The Great Opacity: Why AI Labs Need to Come Clean About Their Data

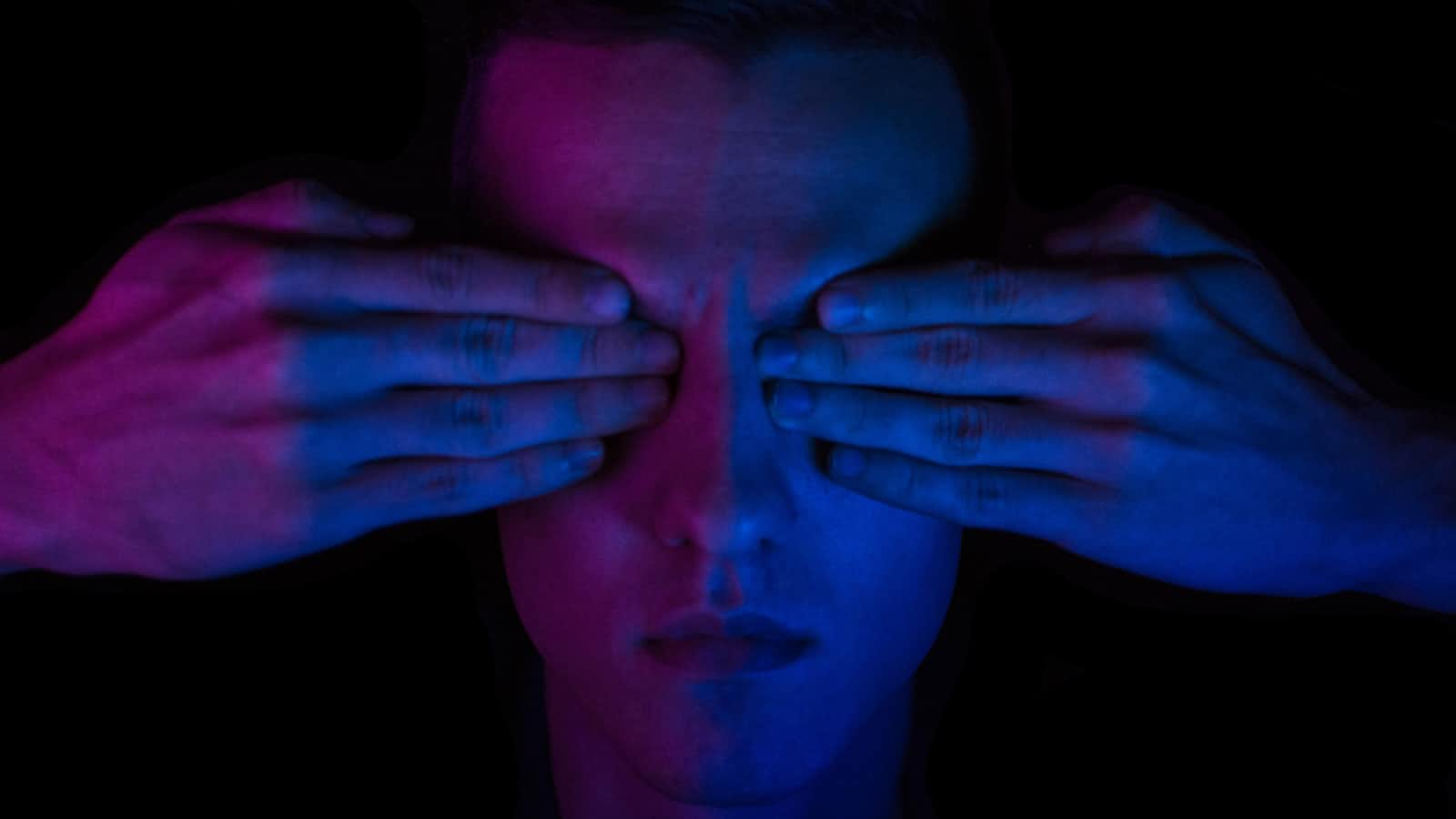
In the world of AI, there's an uncomfortable truth lurking beneath the surface: major AI labs have the power to tell us where their training data comes from, but they're choosing not to. This isn't due to any technical limitation. It's a deliberate choice—one that deserves intense scrutiny.
The Dirty Little Secret of AI
We often think of AI systems as all-knowing and incredibly advanced, but the reality is more mundane. Large Language Models (LLMs) like the ones powering today's AI assistants don't truly "understand" us. They simply process our queries based on patterns in the data they were trained on. Every output they generate is rooted in this data, and when something goes wrong—when the output is biased, misleading, or downright inaccurate—the problem usually traces back to the quality of that data.
But here's the thing: AI labs don't want us to know what data they're using. They claim that disclosing their training data sources is too complex or too costly. Yet, this rings hollow when we consider that search engines have been indexing and tracking billions of sources for decades. It's not that source tracking is impossible; it's that these companies are unwilling to invest in transparency.
What Are They Hiding?
So, why all the secrecy? It's not just about keeping costs down or protecting trade secrets. The reluctance to share training data sources often conceals unsettling truths about how this data is collected:
1. Social Media Harvesting Without Consent
Imagine the data that fuels your favorite AI assistant: personal conversations from Reddit, user interactions on Twitter and Instagram, private Facebook discussions. These sources are often scraped without clear consent, putting user privacy on the line.
2. Copyright Violations Galore
Copyright laws are meant to protect creators, but AI labs aren't always following the rules. Books, articles, academic papers, and other copyrighted materials are frequently swept up in the data sets without permission. This isn't just an oversight; it's a choice to ignore intellectual property rights for the sake of expediency.
3. Personal Data at Risk
It doesn't stop at social media and copyrighted works. These models can also pick up sensitive personal data, from user profiles and demographic information to private discussions about health or finance. The lack of transparency around data use means that people's lives are potentially exposed, with no recourse for those affected.
Who Pays the Price for Opacity?
The consequences of this opacity are far-reaching and should make us all uneasy. Without transparency, AI companies expose themselves (and by extension, all of us) to serious risks:
Legal Vulnerability: There's a ticking time bomb here. From copyright infringement claims to privacy violations, AI labs could face massive legal repercussions as more is uncovered about their data sources.
Ethical Violations: Without the ability to verify data quality, there's no way to assess biases or misinformation in outputs. Users can't check the relevance or accuracy of information, and creators have no way of knowing if their work is being used without permission.
Trust Erosion: How can we trust these systems if we have no visibility into what data they rely on? Without source tracking, users can't assess expertise, identify biases, or verify the credibility of information.
It's Time for Accountability
AI labs often claim they're pushing boundaries for the greater good, but if that were true, they would prioritize transparency over secrecy. If they're serious about building trustworthy AI, here's what they need to do:
Source Attribution
- Start with the basics: track and disclose training data sources. Allow users to see where the information comes from, just as search engines provide URLs for their results.
User Rights
- People should have the right to opt out of having their personal data used in training AI. This includes clear consent mechanisms, data removal options, and transparent policies.
Technical Solutions
- Invest in source-tracking systems and attribution mechanisms. The technology exists; it's just a matter of implementing it.
Industry Standards
- We need clear guidelines on data collection and protection. Regular audits, mandatory source disclosure, and ethical guidelines should be standard in AI development.
A Call to Action
As users and stakeholders, we can't ignore this issue. The AI industry has a responsibility to be transparent about its data practices, and it's on us to hold them accountable. Demand AI that is built on integrity, not secrecy. Demand ethical AI.
The future of AI hinges on transparency. If AI labs continue to cut corners on data quality and user rights, then the ethical concerns around AI will only intensify. This isn't just about technology; it's about building a foundation of trust. Let's make sure the AI of tomorrow is something we can believe in.
Subscribe to my newsletter
Read articles from Gerard Sans directly inside your inbox. Subscribe to the newsletter, and don't miss out.
Written by

Gerard Sans
Gerard Sans
I help developers succeed in Artificial Intelligence and Web3; Former AWS Amplify Developer Advocate. I am very excited about the future of the Web and JavaScript. Always happy Computer Science Engineer and humble Google Developer Expert. I love sharing my knowledge by speaking, training and writing about cool technologies. I love running communities and meetups such as Web3 London, GraphQL London, GraphQL San Francisco, mentoring students and giving back to the community.