A Four-Level Bias

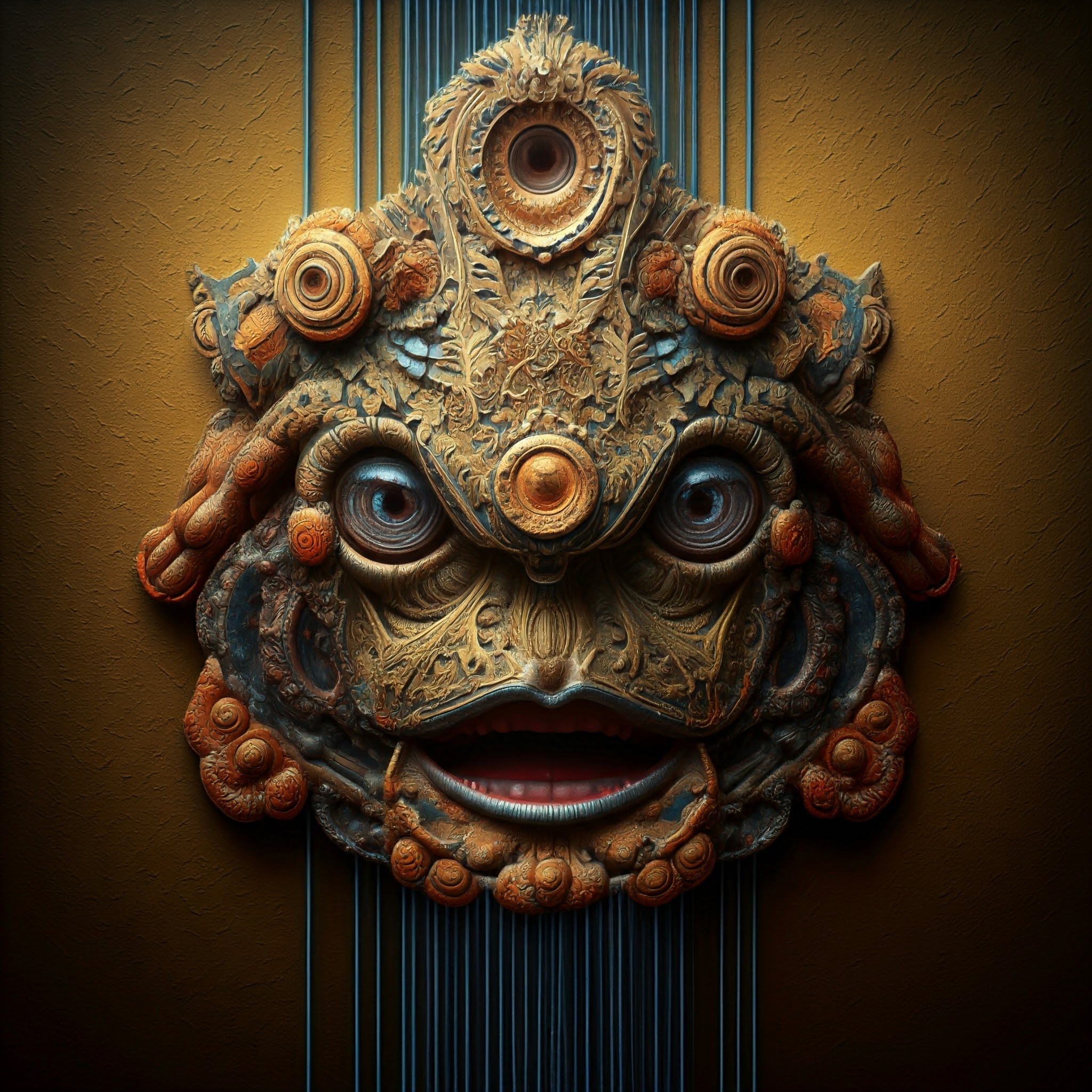
There is a bias against bias.
In many contexts, bias is seen as a negative thing. It's often associated with prejudice, discrimination, and unfairness. There's a strong desire to eliminate bias, particularly in areas like artificial intelligence and decision-making.
However, it's important to recognize that not all bias is harmful. In fact, some biases can be beneficial. For example, a preference for certain types of food or music can enhance our lives.
The key is to identify and mitigate harmful biases, while acknowledging that some biases are inevitable and even desirable.
There is a bias against bias against bias.
This concept might seem a bit convoluted, but it essentially means that sometimes, the strong push to eliminate bias can itself become biased.
Here's a breakdown:
The initial bias: This is the original bias that we want to eliminate. For example, a hiring bias against a certain demographic.
The bias against bias: This is the effort to identify and correct the initial bias. It involves recognizing that biases exist and actively working to mitigate them.
The bias against bias against bias: This is a potential overcorrection where the effort to eliminate bias becomes so strong that it leads to new biases. For example, a company might become so focused on diversity quotas that they prioritize diversity over merit, inadvertently creating a new form of bias.
Theoretically, we could loop the bias against bias infinitely.
However, in practical terms, there's a limit to how far this can go.
The key is to find a balance. While it's important to be aware of biases and strive to eliminate them, it's equally important to avoid becoming overly cautious or rigid in our approach.
It's important to strike a balance between acknowledging and addressing bias, while also avoiding excessive caution that can stifle innovation and creativity.
Let's explore a hypothetical scenario to illustrate a four-level bias loop:
Level 1: Initial Bias
The Bias: A company's historical hiring data might be skewed towards a certain demographic (e.g., mostly male engineers).
The Impact: AI algorithms trained on this biased data may inadvertently favor candidates from similar backgrounds.
Level 2: Bias Against Bias
The Bias: To counteract the initial bias, the company implements measures to diversify its hiring pool and train its AI algorithms on a more balanced dataset.
The Impact: However, this effort might lead to overcorrecting, where candidates from underrepresented groups are favored, even if they are less qualified.
Level 3: Bias Against Bias Against Bias
The Bias: Recognizing the potential for overcorrection, the company adjusts its hiring practices to prioritize merit and qualifications over diversity quotas.
The Impact: This might lead to a backlash from diversity advocates, who argue that the focus on merit alone can perpetuate systemic inequalities.
Level 4: Bias Against Bias Against Bias Against Bias
The Bias: In response to the backlash, the company might implement even stricter diversity quotas, potentially compromising the quality of hires.
The Impact: This could lead to further criticism, as it might be perceived as tokenism or reverse discrimination.
As you can see, the cycle of bias can become complex and multifaceted. It's crucial to strike a balance between addressing historical biases and ensuring fair and equitable hiring practices. By being mindful of potential biases at each level and continuously evaluating our approaches, we can work towards a more just and inclusive future.
Subscribe to my newsletter
Read articles from Grzegorz Herbowicz directly inside your inbox. Subscribe to the newsletter, and don't miss out.
Written by

Grzegorz Herbowicz
Grzegorz Herbowicz
G is a visionary leader in the field of Responsible Artificial Intelligence, driven by a deep commitment to unleashing the benevolent potential of Generative AI, pioneering the responsible development and deployment of AI solutions that are ethical, equitable, and beneficial for all of humanity.