Deep Learning and AI Cloud for Image Super Resolution

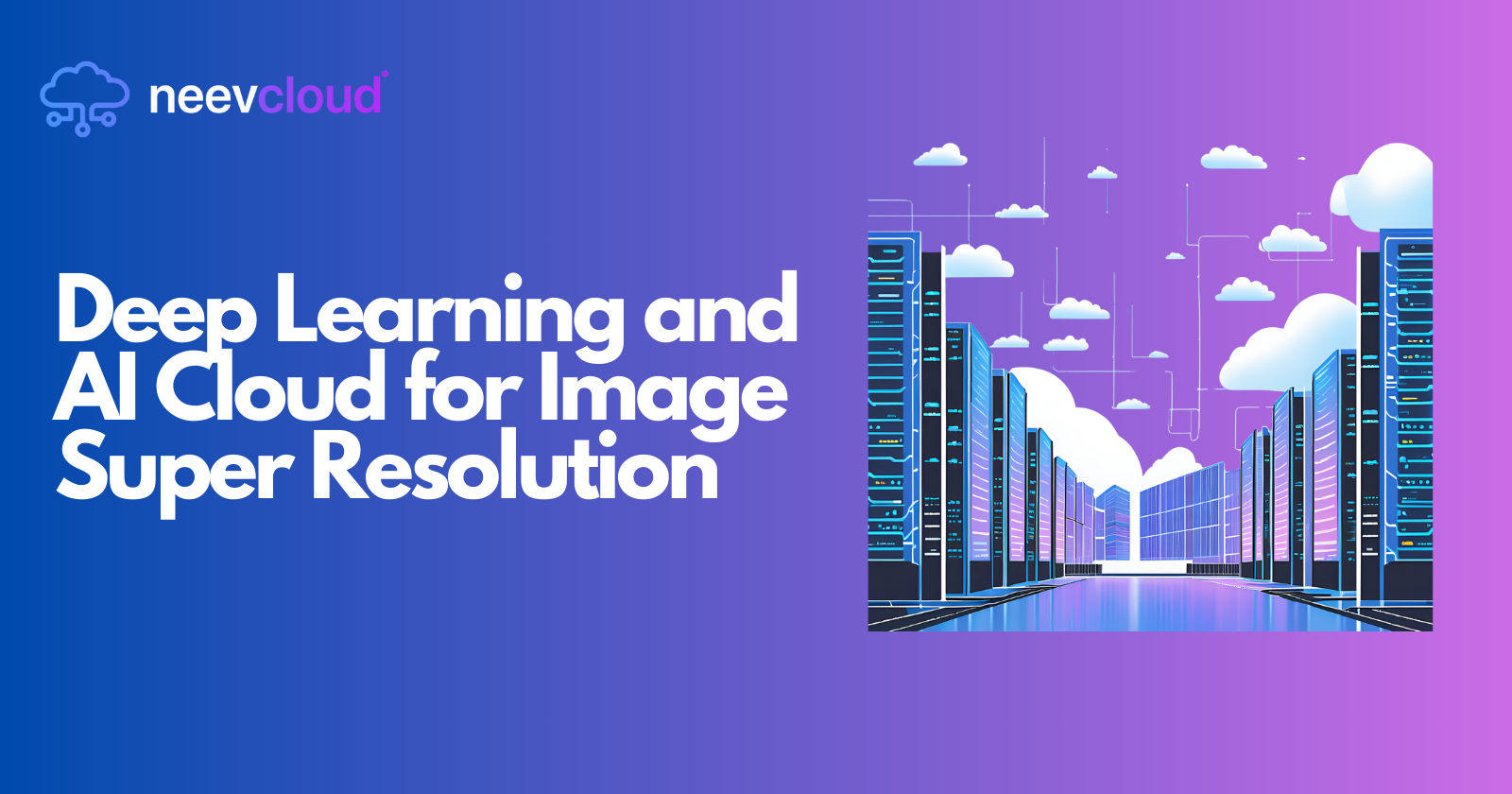
As the demand for high-quality visuals continues to surge across industries, from entertainment to scientific research, so does the need for powerful image enhancement methods. Deep Learning (DL) and Artificial Intelligence (AI) Cloud solutions are unlocking new possibilities in Image Super Resolution (ISR) — the process of reconstructing a high-resolution image from its lower-resolution counterpart. With AI Cloud platforms and specialized AI Datacenters, we now have the ability to leverage robust deep learning networks for efficient and scalable ISR.
This blog will cover how the integration of Deep Learning and AI Cloud technology can deliver remarkable ISR results, including various deep learning approaches, advantages of cloud AI datacenters, and how image super-resolution solutions are transforming industries.
Table of Contents
Introduction to Image Super Resolution (ISR)
The Role of Deep Learning in ISR
Why Use AI Cloud for Image Super Resolution?
Deep Learning Networks for ISR
Case Studies: Industries Leveraging ISR on AI Cloud
Future Trends and Challenges in ISR on AI Cloud
1. Introduction to Image Super Resolution (ISR)
Image Super Resolution is an advanced image-processing technique designed to upscale the resolution of images. Traditional methods relied on interpolation techniques, but with deep learning, ISR achieves unprecedented accuracy and sharpness. It has diverse applications across sectors, such as:
Healthcare: Enhanced diagnostic imaging for more accurate assessments.
Media and Entertainment: High-definition content without requiring native high-resolution footage.
Geospatial Analysis: Satellite and drone imaging with enhanced clarity.
Surveillance: Clearer images from low-resolution footage in security operations.
ISR is essential where clear images are critical, and AI Cloud platforms offer a scalable solution to deploy ISR for these applications.
2. The Role of Deep Learning in ISR
Deep Learning has revolutionized ISR through deep neural networks capable of recognizing and reconstructing intricate image details. Deep learning-based ISR models learn from a large dataset of high-resolution images to infer patterns and details missing in lower-resolution images.
Key advantages of Deep Learning for ISR:
Self-learning capabilities: Deep neural networks can autonomously learn to upscale resolution, adjusting based on unique patterns in the training data.
Accuracy and Clarity: DL models produce high-quality images by learning intricate patterns and image textures.
Adaptability: Models can be retrained for different image types, including medical scans, satellite images, or artistic renderings.
3. Why Use AI Cloud for Image Super Resolution?
AI Cloud platforms offer numerous advantages over traditional hardware deployments for ISR. By utilizing cloud-based deep learning resources, organizations can efficiently train and deploy ISR models without substantial infrastructure investments.
Benefits of Using AI Cloud for ISR:
Scalability: Easily scale up resources based on ISR model requirements without being constrained by on-premises hardware.
AI Datacenters: These provide the computational power necessary for ISR applications, such as high-end GPUs and TPUs, accelerating training and inference.
Cost-effectiveness: Pay-as-you-go pricing models make it affordable to access advanced ISR models without upfront costs.
Integrated tools and services: AI Cloud providers offer pre-built libraries, machine learning operations (MLOps) tools, and data storage options, simplifying model development and deployment.
4. Deep Learning Networks for ISR
Several deep learning networks have emerged as leading solutions for ISR, each offering unique advantages in model architecture and performance. Below are the most commonly used models:
Super-Resolution Convolutional Neural Network (SRCNN)
First deep learning model for ISR: SRCNN was one of the pioneering models for applying deep learning to ISR.
Convolutional Layers: SRCNN uses convolutional layers to learn and enhance features of low-resolution images.
High Accuracy: Achieves good results, though newer models surpass its performance.
Generative Adversarial Network (GAN)
Innovative Architecture: GANs consist of two networks — a generator and a discriminator — that work together to create realistic high-resolution images.
Image Realism: GANs produce images with natural textures, making them suitable for ISR tasks where authenticity is key.
SRGAN: A specialized GAN variant optimized for ISR, providing a balance between resolution and naturalness in the upscaled images.
Enhanced Deep Super-Resolution (EDSR)
Improved over SRCNN: EDSR removes batch normalization to streamline the network, improving ISR performance.
Superior Quality: EDSR achieves unique results, especially in tasks requiring ultra-high resolutions.
Flexible: The model can be modified to handle various ISR tasks across different domains.
Residual Dense Network (RDN)
Residual and Dense Connections: This architecture allows deeper learning, which improves the model’s ability to capture image details.
High Detail Recognition: RDN performs exceptionally well on images with intricate details, making it ideal for applications like satellite imaging.
Transformer-based ISR Models
Attention Mechanism: Transformers apply attention mechanisms that focus on image regions requiring enhancement.
Efficiency: These models often require fewer resources, making them well-suited for cloud-based deployments.
Flexibility: They can handle varying image sizes and types, from medical images to high-definition photography.
5. Case Studies: Industries Leveraging ISR on AI Cloud
Deep Learning and AI Cloud solutions for ISR are being adopted across various industries. Here are some prominent use cases:
Healthcare
Medical Imaging: ISR in medical imaging improves the clarity of MRI, CT, and X-ray scans, enabling better diagnostic accuracy.
Telemedicine: High-quality imaging allows remote diagnoses and consultations, expanding healthcare accessibility.
Media and Entertainment
Video Upscaling: AI Cloud-based ISR is used to upscale lower-resolution videos, making old footage suitable for modern high-definition viewing.
Visual Effects: Enhanced image resolution elevates visual effects quality, producing lifelike graphics.
Geospatial and Satellite Imaging
Enhanced Analysis: ISR on satellite imagery provides greater clarity, aiding in urban planning, agriculture, and environmental monitoring.
Disaster Management: High-resolution images aid in assessing natural disaster impacts and directing relief efforts.
Security and Surveillance
Image Enhancement: ISR helps clarify images in low-resolution surveillance footage, improving incident analysis.
Facial Recognition: Higher resolution improves facial recognition accuracy, even in less-than-ideal imaging conditions.
E-commerce and Retail
Product Visualization: ISR enhances product images, creating a more immersive shopping experience for consumers.
Augmented Reality (AR): High-resolution images improve AR applications, making virtual try-ons and previews more realistic.
6. Future Trends and Challenges in ISR on AI Cloud
Advancements in Transformer Models
- Transformers are gaining popularity due to their efficient processing and adaptability to different image resolutions. They are likely to become even more effective as more sophisticated architectures are developed.
Integration of ISR with Other AI Services
- AI Clouds are increasingly integrating ISR with image classification, segmentation, and object detection models. This integration enables multi-functional applications, especially in fields like healthcare, security, and retail.
Challenges in Privacy and Data Security
- Handling Sensitive Data: ISR applications often involve sensitive images, particularly in sectors like healthcare and security. AI Cloud providers must ensure strong data encryption, secure access controls, and compliance with regulations like GDPR.
Computational Cost Optimization
- AI Datacenters and ISR: Although cloud-based ISR is cost-effective, the computational requirements remain high. New methods are being explored to reduce costs, such as model compression and optimization techniques.
Energy Consumption and Sustainability
- Environmental Impact: AI Datacenters require significant energy, and ISR applications add to this load. Developing energy-efficient deep learning models and sustainable data centers is an ongoing area of research.
Conclusion
Image Super Resolution is a groundbreaking technology with broad applications, from healthcare diagnostics to video streaming and beyond. By utilizing AI Cloud and AI Datacenters, ISR solutions can be efficiently deployed and scaled across industries, achieving high-quality, real-time image enhancement.
The combination of deep learning and cloud computing makes ISR accessible and viable, paving the way for innovative, AI-driven transformations across multiple sectors. As we continue advancing deep learning networks and optimizing cloud infrastructure, the potential for ISR applications will only grow, reshaping how we interact with digital images and creating clearer, more immersive visual experiences.
Subscribe to my newsletter
Read articles from Tanvi Ausare directly inside your inbox. Subscribe to the newsletter, and don't miss out.
Written by
