What AI Researchers Have Gotten So Wrong

Table of contents
- Introduction
- Misunderstanding AI's Capabilities and Limitations
- The Problem of Data Bias and Privacy Issues
- Overlooking the Need for Interdisciplinary Collaboration
- Ignoring Long-term Consequences and Risks
- Fostering transparency and accountability in AI development is crucial for building trust and ensuring ethical practices in the deployment of artificial intelligence technologies. Transparency involves making the processes and decision-making criteria of AI systems clear and understandable to users and stakeholders. This includes providing detailed documentation on how AI models are trained, the data sources used, and the algorithms implemented. By doing so, developers can help demystify AI systems, allowing users to comprehend how decisions are made and fostering trust in the technology.
- Conclusion
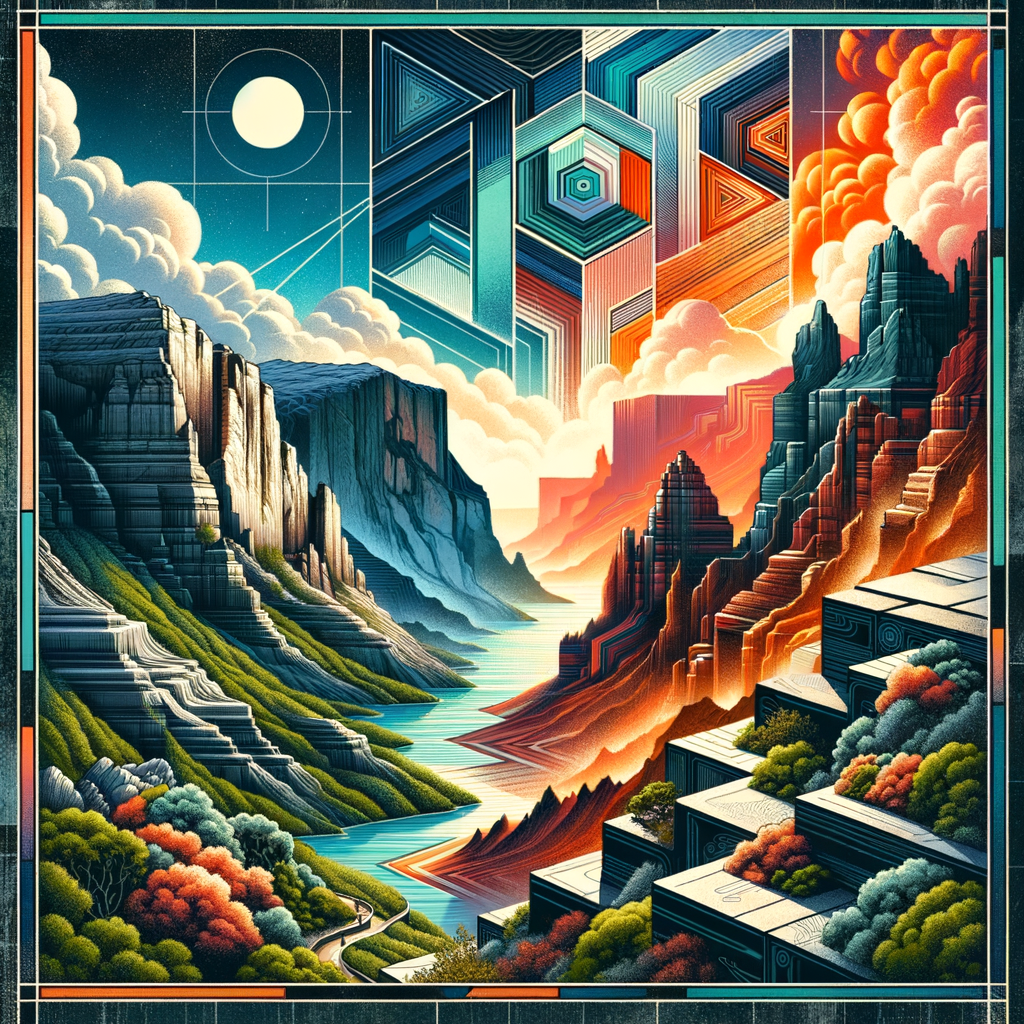
Introduction
Explanation of the growing significance of AI in various fields
The article explores the growing importance of AI across various domains, highlighting common misconceptions such as overestimating its capabilities and underestimating societal impacts. It addresses issues like data bias, privacy concerns, and the need for interdisciplinary collaboration in AI research. The article also emphasizes the importance of transparency, accountability, and long-term considerations in ethical AI development, ultimately advocating for a more responsible and informed approach to AI research.
Brief overview of the common misconceptions and mistakes in AI research
%%[]
Misunderstanding AI's Capabilities and Limitations
Overestimating AI's current capabilities
This article examines the increasing significance of AI across different fields, focusing on common misconceptions like overestimating AI's abilities and underestimating its societal impacts. It discusses issues such as data bias, privacy concerns, and the necessity for interdisciplinary collaboration in AI research. It emphasizes the need for transparency and accountability, while highlighting the importance of ethical and long-term considerations in AI development. The article calls for a more responsible and informed approach to AI research.
Expectations versus reality in AI's performance
The expectations surrounding AI often surpass its current capabilities, leading to a gap between what is anticipated and what is achievable. Many envision AI systems as fully autonomous and capable of human-like reasoning, yet the reality is that AI excels in specific, narrow tasks rather than general intelligence. This disparity can result in over-hyped promises and disillusionment when AI systems fail to deliver expected outcomes. Understanding AI's actual performance limitations is crucial for setting realistic goals and fostering trust in AI technologies.
Examples of AI failures due to overestimation
AI failures often occur when expectations exceed the technology's actual capabilities. For instance, autonomous vehicles have faced challenges in real-world environments, struggling with unpredictable elements like weather conditions and complex traffic scenarios. Similarly, AI chatbots have sometimes failed to understand nuanced human language, leading to unsatisfactory interactions. These examples highlight the importance of recognizing AI's current limitations to avoid over-promising and under-delivering.
Underestimating AI's impact on society
While AI holds great potential, its societal impacts are sometimes underestimated. AI technologies can influence job markets, privacy, and decision-making processes, often in ways that are not immediately apparent. For example, AI-driven automation can lead to job displacement, while biased algorithms can perpetuate discrimination. Acknowledging these impacts is essential for developing policies and frameworks that ensure AI benefits society as a whole.
Ethical and Moral Implications
The ethical and moral implications of artificial intelligence are vast and complex, encompassing a range of issues that society must carefully consider. As AI systems become more integrated into daily life, questions arise about the fairness and transparency of these technologies. For instance, AI algorithms can inadvertently perpetuate biases present in the data they are trained on, leading to unfair treatment of certain groups. This raises concerns about accountability and the need for robust frameworks to ensure that AI systems operate justly and equitably. Moreover, the deployment of AI in sensitive areas such as healthcare, law enforcement, and finance necessitates a thorough examination of ethical standards to protect individual rights and prevent harm.
The Potential for Misuse or Harmful Applications
AI technologies, while offering numerous benefits, also present the potential for misuse or harmful applications. This potential is particularly concerning in areas such as surveillance, where AI can be used to infringe on privacy rights through facial recognition and data tracking. Additionally, the development of autonomous weapons systems poses significant ethical dilemmas, as these technologies could be used in warfare without adequate human oversight. The risk of AI being used for malicious purposes, such as creating deepfakes or conducting cyber-attacks, further underscores the need for stringent regulations and international cooperation to mitigate these threats. It is crucial for policymakers, technologists, and society at large to work together to establish safeguards that prevent the misuse of AI and ensure its applications are aligned with human values and ethics.
The Problem of Data Bias and Privacy Issues
The Significance of Bias in AI Datasets
Bias in AI datasets is a critical issue that can significantly impact the effectiveness and fairness of AI systems. When datasets used to train AI models contain biased information, the resulting AI systems can perpetuate and even amplify these biases. This can lead to outcomes that are not only inaccurate but also unfair, affecting various aspects of society.
How Biased Data Can Lead to Flawed AI Systems
When AI systems are trained on biased data, they can produce skewed results that reflect the underlying prejudices present in the data. For example, if an AI system used for hiring decisions is trained on data that reflects historical gender or racial biases, it may unfairly favor certain groups over others. This can result in flawed decision-making processes that reinforce existing inequalities and discrimination.
Representation Issues and Discrimination Concerns
One of the major challenges with AI datasets is ensuring that they are representative of the diverse populations they are meant to serve. Lack of diversity in training data can lead to AI systems that do not perform well for underrepresented groups, leading to discrimination and exclusion. Addressing these representation issues is crucial to developing AI systems that are equitable and just.
Concerns Over Data Privacy and Misuse
Data privacy is another significant concern when it comes to AI. The vast amounts of personal data required to train AI systems raise questions about how this data is collected, stored, and used. There is a risk that sensitive information could be misused or accessed without consent, leading to privacy violations. Ensuring robust data protection measures and transparent data handling practices is essential to maintaining trust in AI technologies.
The balance between data collection and privacy rights
Case studies on privacy breaches involving AI
Overlooking the Need for Interdisciplinary Collaboration
Interdisciplinary collaboration is crucial in the field of AI research, yet it is often overlooked. The integration of diverse fields such as computer science, ethics, sociology, psychology, and law can significantly enhance the development and implementation of AI technologies. By bringing together experts from various disciplines, we can address complex challenges more effectively and create AI systems that are not only technically advanced but also socially responsible and ethically sound.
The Importance of Integrating Diverse Fields in AI Research
Integrating diverse fields in AI research allows for a more comprehensive understanding of the implications and potential impacts of AI technologies. For instance, computer scientists can work alongside ethicists to ensure that AI systems are designed with ethical considerations in mind. Similarly, collaboration with sociologists can help identify and mitigate potential social biases in AI algorithms. By involving legal experts, we can navigate the regulatory landscape and ensure compliance with data protection laws. This holistic approach fosters innovation and helps build AI systems that are aligned with societal values and needs.
Examples of Successful Interdisciplinary Projects
There are numerous examples of successful interdisciplinary projects in AI research. One notable example is the development of AI-driven healthcare solutions, where collaboration between medical professionals, data scientists, and engineers has led to advancements in diagnostic tools and personalized medicine. Another example is the creation of AI systems for environmental monitoring, where ecologists, data analysts, and software developers work together to track and predict ecological changes. These projects demonstrate how interdisciplinary collaboration can lead to groundbreaking innovations that address real-world problems.
Consequences of a Lack of Collaboration
Failing to embrace interdisciplinary collaboration in AI research can lead to several negative consequences. Without input from diverse fields, AI systems may be developed with narrow perspectives, resulting in technologies that do not adequately address ethical, social, or legal considerations. This can lead to biased outcomes, public distrust, and even legal challenges. Moreover, the lack of collaboration can stifle innovation, as valuable insights from other disciplines are not incorporated into the research process. Ultimately, neglecting interdisciplinary collaboration can hinder the development of AI systems that are truly beneficial to society.
Ignoring Long-term Consequences and Risks
When developing AI technologies, it is crucial to consider the long-term consequences and potential risks associated with their deployment. These concerns often do not receive the attention they deserve, which can lead to unforeseen challenges down the line.
Insufficient Attention to AI Safety and Security
One of the primary areas of concern is the lack of sufficient focus on AI safety and security measures. As AI systems become more integrated into critical infrastructure and everyday life, ensuring their reliability and security becomes paramount. Without rigorous safety protocols, AI systems could malfunction or be exploited by malicious actors, leading to significant harm.
Risks Related to Autonomous Systems
Autonomous systems, such as self-driving cars and drones, present unique risks that need careful consideration. These systems operate with a high degree of independence, which can lead to unpredictable behavior in complex environments. The potential for accidents, misuse, or failure in these systems raises important questions about liability and accountability.
The Future Ethical and Societal Impacts of AI Advancement
As AI technology continues to advance, it is essential to consider its ethical and societal implications. The widespread adoption of AI could lead to significant changes in employment, privacy, and social dynamics. It is important to engage in ongoing dialogue about these impacts to ensure that AI development aligns with societal values and promotes the well-being of all individuals.
Fostering transparency and accountability in AI development is crucial for building trust and ensuring ethical practices in the deployment of artificial intelligence technologies. Transparency involves making the processes and decision-making criteria of AI systems clear and understandable to users and stakeholders. This includes providing detailed documentation on how AI models are trained, the data sources used, and the algorithms implemented. By doing so, developers can help demystify AI systems, allowing users to comprehend how decisions are made and fostering trust in the technology.
Accountability, on the other hand, requires establishing clear lines of responsibility for the outcomes produced by AI systems. This means identifying who is responsible for the actions of AI, whether it be the developers, the companies deploying the technology, or the policymakers regulating its use. Implementing robust accountability measures ensures that when AI systems cause harm or operate in unintended ways, there are mechanisms in place to address these issues and provide remedies.
To achieve these goals, it is essential to develop comprehensive guidelines and standards that govern AI development and deployment. This includes setting up independent oversight bodies to monitor AI systems, conducting regular audits to ensure compliance with ethical standards, and engaging with diverse stakeholders, including ethicists, legal experts, and affected communities, to gather a wide range of perspectives.
Moreover, fostering an open dialogue between AI developers and the public can help address concerns and build a shared understanding of the benefits and risks associated with AI technologies. By prioritizing transparency and accountability, we can create AI systems that not only advance technological progress but also align with societal values and ethical principles.
The Role of Transparency in Building Trustworthy AI
Transparency plays a crucial role in developing AI systems that users can trust. It involves openly sharing information about how AI systems are designed, how they function, and the data they use. By providing clear and accessible documentation, developers can help users understand the decision-making processes of AI systems. This openness not only fosters trust but also allows for external scrutiny, which can identify potential biases or errors. Transparency ensures that stakeholders, including users, developers, and regulators, have a clear understanding of AI operations, which is essential for building confidence in these technologies.
Importance of Holding Researchers Accountable for AI Outcomes
Holding researchers accountable for the outcomes of AI systems is vital to ensure responsible development and deployment. Researchers must be aware of the potential impacts their creations can have on society and take steps to mitigate any negative consequences. This accountability involves establishing clear lines of responsibility, where researchers, developers, and organizations must answer for the actions of their AI systems. By implementing accountability measures, we can ensure that when AI systems cause harm or operate in unintended ways, there are mechanisms to address these issues and provide remedies. This responsibility encourages ethical practices and helps maintain public trust in AI technologies.
Proposed Frameworks and Guidelines for Ethical AI Practices
To guide the ethical development and use of AI, several frameworks and guidelines have been proposed. These frameworks aim to establish standards that ensure AI systems are developed and deployed in ways that align with ethical principles and societal values. Proposed guidelines often include principles such as fairness, transparency, accountability, and privacy protection. They suggest the creation of independent oversight bodies to monitor AI systems and recommend regular audits to ensure compliance with ethical standards. Engaging with diverse stakeholders, including ethicists, legal experts, and affected communities, is also emphasized to gather a wide range of perspectives. By adopting these frameworks, we can create AI systems that not only drive technological progress but also respect and uphold ethical standards.
Conclusion
Recap of the Key Issues Researchers Have Overlooked in AI Development
In the rapidly evolving field of AI development, several critical issues have often been overlooked by researchers. One major concern is the lack of comprehensive understanding of the ethical implications of AI technologies. Many researchers focus primarily on technical advancements, sometimes neglecting the broader societal impact. Additionally, there is a tendency to underestimate the importance of transparency in AI systems, which can lead to a lack of trust among users. Another overlooked issue is the potential for bias in AI algorithms, which can perpetuate or even exacerbate existing inequalities. Furthermore, the need for robust data privacy measures is frequently underestimated, risking the exposure of sensitive information. These issues highlight the necessity for a more holistic approach to AI development that considers both technical and ethical dimensions.
Call to Action for a More Responsible and Informed Approach to AI Research
Given these overlooked issues, it is crucial for the AI research community to adopt a more responsible and informed approach. Researchers are encouraged to integrate ethical considerations into every stage of AI development, from initial design to deployment. This involves actively seeking diverse perspectives, including those from ethicists, sociologists, and affected communities, to ensure that AI systems are fair and equitable. Transparency should be prioritized, with researchers making efforts to explain how AI systems work and the decisions they make. Addressing bias is another critical area, requiring rigorous testing and validation to ensure AI systems do not reinforce existing prejudices. Moreover, implementing strong data privacy protections is essential to safeguard user information. By taking these steps, researchers can contribute to the development of AI technologies that are not only innovative but also aligned with societal values and ethical standards.
Subscribe to my newsletter
Read articles from Gareth Roberts directly inside your inbox. Subscribe to the newsletter, and don't miss out.
Written by

Gareth Roberts
Gareth Roberts
I have a degree in Psychology and a PhD in Cognitive Neuroscience, Developmental Psychology, and Artificial Intelligence. After my PhD I did three postdoctoral research fellowships before moving across to industry. I started with Data and Statistical Consulting before moving over to several startups in the ecommerce and proptech space. Subsequently, I've held positions such as Chief Technical Officer, Head of Data Analytics, and Head of AI across a broad range of sectors including mineral exploration, life insurance, and legal analysis. I completed an online MBA in 2020 and have a lot of Deep Learning certifications but I'm all about the self-learning.