A Practical Guide to Sociological Research

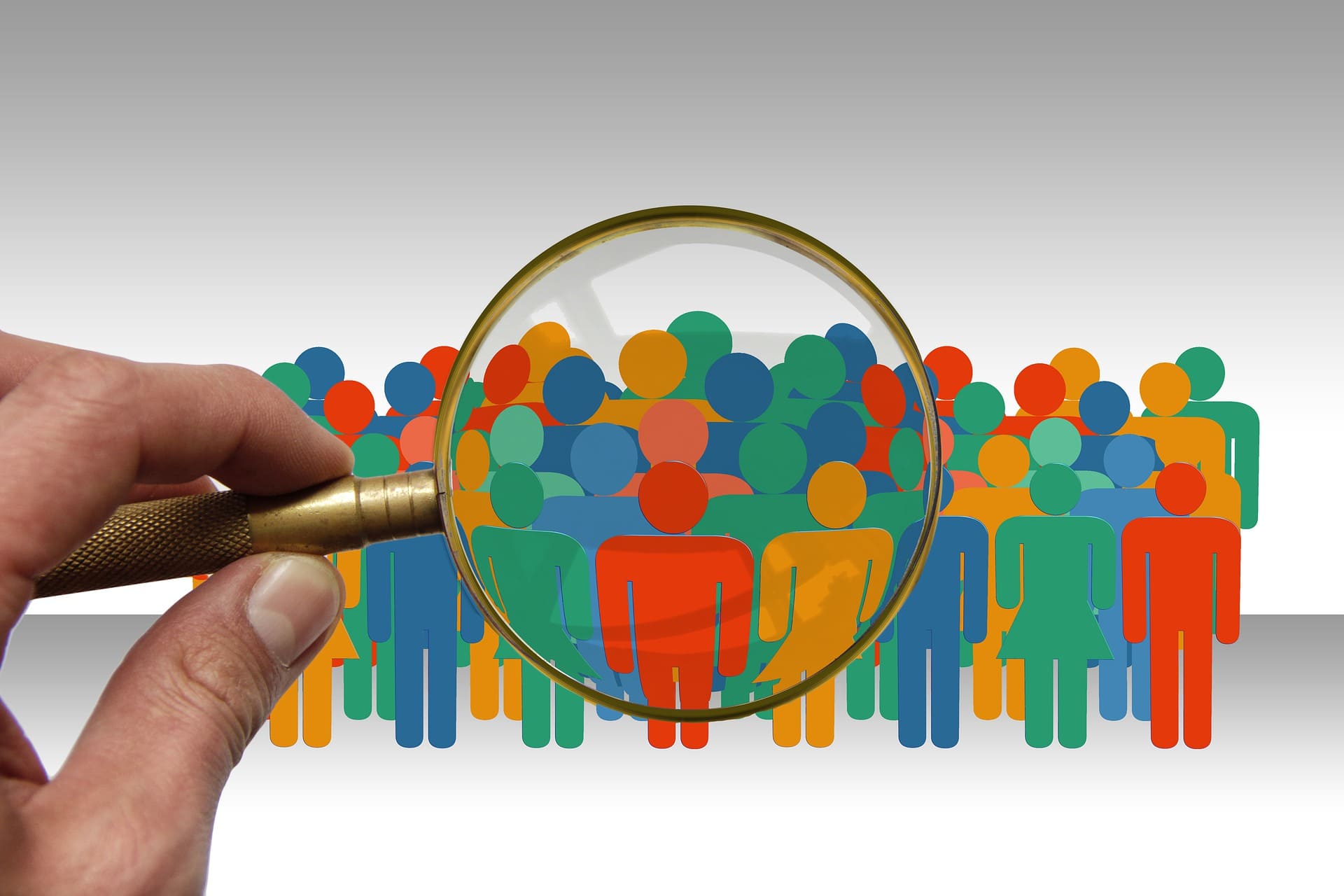
When we think of hobbies, research may not be the first thing that comes to mind. But for the naturally curious, research can be one of the most fulfilling pursuits—a way of not just gathering information but of deepening our understanding of the world. Last time, I wrote about “research as a hobby,” diving into the idea of freestyle research: the kind that’s curiosity-driven, unbound by rigid methodologies, and focused on exploration. This time, we’re building on that foundation, moving from freestyle inquiry to a structured research framework.
In this guide, I’ll walk through each step of the sociological research process with a detailed, data-centric example. Imagine we’re studying income trends in India’s Tier-2 cities, trying to answer questions about the impact of education, age, and gender on income levels. Using structured research methods, we can uncover insights about socioeconomic dynamics in these rapidly developing areas, while gaining practical skills in data analysis and research design.
1. The Research Question and Hypothesis:
Research begins with a question—a point of genuine interest that drives us to seek answers. When transitioning from casual research to formal study, formulating a well-defined research question is essential. This question serves as the study’s purpose, directing every aspect from methodology to data interpretation. For this study on income levels, our initial curiosity could be broad, like “What factors influence income in Indian cities?” but with structure, we refine it into a focused, specific question.
Example Initial Question: What demographic factors influence income levels in India’s Tier-2 cities?
Research Question: To what extent do age, education, and gender predict income levels in India’s Tier-2 cities?
Developing a Hypothesis:
With a question in hand, we move to hypothesizing—a statement of expected outcomes based on existing knowledge or theory. In this case, we may hypothesize that higher education leads to higher income levels, aligned with Human Capital Theory, which posits that investment in education and skills yields economic returns. Hypotheses need to be specific and, ideally, quantifiable, laying the groundwork for empirical testing.
- Hypothesis Example: In Indian Tier-2 cities, individuals with advanced education are likely to have higher income levels than those with lower educational attainment.
This hypothesis takes our research question and translates it into a testable statement that aligns with established sociological theory.
2. Literature Review:
A well-executed literature review frames your study within the larger body of knowledge. For a study on income trends, this means reviewing economic and sociological theories as well as empirical studies on income distribution, labor markets, and socioeconomic demographics in similar urban settings.
Key Theories to Explore
Human Capital Theory: Central to our hypothesis, this theory emphasizes the role of education and skill acquisition in determining income.
Social Stratification Theory: This sociological theory examines how social hierarchy, often related to class, education, and employment, impacts access to resources and, consequently, income.
Gender Stratification Theory: Focusing on how gender affects access to economic resources, this theory can help frame our analysis of income disparities by gender in Tier-2 cities.
By exploring these theories, we create a theoretical foundation, linking our research question to existing knowledge. The literature review should summarize existing findings, identify gaps, and demonstrate how your study will contribute new insights.
Empirical Studies and Data Sources
Look into previous studies, both local and international, that have explored similar themes. Reviewing these studies reveals patterns and methodologies that can inform your own research, helping you refine your approach. Examples include national labor surveys, socioeconomic analyses from government databases, and industry reports on income disparities in developing regions. Studies on urban economics and Tier-2 cities may reveal how these urban centers serve as a bridge between rural and metropolitan dynamics, making them unique in terms of income trends.
3. Research Design:
The research design is your project’s roadmap, specifying how you’ll collect, analyze, and interpret data to answer your research question. For this study, we’ll use a quantitative methodology with a cross-sectional survey design, an approach that captures demographic and income data at a single point in time, enabling us to identify correlations.
Methodological Approach
Quantitative Methodology: By focusing on numerical data, we can quantify relationships between demographics and income levels, making statistical analysis possible.
Cross-Sectional Design: Capturing data from different individuals simultaneously allows us to analyze demographic trends across a diverse group of participants.
Sampling Strategy
Choosing an appropriate sample is crucial for the validity of our results. In our case, a stratified random sample will provide a balanced representation across demographic groups, enabling meaningful comparisons.
Define Strata: Age, education level, and gender are primary variables of interest, so we’ll categorize our sample accordingly.
Determine Sample Size: For significant results, a sample of at least 300-500 participants from different Tier-2 cities is recommended. Use a power analysis to ensure your sample size is large enough to detect meaningful differences between groups.
Recruit Participants: Collaborate with local organizations, use online survey platforms, or engage community centers for in-person surveys.
By specifying these details in advance, you create a clear, replicable structure that ensures robust, generalizable results.
Ethical Considerations
Income data is inherently sensitive, requiring ethical guidelines for privacy, confidentiality, and informed consent. Before starting, develop an ethics protocol, secure consent from participants, and ensure data anonymization. Ethical transparency fosters trust, making participants more likely to provide accurate responses.
4. Data Collection:
With a clear design and ethics plan, it’s time to collect data. This phase translates theoretical questions into concrete observations, providing the raw material for analysis.
Survey Development
Creating a survey involves transforming the research question into quantifiable, clear questions. Survey sections might include:
Demographics: Age, gender, education level, years of experience, and occupation.
Income Information: Monthly or yearly income brackets, formatted to fit local norms.
Additional Context: Optional questions about job satisfaction, perceived job security, and industry type add qualitative context.
Crafting Clear, Unbiased Questions
Carefully word questions to ensure accuracy and avoid bias. Instead of vague questions, use precise income brackets and standard demographic categories. For example, avoid “Do you have a high income?” Instead, ask, “What is your monthly income range?” with clearly defined options.
Pilot Testing the Survey
Pilot testing helps detect issues with question clarity, length, and respondent engagement. Collecting feedback from a small, diverse sample (about 20-30 people) can reveal ambiguities and ensure that questions are accessible to people from varied backgrounds.
Data Collection Techniques
Online Surveys: Online distribution via platforms like Google Forms or Qualtrics offers broad reach and efficiency.
In-Person Surveys: For populations with limited internet access, consider in-person interviews, which allow for deeper engagement and can yield richer qualitative insights.
Hybrid Approach: Combining online and offline methods ensures inclusivity, capturing data from diverse socioeconomic groups.
Data collection is an active process where curiosity remains crucial. Observing respondents’ reactions or noting context during in-person interviews can uncover nuances that enrich your analysis.
5. Data Preparation:
Once data is gathered, preparing it for analysis requires thorough cleaning and organization. This stage is time-consuming but essential; small errors here can lead to significant misinterpretations.
Data Cleaning Steps
Removing Duplicates: Duplicate responses, common in online surveys, can skew data and need removal.
Handling Missing Data: Missing responses are inevitable; decide in advance how to handle them. Common methods include:
Excluding Cases: If only a few values are missing, exclude those cases to preserve data integrity.
Mean Imputation: For larger datasets, filling missing values with the group mean can maintain statistical power.
Coding and Categorizing Data: For qualitative responses (e.g., open-ended perceptions of job security), create coding categories. Use software like NVivo for larger datasets, or code manually if responses are manageable.
Tools for Data Management
Data cleaning is facilitated by tools like Excel, Python’s Pandas library, or SPSS. With organized data, you’re prepared for analysis, confident that the insights you derive will be based on reliable information.
6. Advanced Data Analysis:
Analysis is the stage where data transforms into insights. This study’s focus on demographic influences on income lends itself to quantitative techniques that identify correlations, patterns, and differences.
Descriptive Statistics
Descriptive statistics provide an overview of the dataset’s main characteristics:
Mean, Median, and Mode Income: These indicators reveal central tendencies in income and highlight any income disparities.
Standard Deviation and Range: These measures help understand income variability across demographics.
Inferential Analysis
For deeper insights, inferential statistics explore the relationships and differences between variables:
Correlation Analysis: Using Pearson’s correlation, you can measure the relationship strength between continuous variables, like age and income level.
T-tests and ANOVA: These techniques test for income differences between groups, such as gender or education level, revealing significant patterns.
Multiple Regression Analysis: Regression models examine how demographic factors predict income. A multiple regression, for instance, can show the extent to which age, education, and gender collectively explain income variance.
Software for Data Analysis
SPSS, R, and Python (with libraries like statsmodels
and scikit-learn
) facilitate advanced analyses. SPSS is user-friendly for beginners, while Python and R offer extensive flexibility and are highly customizable.
Example Output
Suppose the regression model shows that education level and age significantly predict income, while gender has a smaller effect. You might find:
Coefficients: Each coefficient indicates the average income increase associated with an additional year of education or age.
R-Squared Value: A high R-squared suggests that the model explains a large proportion of income variation, validating your hypotheses.
Interpreting Findings
Once statistical results are obtained, interpretation requires linking findings back to the research question and theory. For instance, a finding that education predicts income aligns with Human Capital Theory, suggesting that individuals with advanced degrees can demand higher wages. Comparing your findings to past studies strengthens your discussion, positioning your study within the larger academic discourse.
7. Reporting and Presenting Findings:
The research journey culminates in presenting and sharing findings, making the journey and its insights accessible to others. For hobbyist researchers, online platforms, blog articles, or community presentations are effective formats.
Structuring the Report
Introduction and Literature Review: Start with the research question, hypotheses, and theoretical background.
Methods: Describe sampling, data collection, and analysis procedures in detail.
Results: Present descriptive and inferential findings clearly, using tables and graphs for readability.
Discussion: Interpret findings within the context of the research question, theory, and existing literature.
Conclusion and Implications: Summarize key insights and suggest how findings could inform policy or future research on income in developing urban areas.
Visualizing Data
Using graphs, charts, and infographics makes data more digestible and engaging. Visuals like bar charts for income differences by education level or scatter plots showing age-income relationships can clarify complex results.
Reflecting on the Journey:
Reflecting on your research process brings full-circle understanding and reveals how structured research adds depth to curiosity-driven inquiries. Each research step, from hypothesis formulation to data analysis, reinforces the value of disciplined curiosity, blending passion with rigor to produce insights that inform and inspire.
Conclusion
Research, whether casual or structured, is about the pursuit of understanding. By embracing both curiosity and method, you can conduct meaningful studies that contribute to a larger body of knowledge, impacting not just your own understanding but that of others. Whether you’re conducting research as a hobby or for academic purposes, structured inquiry channels curiosity into results that can shape our understanding of complex issues, like income distribution, in tangible, transformative ways.
Research done with passion and rigor offers a window into the world, allowing us to better understand and, perhaps, improve it. This guide is an invitation to take your curiosity further, using structure as a tool to unlock the full potential of discovery.
Thank you for reading. Feel free to reach out to me at AhmadWKhan.com.
Subscribe to my newsletter
Read articles from Ahmad W Khan directly inside your inbox. Subscribe to the newsletter, and don't miss out.
Written by
