Computer Vision's Impact on Autonomous Vehicles: Obstacles and Future Insights

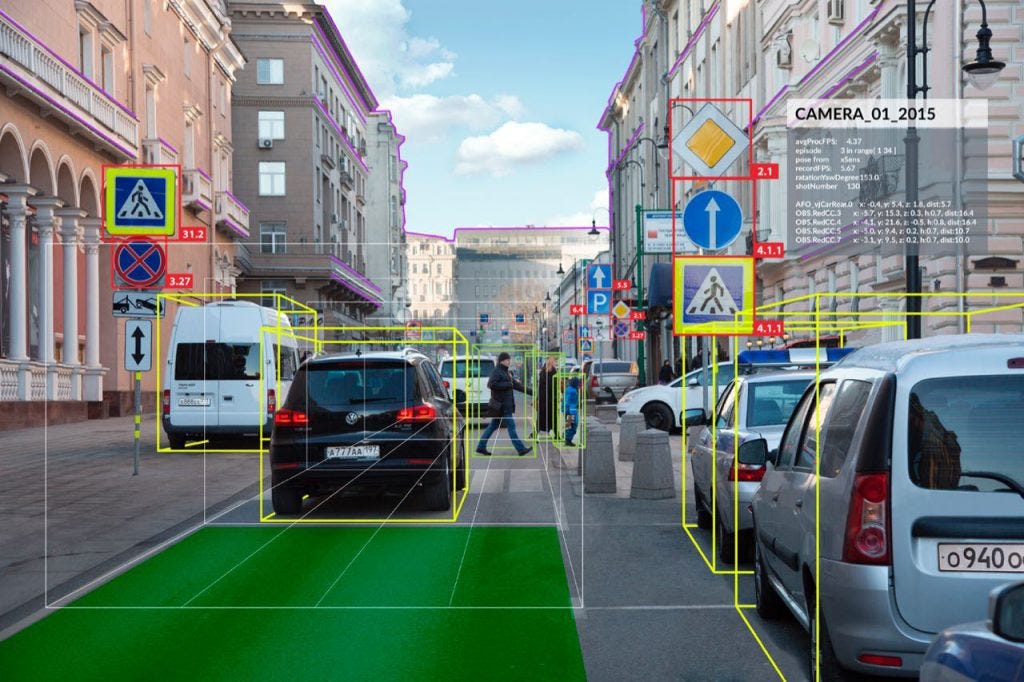
In the world of autonomous vehicles, computer vision is the driving force behind machine perception, allowing vehicles to interpret the environment and make safe decisions on the road. Here’s a look at its role, challenges, and exciting future potential.
Computer vision enables self-driving vehicles to recognize and track objects, interpret road signs, detect lanes, and understand traffic conditions. By analyzing data from cameras, LIDAR, and RADAR sensors, autonomous systems can make split-second decisions—transforming vehicles into intelligent, self-sufficient navigators.
Core Functions of Computer Vision in AV
Object Detection & Tracking: Recognizing other cars, pedestrians, and cyclists in real-time.
Scene Understanding: Interpreting road layouts and potential hazards.
Depth Estimation: Calculating distances to navigate through space safely.
Challenges in Implementing Computer Vision
Despite advances, several challenges persist:
Adverse Conditions: Low light, rain, and fog can obstruct sensors, compromising accuracy.
Complex Urban Environments: Urban streets are unpredictable; vehicles must adapt to dynamic surroundings.
Edge Cases: Unusual scenarios—like unexpected animals or temporary roadwork—are difficult to account for.
Data Latency: Real-time processing is critical for safety, requiring high computing power with minimal delay.
To overcome these challenges, exciting innovations are emerging.
The Future of Computer Vision in Autonomous Vehicles
Improved AI Models: Advanced neural networks, like CNNs and transformers, enhance accuracy and adaptability.
3D Vision & Depth Sensing: Advanced algorithms are enabling better spatial understanding, critical for navigating complex spaces.
Synthetic Data: Simulated data helps train models on rare events, without the need for risky real-world testing.
Edge Computing: Real-time, on-device processing reduces latency and allows faster responses on the road.
Ethical and Regulatory Considerations
With computer vision capturing massive data, privacy, accountability, and transparency become essential. Who is responsible if a system error occurs? How should data from streets and pedestrians be securely stored? As these technologies grow, regulatory frameworks will be key to ensuring safe, responsible adoption.
Conclusion
Computer vision is revolutionizing autonomous vehicles, bringing us closer to a future of safer, smarter roads. While challenges like weather resilience and ethical issues remain, rapid advancements in AI, sensor fusion, and edge computing are pushing the boundaries of what’s possible. As this technology matures, computer vision will play an even more critical role in making autonomous transportation a reality worldwide.
Subscribe to my newsletter
Read articles from ADARSH TIWARI directly inside your inbox. Subscribe to the newsletter, and don't miss out.
Written by

ADARSH TIWARI
ADARSH TIWARI
👨💻 Computer Vision Engineer | AI Enthusiast | Problem Solver Hi there! I'm a passionate Computer Vision Engineer with a focus on deep learning, image processing, and AI-driven solutions. I specialize in developing state-of-the-art models and Automation systems that interpret and understand visual data, enabling machines to see, learn, and make decisions like never before. 🔍 What I Do: Develop and optimize deep learning models for image classification, object detection, and segmentation. Work with cutting-edge frameworks like TensorFlow, PyTorch, and OpenCV. Dive into real-world applications like autonomous driving, medical imaging, and robotics. 💡 My Passion: I thrive on the challenge of transforming complex problems into elegant, scalable solutions. Exploring the endless possibilities of AI and computer vision keeps me excited, whether it's improving model performance or creating innovative tools to make an impact. 📚 Let's Connect: I'm always eager to share ideas, collaborate on projects, and learn from fellow tech enthusiasts! Follow along as I share insights, tutorials, and breakthroughs in the field.