Generative AI in Healthcare: Transforming Medicine and Patient Care
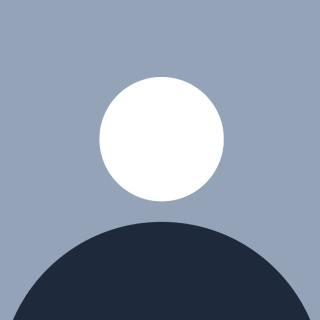
In recent years, artificial intelligence (AI) has made significant strides in healthcare, offering revolutionary solutions across various aspects of the industry. Among the most exciting developments is generative AI in healthcare, a branch of AI that focuses on creating new, synthetic data or content through advanced machine learning models. Unlike traditional AI, which primarily analyzes and processes existing data, generative AI can generate new insights, designs, and even medical treatments, presenting new opportunities for healthcare innovation.
In this blog, we’ll explore how generative AI in healthcare is transforming medicine, improving patient care, and reshaping the healthcare landscape.
What is Generative AI in Healthcare?
Generative AI refers to AI systems that can generate new data or content based on existing information, often through techniques like deep learning and neural networks. In healthcare, generative AI goes beyond simply analyzing patient data or medical records. It has the potential to create synthetic data for research, help design personalized treatment plans, aid in drug discovery, and even generate medical imaging and diagnostic tools.
For example, a generative AI model can analyze a vast amount of medical data—such as patient histories, lab results, and imaging scans—and generate new, synthetic data that mimics the structure and patterns of real data. This synthetic data can then be used to train other AI systems, helping to reduce the risk of bias and improve model accuracy.
Applications of Generative AI in Healthcare
Generative AI is quickly becoming a powerful tool across various domains in healthcare. Some of its most promising applications include:
1. Drug Discovery and Development
One of the most exciting uses of generative AI in healthcare is in drug discovery and the development of new therapies. Traditional drug discovery is a time-consuming, expensive process that can take years or even decades to bring a drug to market. Generative AI, however, can accelerate this process by predicting molecular structures and generating potential drug candidates.
AI models can analyze millions of chemical compounds, learn from past successes and failures, and propose new molecular structures that are likely to be effective against specific diseases. This has the potential to revolutionize the pharmaceutical industry, significantly reducing the time and cost required for drug development.
For example, AI algorithms have already been used to design novel antibiotics that could potentially fight antibiotic-resistant bacteria—an issue that has been a growing concern in global health. Similarly, generative AI in healthcare has contributed to the rapid development of COVID-19 vaccines by analyzing viral genomes and predicting the most effective vaccine formulations.
2. Personalized Medicine and Treatment Plans
Generative AI in healthcare is also paving the way for more personalized and precise medical treatments. Traditionally, treatments and medications are prescribed based on general patient categories, such as age, gender, and diagnosis. However, this one-size-fits-all approach doesn’t account for the unique genetic makeup, environment, and lifestyle of each patient.
Generative AI models can analyze a patient’s genetic data, medical history, and other personal factors to generate individualized treatment plans. These plans can suggest specific medications, dosage adjustments, and lifestyle modifications that are tailored to the patient’s unique needs. Personalized treatments are likely to be more effective and come with fewer side effects, improving patient outcomes and overall quality of care.
3. Medical Imaging and Diagnostics
Another promising application of generative AI in healthcare is in medical imaging and diagnostics. AI-powered models can be trained to generate high-quality medical images, such as MRIs, CT scans, or X-rays, based on limited input data. This can be especially useful in settings where high-quality imaging equipment is unavailable or where images are of low quality.
For example, generative AI models can enhance low-resolution images, improving diagnostic accuracy. In oncology, AI systems have already shown promise in generating synthetic medical images of cancerous tissues and predicting the growth or spread of tumors. By creating accurate synthetic images, generative AI can help doctors detect diseases at earlier stages, when treatment is more likely to be successful.
4. Synthetic Healthcare Data for Research
A significant challenge in healthcare research is the scarcity and privacy concerns surrounding patient data. Generative AI in healthcare can address this issue by generating synthetic healthcare data that resembles real-world patient information without violating privacy regulations such as HIPAA (Health Insurance Portability and Accountability Act).
Researchers can use synthetic data generated by AI models to conduct studies, test hypotheses, and train AI systems without risking patient confidentiality. This synthetic data can include medical records, lab test results, and other health-related information, all of which can be used to train algorithms and improve AI-driven tools.
Moreover, generative AI can simulate various scenarios, allowing researchers to model the progression of diseases and the effectiveness of different interventions. This enables researchers to test treatment options in a controlled environment before conducting clinical trials.
5. Virtual Health Assistants and Chatbots
Virtual health assistants and chatbots powered by generative AI in healthcare can provide patients with personalized medical advice, reminders, and real-time health monitoring. These AI-driven assistants can engage in conversations with patients, answer questions about symptoms, and even suggest lifestyle changes based on individual health data.
For example, generative AI can help a patient track their daily habits—such as diet, exercise, and sleep patterns—and offer suggestions to improve their well-being. These virtual assistants can also monitor a patient’s chronic conditions, such as diabetes or hypertension, and alert healthcare providers if abnormalities are detected.
6. Clinical Trial Design
The design of clinical trials is another area where generative AI in healthcare can make a significant impact. Clinical trials often face issues such as patient recruitment challenges, biased sampling, and inefficient study designs. Generative AI can optimize the design of clinical trials by simulating patient populations, predicting outcomes, and identifying the most relevant factors to include in a study.
AI models can also generate synthetic data to help with the initial stages of clinical trial design, allowing researchers to test different hypotheses before recruiting real participants. This can speed up the process of drug development and improve the accuracy of clinical trial results.
Challenges and Considerations
While the potential of generative AI in healthcare is immense, there are several challenges that need to be addressed for its widespread adoption:
Data Quality and Bias: AI models rely heavily on the quality and diversity of data. If the data used to train generative AI models is biased or incomplete, it can lead to inaccurate or unfair outcomes.
Regulatory and Ethical Issues: The use of generative AI in healthcare raises important ethical and regulatory questions, especially around data privacy, consent, and the transparency of AI decision-making. Ensuring that AI-generated data and treatment recommendations are safe and compliant with regulations is crucial.
Acceptance and Trust: Healthcare providers and patients must trust AI-powered tools for them to be effective. Ensuring that generative AI models are explainable and transparent is essential for building that trust.
Conclusion
Generative AI in healthcare has the potential to revolutionize the way we approach medicine, from drug discovery and personalized treatments to medical imaging and clinical trials. By harnessing the power of AI to generate new data, designs, and insights, healthcare providers can offer more efficient, personalized, and effective care.
As AI technologies continue to evolve, it’s important to address challenges related to data quality, regulation, and trust. With proper safeguards in place, generative AI can drive the next wave of innovation in healthcare, ultimately improving patient outcomes and transforming the future of medicine.
Subscribe to my newsletter
Read articles from Kevin Anderson directly inside your inbox. Subscribe to the newsletter, and don't miss out.
Written by