethical waters, we can ensure that machine learning technologies are developed and implemented responsibly, ultimately benefiting society as a whole.
Understanding Bias in AI
What is Bias in AI?
Bias in AI refers to the tendency of algorithms to produce skewed or unfair outcomes due to underlying prejudices present in the training data or model design. When we think of AI bias, it’s essential to understand that algorithms do not possess opinions or feelings. Instead, they reflect the data they're trained on, which can often include historical biases. This means that if a model is trained on biased data, it will likely replicate those biases in its predictions and decisions.
For example, if a facial recognition system is predominantly trained on images of lighter-skinned individuals, it may struggle to accurately identify darker-skinned faces. This can lead to significant consequences, such as wrongful accusations or the denial of services. Understanding and addressing bias in AI is critical to ensuring fair and equitable outcomes for all users.
Sources of Bias in Machine Learning
Several key sources of bias can influence machine learning models:
Data Selection: The data used to train algorithms is often not representative of the population. If certain groups are underrepresented, the model may perform poorly for those groups. For instance, a hiring algorithm trained on resumes from a predominantly male workforce may undervalue resumes from female candidates.
Feature Selection: The choice of features included in a model can introduce bias. If features that correlate with sensitive attributes (like race or gender) are included, they may lead to biased outcomes.
Algorithmic Design: Some algorithms may inherently favor specific outcomes based on their structure or decision-making process. For example, a decision tree that splits data based on specific thresholds might inadvertently favor one demographic over another.
Historical Bias: If historical data reflects societal biases, the algorithm trained on that data will likely perpetuate those biases. This is particularly concerning in areas like criminal justice, where biased data can lead to discriminatory sentencing.
Real-World Examples of Bias in AI
Real-world examples of bias in AI are numerous and concerning. One notable case involved a machine learning tool used by law enforcement to predict criminal activity. An investigation revealed that the algorithm disproportionately flagged Black individuals as potential criminals, leading to unjust targeting by police.
Another example comes from a hiring algorithm developed by a major tech company. The system was found to favor male candidates over equally qualified female candidates due to biases present in the training data, which was sourced from previous hiring decisions that favored men. These examples illustrate the real and harmful impacts of biased algorithms, emphasizing the need for vigilance and proactive measures in developing AI systems.
Fairness in Machine Learning
Defining Fairness in AI
Fairness in AI is a complex concept that involves ensuring equitable treatment and outcomes for all individuals affected by automated systems. It means that decisions made by AI should not be influenced by bias related to race, gender, socio-economic status, or other protected characteristics. Fairness is not a one-size-fits-all principle; it varies based on context and stakeholder perspectives.
Achieving fairness requires careful consideration of various factors, including the values of the society in which the AI is deployed. What is considered fair in one cultural context may not be viewed the same way in another. Therefore, defining fairness in AI is an ongoing dialogue involving developers, ethicists, and the communities affected by these technologies.
Different Types of Fairness
There are several frameworks for understanding fairness in AI, including:
Individual Fairness: This principle posits that similar individuals should receive similar treatment from algorithms. For example, if two job applicants have the same qualifications, they should have an equal chance of being hired, regardless of their backgrounds.
Group Fairness: This approach focuses on ensuring that outcomes are equitable across different demographic groups. For instance, an algorithm used for loan approval should aim for similar approval rates among various racial or gender groups.
Subgroup Fairness: This concept goes a step further by ensuring that specific subgroups (e.g., minority groups) receive equitable treatment within broader categories. For example, if a health insurance algorithm evaluates applicants, it should ensure that women of color are not systematically disadvantaged compared to their peers.
Counterfactual Fairness: This approach evaluates whether an algorithm's decision would change if a sensitive attribute (like race or gender) were altered. If changing this attribute would lead to a different outcome, the algorithm may be considered biased.
Measuring Fairness in Algorithms
Measuring fairness in algorithms involves applying specific metrics to assess their outcomes. Some common metrics include:
Demographic Parity: This metric assesses whether the acceptance rates of different demographic groups are equal. For instance, if 80% of white applicants are accepted, then ideally, 80% of Black applicants should be accepted as well.
Equal Opportunity: This metric evaluates whether true positive rates (the percentage of actual positives correctly identified by the model) are similar across groups. For example, if 70% of qualified applicants are identified as suitable by the algorithm for one group, the same should hold for another group.
By applying these metrics, developers can better understand how their models perform across different demographics, helping them identify and address any potential biases.
Accountability in AI Systems
Importance of Accountability
As machine learning systems become more autonomous, the question of accountability becomes increasingly important. When an algorithm makes a decision that negatively impacts an individual or a group, who is responsible? Is it the data scientists who created the model, the organization deploying it, or the AI itself? Establishing clear lines of accountability is essential to ensure that individuals or organizations can be held responsible for the consequences of AI decisions.
Accountability is crucial not just for ethical considerations but also for fostering trust in AI systems. When people know that there are mechanisms in place to hold entities accountable for their AI's decisions, they are more likely to trust and engage with these technologies. Therefore, fostering a culture of accountability within organizations that develop AI systems is key to their successful implementation.
Who is Responsible for AI Decisions?
Determining who is responsible for the outcomes produced by AI systems can be complex. Several parties may share accountability, including:
Developers and Data Scientists: These individuals are responsible for designing algorithms and selecting data. They should ensure that their models are fair and unbiased.
Organizations: Companies deploying AI systems must establish governance frameworks to oversee the ethical implications of their technologies. They should implement checks and balances to ensure responsible use.
Policy Makers: Governments play a crucial role in establishing regulations and standards for AI development and deployment. They must create legal frameworks that hold organizations accountable for the impacts of their AI systems.
Users: In some cases, users of AI systems may also bear responsibility, especially if they misuse or misinterpret the outputs produced by algorithms.
Accountability Mechanisms in Machine Learning
Implementing effective accountability mechanisms in machine learning is vital to ensure ethical practices. Some strategies include:
Regular Audits: Conducting periodic reviews of AI systems to assess their fairness, transparency, and overall performance. Audits can help identify potential biases and mitigate their impacts.
Documentation: Maintaining thorough records of the algorithm's development process, including data sources, feature selection, and decision-making criteria. Documentation helps track the origins of bias and provides insights into how to improve the system.
Ethics Boards: Organizations can establish ethics committees to oversee AI initiatives and ensure adherence to ethical standards. These boards can provide guidance and recommendations on ethical dilemmas related to AI deployment.
By integrating these accountability mechanisms, organizations can promote ethical AI practices and build trust with users and stakeholders.
Transparency in AI Models
What is Transparency in AI?
Transparency in AI refers to the extent to which the workings of an AI system are made clear and understandable to stakeholders. It encompasses both the data used for training and the decision-making processes of algorithms. In a transparent AI system, users should be able to understand how decisions are made and what factors influence those decisions.
Transparency is critical for fostering trust among users and stakeholders. When individuals understand how an AI system operates, they are more likely to accept its decisions. Additionally, transparency aids in identifying and addressing biases, allowing for more ethical deployment of AI technologies.
Benefits of Transparency in Machine Learning Words
Transparency offers several key benefits in the context of machine learning:
Enhanced Trust: When users can see how decisions are made, they are more likely to trust AI systems. This trust is crucial for encouraging the adoption and use of these technologies.
Bias Detection: Transparent AI systems enable stakeholders to analyze the data and algorithms for potential biases. By understanding the inputs and decision processes, organizations can address biases and improve fairness.
Accountability: Transparency promotes accountability by making it easier to trace decisions back to specific data and algorithms. If an algorithm produces biased results, it is easier to identify the source of the problem and rectify it.
Informed Decision-Making: Transparency allows users to make informed choices based on a clear understanding of how an AI system operates. This is particularly important in high-stakes scenarios, such as healthcare or criminal justice, where decisions can significantly impact individuals' lives.
Achieving Transparency in AI Systems
To achieve transparency in AI systems, several strategies can be employed:
Explainable AI (XAI): Developing AI models that can provide clear explanations for their decisions. XAI techniques allow users to understand the reasoning behind specific outcomes, making it easier to trust and validate the results.
Open Data Practices: Making training datasets available for public scrutiny can enhance transparency. When datasets are accessible, it allows for independent assessments of potential biases.
User Education: Educating users about how AI systems work can foster transparency. Providing resources and training can help users understand the technology, increasing their comfort and confidence in using it.
By prioritizing transparency, organizations can build trust, promote accountability, and ensure that their AI systems are fair and ethical.
Conclusion
The ethical implications of machine learning are vast and complex, touching on critical issues of bias, fairness, accountability, and transparency. As machine learning technologies become increasingly integrated into our daily lives, it is essential to address these ethical concerns proactively.
Bias in AI can lead to unfair outcomes, perpetuating historical injustices and discrimination. Recognizing the sources of bias and implementing strategies to mitigate it is crucial for the development of fair algorithms. Moreover, establishing accountability frameworks ensures that individuals and organizations can be held responsible for the decisions made by AI systems, fostering trust and ethical practices.
Transparency in AI is equally important, as it allows stakeholders to understand how decisions are made and promote informed decision-making. By prioritizing transparency, organizations can identify biases and build systems that are fair and equitable.
As we continue to advance in the field of machine learning, it is imperative to engage in ongoing discussions about these ethical implications. By working collaboratively, developers, organizations, policymakers, and communities can navigate the challenges of AI ethics and create a future where machine learning technologies serve the best interests of society.
FAQs
1. What are the ethical implications of machine learning?
The ethical implications of machine learning include concerns about bias, fairness, accountability, and transparency. These issues arise from the potential for algorithms to make biased decisions based on historical data, affecting various aspects of life, including hiring, lending, and law enforcement.
2. How can bias in AI be mitigated?
Bias in AI can be mitigated by ensuring diverse and representative training data, applying fairness metrics, conducting regular audits, and employing techniques such as Explainable AI (XAI) to understand decision-making processes.
3. Why is fairness important in machine learning?
Fairness is essential in machine learning because it ensures that individuals are treated equitably and that algorithms do not perpetuate existing inequalities. Fair algorithms contribute to just outcomes and foster trust among users.
4. How can organizations ensure accountability in AI systems?
Organizations can ensure accountability by implementing governance frameworks, conducting regular audits, maintaining thorough documentation of algorithm development, and establishing ethics boards to oversee AI initiatives.
5. What role does transparency play in AI ethics?
Transparency is critical for fostering trust in AI systems, enabling users to understand how decisions are made, and promoting accountability. Transparent systems help identify biases and encourage informed decision-making among users.
Join Weskill’s Newsletter for the latest career tips, industry trends, and skill-boosting insights! Subscribe now:https://weskill.beehiiv.com/
Subscribe to my newsletter
Read articles from Weskill directly inside your inbox. Subscribe to the newsletter, and don't miss out.
Written by

Weskill
Weskill
WeSkill.org is an innovative online learning platform dedicated to empowering individuals through accessible, high-quality education. We provide a wide range of courses covering various fields such as technology, business, health, and personal development, aimed at helping learners acquire new skills and advance their careers. With a focus on practical knowledge and real-world applications, WeSkill.org offers interactive content, expert-led instruction, and community support to ensure that every student achieves their educational goals. Whether you’re a beginner looking to start your journey or a professional seeking to enhance your expertise, WeSkill.org is your partner in lifelong learning.