The Impact of GPU Temperatures on Graphics Card Clock Speeds

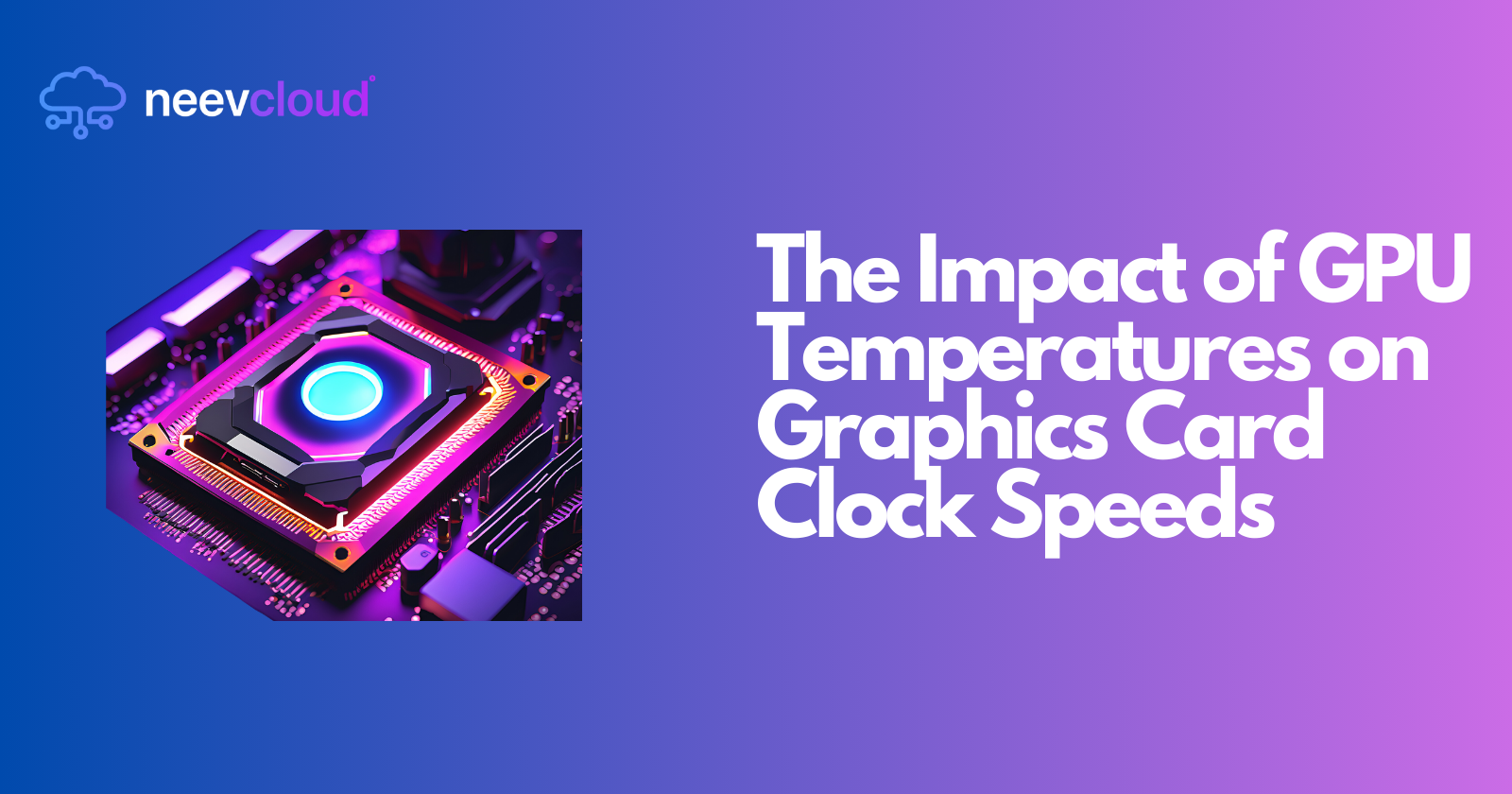
In AI-driven fields, high-performance computing is crucial, especially for tasks like deep learning and data processing in cloud environments. With industry-leading manufacturers like NVIDIA, graphics cards have evolved into powerful devices capable of handling immense computational workloads. However, maintaining optimal GPU temperatures is essential to ensure peak performance, as heat can directly affect clock speeds, and subsequently, the performance of applications in AI cloud and datacenter environments.
In this article, we’ll explore the relationship between GPU temperatures and clock speeds, with a focus on cloud GPUs, AI clouds, and AI datacenters.
Understanding GPU Clock Speed
What is GPU Clock Speed?
- Clock speed refers to the frequency at which a GPU's processor operates, measured in MHz or GHz. Higher clock speeds generally translate to faster processing, critical in real-time rendering, AI tasks, and deep learning computations.
Why is Clock Speed Important for Cloud GPU and AI Cloud Computing?
Faster clock speeds in cloud GPUs mean quicker data processing, which is vital for applications requiring real-time or near real-time responses.
It directly affects AI workloads, where milliseconds count in providing insights or training models.
For AI datacenters, optimizing clock speed across multiple GPUs maximizes throughput and reduces latency for end-users.
Thermal Dynamics: The Role of Temperature in GPU Performance
Temperature and Its Impact on Hardware
Heat is an inherent byproduct of processing power. As a GPU works harder, it generates more heat, which needs to be dissipated to avoid thermal damage and throttling.
In cloud environments, where GPUs are often running 24/7 at high utilization, thermal management becomes critical to avoid damage or reduced lifespan of hardware.
How Temperature Affects Clock Speed
When a GPU’s temperature exceeds a certain threshold, its clock speed will throttle, or reduce, to prevent overheating.
This throttling is an automatic mechanism by which GPUs protect themselves but directly impacts the performance of tasks, especially in an AI cloud or datacenter setting.
Why Managing GPU Temperature is Crucial in AI Cloud and Datacenters
Consistent Performance
AI datacenters and cloud GPUs operate at high loads. Consistently high temperatures can lead to continuous throttling, causing slower processing times and reduced efficiency.
Consistency is key in AI cloud environments where tasks are often parallelized, and fluctuations in one part of the process can impact the entire workflow.
Longevity of Hardware in AI Datacenters
- Overheating not only reduces performance in the short term but also shortens the lifespan of GPUs. This can lead to increased operational costs as cloud providers would need to replace hardware more frequently.
Energy Efficiency Concerns
Running GPUs at high temperatures can increase power consumption as cooling systems work harder. For datacenters, which operate thousands of GPUs, this can significantly impact the facility's energy budget.
Optimized temperature management is essential for the eco-sustainability goals of many AI datacenters and cloud GPU providers.
How NVIDIA Tackles Temperature-Related Clock Speed Throttling
Dynamic Clock Adjustment Technology
NVIDIA GPUs incorporate thermal throttling mechanisms where clock speeds dynamically adjust to prevent temperatures from exceeding safe limits.
This feature is crucial for maintaining stability in long-duration tasks typical of AI cloud and datacenter environments.
Advanced Cooling Solutions in AI Datacenter GPUs
- NVIDIA’s cloud GPUs, such as the A100 and H100, are equipped with high-efficiency cooling solutions tailored for datacenter use. This helps mitigate throttling due to heat by maintaining lower temperatures under sustained workloads.
NVIDIA’s NVLink for Temperature Balancing in Multi-GPU Systems
- NVIDIA’s NVLink technology facilitates faster communication between GPUs and balances thermal loads, which helps to maintain a stable clock speed across multiple units in high-density AI datacenter setups.
Practical Tips to Manage GPU Temperatures in AI Clouds and Cloud GPU Infrastructures
Managing GPU temperatures in large-scale deployments like AI clouds and datacenters is a complex task, requiring attention to both physical infrastructure and operational practices:
Ensure Sufficient Cooling and Airflow
Use efficient cooling systems tailored for high-performance GPUs. Liquid cooling is often recommended in datacenters to maintain optimal temperatures across rows of GPUs.
AI datacenters can benefit from a combination of liquid and air cooling to efficiently manage heat generated from continuous, high-load GPU usage.
Automated Monitoring with AI-Driven Solutions
AI-based monitoring solutions can predict when a GPU is likely to throttle by analyzing usage patterns and temperatures.
This allows for preemptive actions, such as redistributing workloads or adjusting clock speeds before throttling occurs, keeping cloud GPUs running smoothly.
Use of DCGM (NVIDIA Data Center GPU Manager)
NVIDIA’s DCGM provides real-time monitoring of GPU health, including temperature tracking, helping cloud providers proactively manage throttling.
For AI cloud providers, integrating DCGM into their infrastructure can help ensure high GPU performance without the risk of overheating.
Leverage Dynamic Power Management
Adjusting power settings dynamically based on workload can prevent unnecessary heat generation.
AI clouds can employ automated power adjustments to manage GPU power consumption, reducing the risk of overheating without sacrificing clock speed.
Utilize Efficient Data Center Design
AI datacenters optimized for airflow, with efficient hot and cold aisle containment, help keep GPUs within safe operating temperatures.
Strategic rack placements for airflow direction can also mitigate hot spots that could otherwise lead to localized overheating and clock throttling.
Case Study: NVIDIA’s Role in Managing Thermal Efficiency for AI Cloud and Datacenter Applications
NVIDIA’s cloud GPUs are engineered to perform under pressure, handling extensive workloads while maintaining optimal thermal levels. Here’s a breakdown of NVIDIA’s design and cooling technologies specific to cloud and datacenter deployments:
NVIDIA H100’s Heat Dissipation Technology
- The H100, designed for next-gen AI applications, includes advanced cooling mechanisms that reduce the risk of throttling and promote steady clock speeds even under intense workloads.
A100’s Thermal Management in Datacenters
- A100 GPUs are tailored for AI datacenters, providing excellent performance per watt through thermal optimization. This makes them ideal for continuous use in cloud GPU environments without the risk of frequent throttling.
NVSwitch Architecture for Multi-GPU Systems
In multi-GPU AI datacenter configurations, NVIDIA’s NVSwitch technology helps prevent thermal bottlenecks by balancing workloads efficiently across GPUs.
This design is crucial in maintaining consistent clock speeds across GPUs, minimizing latency in high-throughput environments.
Future Directions: NVIDIA and Thermal Innovations in AI Cloud GPUs
The future of AI cloud GPUs lies in better managing thermal dynamics as cloud providers and datacenters push the boundaries of what GPUs can handle.
AI-Enhanced Thermal Prediction Systems
Using AI to predict and manage thermal conditions dynamically can further enhance the performance of cloud GPUs in AI workloads.
Predictive systems may eventually be able to adjust GPU clock speeds preemptively, avoiding any degradation in performance without requiring human intervention.
Solid-State Cooling Innovations
- NVIDIA and other industry leaders are exploring solid-state cooling, which could allow for even more efficient heat management without the complexities of liquid cooling.
New Materials and Cooling Agents
Future AI cloud GPUs may incorporate cutting-edge materials with higher thermal conductivity, allowing them to dissipate heat more efficiently.
Emerging cooling agents that can maintain low temperatures without increasing power consumption could be pivotal in AI datacenter design.
Key Takeaways: Optimizing GPU Performance by Managing Temperatures in AI Cloud and Datacenters
The relationship between GPU temperatures and clock speeds is crucial in ensuring optimal performance for AI cloud applications and large-scale datacenter operations. Here’s a quick summary of how temperature affects GPU clock speed and what measures can be taken:
High temperatures cause GPUs to throttle, reducing clock speeds and, by extension, processing power.
For AI clouds and cloud GPU applications, thermal throttling can lead to delays and inconsistencies, impacting the efficiency and reliability of services.
Proper cooling solutions, AI-based predictive monitoring, and efficient infrastructure design are essential for managing GPU temperatures.
NVIDIA’s innovations in thermal management and dynamic clock adjustment contribute to the steady performance of their GPUs, making them ideal for AI datacenters and cloud environments.
By understanding the intricate balance between GPU temperatures and clock speeds, AI cloud providers and datacenters can ensure optimal performance, manage costs, and extend the longevity of their infrastructure. With continuous advancements from companies like NVIDIA, the future of GPU performance in AI cloud environments is poised for efficiency and sustainability.
Subscribe to my newsletter
Read articles from Tanvi Ausare directly inside your inbox. Subscribe to the newsletter, and don't miss out.
Written by
