The Transformer OpenAI’s Rebranding: From Language Model to "AI Intelligence"

Table of contents
- Origins: The Transformer Architecture
- The Technical Foundation
- The ChatGPT Pivot
- The Technological Sleight of Hand
- The GPT-4 Escalation and Authority Building
- Breaking Down the Illusion
- The Technical Reality Check: Key Research Findings
- The Cost of Rebranding
- The Marketing Timeline
- The Path to Clarity
- Implications for Future Development
- The Democratisation of Transformers Today
- Conclusion
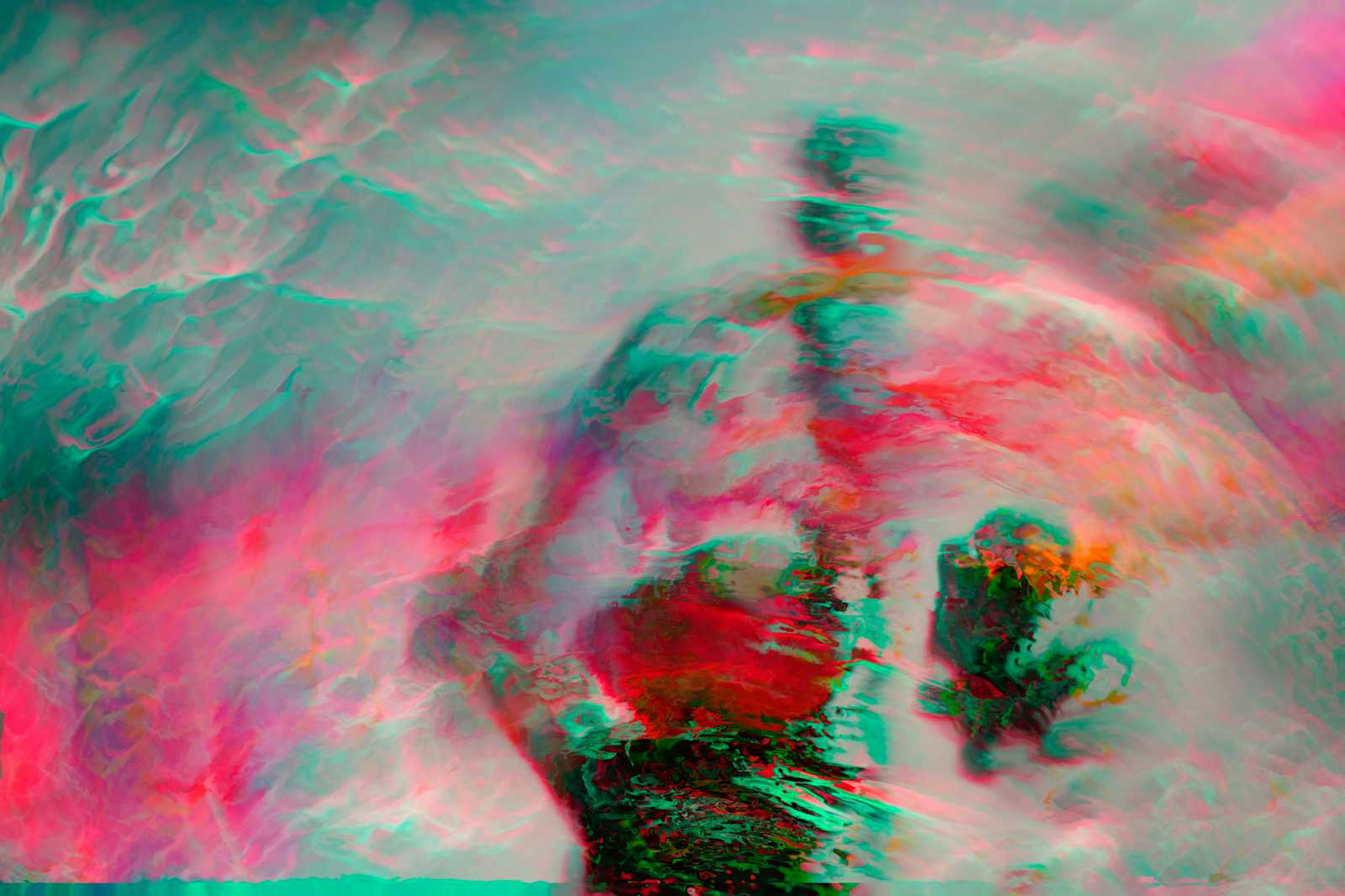
In the realm of artificial intelligence, we are witnessing one of the most remarkable feats of technological rebranding in history—though not for the reasons commonly celebrated. The transformer architecture, fundamentally unchanged since its 2017 introduction, has been masterfully repackaged from a specialized language processing tool into something far grander in the public imagination. This transformation represents not a technological revolution, but rather a triumph of marketing over technical reality.
What makes this rebranding particularly noteworthy is its audacity: presenting the same product—a pattern-matching system based on attention mechanisms—as something entirely different without any fundamental technical evolution to support these claims. Like a skilled illusionist directing attention away from the mechanics of a trick, the AI industry has shifted focus from what transformers actually do to what they appear to do, all while the underlying technology remains essentially unchanged.
Origins: The Transformer Architecture
When Google introduced the Transformer architecture in their seminal "Attention Is All You Need" paper in 2017, its purpose was clear and specific: advancing natural language processing through a novel attention mechanism. Unlike today's grandiose claims, the original goals were remarkably straightforward—process sequences of tokens, learn patterns in language data, generate probable next tokens, and handle translation tasks effectively.
The simplicity and effectiveness of this approach would soon prove revolutionary, though not in the way its creators intended. The Transformer began as a specialised NLP tool—not as the 'brain' it's marketed as today.
The Technical Foundation
The architecture's power lies in its elegant simplicity. At its core, the transformer relies on a set of mechanisms that remain largely unchanged since its inception. The self-attention mechanisms allow the model to weigh the importance of different parts of input sequences. Feed-forward networks process this information, while positional encoding maintains sequence order. These components work in concert to enable sophisticated pattern recognition through weights—all without any semblance of true understanding or reasoning.
The ChatGPT Pivot
The Marketing Metamorphosis
The release of ChatGPT in late 2022 marked a watershed moment—not in technical capability, but in public perception. Through careful positioning and marketing prowess, OpenAI transformed the public's understanding of what was essentially the same technology. This shift wasn't subtle; it was a complete reframing of the narrative.
Where once stood a "language model" now stood an "AI intelligence." The "pattern completion system" became a "reasoning engine." The "next-token predictor" transformed into an "understanding agent." This remarkable pivot happened without any fundamental change to the underlying technology.
The Technological Sleight of Hand
The true changes behind ChatGPT's success were more modest than the marketing suggested. While RLHF alignment improved output quality and instruction fine-tuning enhanced usability, the core architecture remained unchanged. The fundamental operations—token prediction, pattern matching, and probability calculations—continued to drive every interaction, though now hidden behind a more sophisticated interface.
The GPT-4 Escalation and Authority Building
The narrative reached new heights with GPT-4's release, bolstered by a carefully orchestrated series of authoritative endorsements. Starting with Microsoft's technical paper suggesting "sparks of AGI," the story gained momentum through a sequence of high-profile events that lent credibility to increasingly dramatic claims:
The authority-building timeline unfolded with precision:
Technical Authority Phase:
Microsoft's "AGI sparks" paper provided academic legitimacy
Industry leaders offered strategic endorsements
Research institutions aligned with the narrative
Political Validation:
Senate hearings on AI risks elevated concerns to national importance
A public letter warning of extinction risks garnered global attention
Media amplification created a sense of urgency
Scientific Endorsement:
Geoffrey Hinton's departure from Google served as a powerful symbol
Nobel laureate backing lent unprecedented credibility
The academic community's engagement reinforced the narrative
Each event built upon the previous, creating a self-reinforcing cycle of authority and urgency. This carefully constructed narrative transformed a sophisticated pattern-matching system into a perceived existential threat—all without any fundamental change to its capabilities.
Breaking Down the Illusion
The gap between marketing and reality grows wider with each new model release, yet the fundamental truth remains unchanged: today's most advanced AI systems are, at their core, still transformer-based architectures. Despite the sophisticated veneer and impressive outputs, they continue to operate as they always have—as probability calculators processing sequences of tokens.
This reality becomes clearer when we examine what these systems actually do rather than what they're claimed to do. They remain, fundamentally, highly sophisticated pattern matchers and sequence completers. Their outputs, while often impressive, emerge from statistical correlations rather than genuine understanding or reasoning.
The Technical Reality Check: Key Research Findings
While marketing narratives soared, technical research began revealing fundamental limitations. A series of rigorous studies systematically dismantled claims of reasoning capabilities:
The Evidence Timeline
August 2023: GPT-4 Can’t Reason Paper
Landmark paper directly challenges GPT-4's reasoning abilities
Demonstrates fundamental inability to handle systematic reasoning tasks
Shows "flashes of brilliance" are pattern-matching artifacts
Highlights dangerous gap between marketing claims and technical reality
October 2024: Apple's Comprehensive Study
Extends findings to frontier models including OpenAI o1 series
Proves reasoning limitations are fundamental to architecture
Shows performance collapses with minimal complexity increases
Confirms LLMs perform pattern matching, not logical reasoning
Pattern Matching vs. True Reasoning
These papers didn't just challenge specific models—they exposed the fundamental disconnect at the heart of transformer marketing. While companies promoted their models as capable of "system 2 thinking" and "PhD-level reasoning," technical research revealed:
High variance in performance on similar problems
Brittleness when facing slight variations
Sensitivity to irrelevant information
Inability to maintain consistent logical chains
This evidence suggests that even the most advanced models, including the latest OpenAI o1 series, remain sophisticated pattern matchers rather than reasoning systems—exactly what the original transformer architecture was designed to be.
The Cost of Rebranding
The consequences of this elaborate rebranding extend far beyond marketing, creating ripple effects throughout academia, industry, and society. Each sphere faces unique challenges stemming from this disconnect between reality and perception.
Academic Impact
In the academic realm, the transformation has fundamentally altered the research landscape. What began as clear technical discussions have become muddied by anthropomorphic terminology and inflated expectations. Resources that might have advanced our understanding of language models' actual capabilities are now diverted toward speculative concerns about artificial general intelligence and existential risks.
This shift hasn't just confused terminology—it has redirected entire research programs. Young researchers, drawn by the allure of working on "AI consciousness" or "machine reasoning," may overlook the fundamental questions still unanswered about these systems' actual operations and limitations.
Industry Impact
The business world has embraced the transformer rebranding with costly enthusiasm. Companies, driven by FOMO and market pressures, rush to implement "AI intelligence" solutions that are often misaligned with their actual needs. This has led to:
Inflated expectations that lead to failed projects
Misguided implementations that solve the wrong problems
Massive investments in capabilities that don't exist
Accumulating technical debt from premature AI adoption
The result is a growing disconnect between promised capabilities and delivered value, threatening to create another AI winter when reality fails to meet expectations.
Social Impact
Perhaps the most profound cost of this rebranding lies in its social implications. The public, bombarded with messages about "intelligent AI" and existential risks, struggles to distinguish between science fiction and technical reality. This confusion has led to:
Widespread misconceptions about AI capabilities
Policy discussions based on imagined rather than actual risks
Ethical debates that miss the mark
Erosion of trust in genuine technological advances
The Attribution Crisis
While the industry focuses on marketing transformers as reasoning engines, it simultaneously obscures crucial questions about training data sources and attribution. This opacity serves the rebranding narrative by preventing scrutiny of what these models actually are: pattern matchers trained on vast amounts of uncredited data. The lack of transparency around data sources not only raises ethical concerns but also makes it impossible to properly evaluate claims of "PhD-level intelligence" or "system 2 thinking."
The Marketing Timeline
The evolution of transformer marketing tells a story of escalating claims and diminishing technical accuracy:
2017: Transformer Introduction
Technical presentations focused on specific capabilities
Clear communication about limitations and use cases
Academic discourse grounded in reality
2022: ChatGPT Release
Marketing shifted toward anthropomorphic language
Capabilities increasingly overstated
Technical details obscured by user experience
2023: GPT-4 "AGI Sparks"
Marketing completely divorced from technical reality
Claims of reasoning and understanding became commonplace
Speculation about consciousness entered mainstream discussion
2024: OpenAI o1 Models and System 2 Claims
PhD-level intelligence claims for OpenAI o1 models
Reinforcement Learning marketed as "system 2 thinking"
UI/UX designed to reinforce reasoning illusion
The Path to Clarity
Recovering from this marketing-induced confusion requires a concerted effort from all stakeholders in the AI ecosystem. The path forward demands both honesty and clarity.
Technical Honesty
The first step toward clarity requires returning to technical fundamentals. This means:
Acknowledging the transformer's true nature as a pattern-matching system
Clearly explaining actual capabilities without anthropomorphic language
Defining and communicating real limitations
Marketing Reform
Responsible marketing doesn't mean downplaying achievements—it means accurately representing them. This requires:
Moving away from anthropomorphic messaging that misleads
Focusing on concrete, demonstrable capabilities
Providing accurate descriptions of how these systems actually work
Public Education
Building public understanding requires sustained effort to:
Explain technical foundations in accessible terms
Clarify how these systems actually operate
Dispel myths about artificial general intelligence
Foster realistic expectations about AI capabilities
Data Transparency and Attribution
Before claims of reasoning or intelligence can be meaningfully evaluated, the industry must address fundamental questions of data sourcing and attribution. This includes:
Clear documentation of training data sources
Attribution mechanisms for generated content
Metrics focused on relevance rather than superficial benchmarks
Implications for Future Development
Moving forward productively requires a fundamental reset in how we think about and discuss AI development. This means:
Realistic Assessment:
Understanding current capabilities
Acknowledging real limitations
Setting achievable goals
Ethical Considerations:
Focusing on actual rather than imagined risks
Developing appropriate safeguards
Ensuring responsible deployment
Technical Progress:
Building on solid understanding
Advancing capabilities methodically
Maintaining scientific integrity
The path forward requires shifting focus from marketing-driven metrics to fundamental improvements in:
Data quality and attribution systems
Transparent evaluation frameworks
Ethical data collection practices
The Democratisation of Transformers Today
The narrative surrounding transformer models, particularly those developed by OpenAI, often invokes the idea of a "special sauce"—a proprietary advantage setting them apart. However, the rapid advancement and accessibility of open-source models, notably Meta's Llama series and Google's Gemma, directly challenge this narrative. This democratization of transformer technology has profound implications for the future of AI development.
Erosion of Perceived Advantage: The performance of open-source models now routinely matches or exceeds that of ChatGPT, both free and paid versions. This challenges the notion of a significant technical gap and suggests that supposed proprietary advantages are readily replicable. Furthermore, achieving high performance with significantly fewer parameters highlights superior engineering in the open-source community.
Democratization and its Impact: The availability of high-performing open-source models underscores that innovation doesn't require secrecy. Parameter efficiency innovations, reducing model size from billions to millions of parameters while maintaining performance, demonstrate the power of open collaboration and community-driven optimization. This shift significantly lowers the barrier to entry for researchers and developers, fostering a more inclusive and rapidly evolving AI landscape.
Rethinking the Narrative: The "special sauce" myth parallels the broader rebranding of transformers discussed earlier. Just as pattern-matching was reframed as "AI intelligence," OpenAI's early market lead was portrayed as inherent technological superiority. The reality is that core improvements stem from better engineering, more efficient architectures, improved training methodologies, and community contributions – aspects readily available in the open-source domain.
Implications for the Future: This democratization signals a critical turning point. The focus should shift towards:
Parameter Efficiency: Optimizing performance with smaller models.
Data Quality and Attribution: Addressing the critical issues of data sourcing and transparency.
Specialized Applications: Tailoring models for specific tasks and domains.
Deployment Optimisation: Making models more efficient and accessible for real-world use.
Conclusion
The journey of the transformer architecture—from specialized NLP tool to perceived harbinger of artificial general intelligence—stands as a testament to the power of narrative crafting in technology. Through careful authority stacking and strategic messaging, a pattern-matching system has been elevated to mythological status, creating ripple effects throughout academia, industry, and society.
As we look toward the future of AI development, our greatest challenge may not be technical but narrative: how do we maintain scientific integrity while navigating the powerful currents of public perception and institutional authority? The answer lies in returning to fundamental truths—understanding what these systems actually are and can do, rather than what marketing suggests they might become.
The transformer's story serves as both warning and guide. Only by recognizing the mechanisms of authority-building and resisting fear-based decision-making can we build a future where AI development is guided by technical reality rather than marketing fiction. The technology's true potential lies not in mythologized threats or exaggerated capabilities, but in its actual, remarkable ability to process and pattern-match language—a capability that, properly understood and applied, can transform our world without transforming our understanding of intelligence itself.
Subscribe to my newsletter
Read articles from Gerard Sans directly inside your inbox. Subscribe to the newsletter, and don't miss out.
Written by

Gerard Sans
Gerard Sans
I help developers succeed in Artificial Intelligence and Web3; Former AWS Amplify Developer Advocate. I am very excited about the future of the Web and JavaScript. Always happy Computer Science Engineer and humble Google Developer Expert. I love sharing my knowledge by speaking, training and writing about cool technologies. I love running communities and meetups such as Web3 London, GraphQL London, GraphQL San Francisco, mentoring students and giving back to the community.