AI Is Not a Replacement for Your Brain

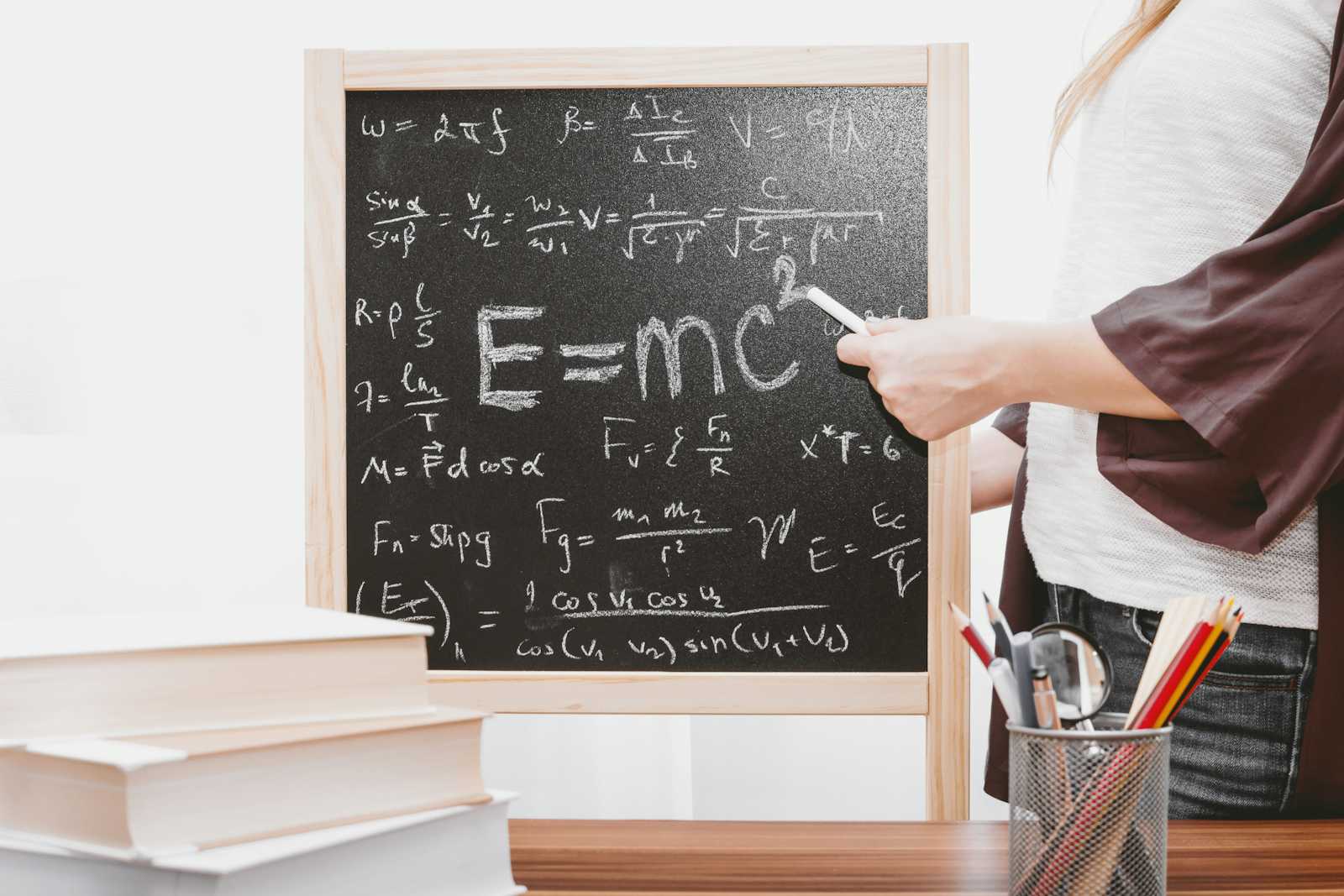
In our rapidly evolving digital landscape, we find ourselves swimming in an ocean of information, much of it served to us instantly by artificial intelligence tools like Large Language Models (LLMs). This unprecedented access has led many to conflate information availability with genuine knowledge—a dangerous misconception that threatens to undermine the very nature of learning and expertise.
Consider this analogy: Having access to every diet plan ever written doesn't automatically make you healthier. Weight loss, despite all available information, still requires dedication, consistent effort, and a clear objective. Similarly, while AI can provide us with vast amounts of information, it cannot do the heavy lifting of learning and understanding for us. The journey from information to knowledge, like the path to physical fitness, demands personal investment and active engagement.
The Information-Knowledge Divide
The distinction between information and knowledge, though subtle, is profound. LLMs like ChatGPT excel at providing quick access to information across various complexity levels. They can spark curiosity and point us toward valuable resources. However, merely having access to this information doesn't confer expertise. True mastery in any field requires deep engagement, questioning assumptions, and—perhaps most importantly—applying knowledge through real-world experience.
This reality check is particularly relevant in our current era, where the temptation to outsource our learning to AI grows stronger by the day. Just as knowing which foods are healthy differs vastly from maintaining a healthy lifestyle, understanding a concept requires more than simply accessing information about it.
Learning as a Journey, Not a Download
The fundamental misconception about AI and learning lies in viewing knowledge as a product rather than a process. Learning is not merely about acquiring information through web searches or chatbot interactions—it's a complex, multi-faceted journey that involves:
Acquisition: Initial exposure to new information and concepts
Understanding: Processing and making sense of new information
Consolidation: Connecting new information with existing knowledge
Adoption: Accepting and internalizing new concepts
Application: Using knowledge in real-world situations
Experimentation: Testing understanding through practical attempts
Failure: Learning from mistakes and misunderstandings
Adaptation: Modifying understanding based on experience
This process cannot be shortcut by AI tools, no matter how sophisticated they become. Each stage requires active engagement and personal investment. When we attempt to bypass these stages by relying solely on AI interactions, we rob ourselves of the deep learning that comes from struggle, reflection, and practical application.
The Irreplaceable Role of Education
Traditional education, despite its critics, remains our most effective framework for building lasting knowledge. Through structured learning, trial and error, and critical thinking, we transform raw information into something more valuable: genuine understanding. Educational processes help us move beyond surface-level facts, encouraging us to connect ideas and build comprehensive mental models.
While LLMs serve as exceptional information delivery systems, they cannot replace the purposeful direction that education provides. Without clear goals and structured learning pathways, even the most sophisticated AI tools can only offer scattered pieces of information, leaving us to navigate without a compass.
The Dangerous Illusion of Instant Expertise
Perhaps the most concerning trend is the growing belief that AI can somehow bestow expertise without requiring effort from the learner. This misconception leads to what we might call "artificial confidence"—a false sense of mastery based on casual exposure to information rather than deep understanding.
Think again about the weight loss analogy: Reading every fitness article online doesn't transform your body; only consistent, focused effort achieves that goal. Similarly, genuine understanding demands active engagement with the material, regular practice, and the gradual building of knowledge upon knowledge.
The E=mc² Illusion
Perhaps no better example exists of the gap between information access and true knowledge than Einstein's famous equation, E=mc². In today's world, anyone can ask a chatbot about special relativity and receive an immediate, apparently comprehensive response. We can even dig deeper, asking follow-up questions and exploring its implications. But this instant access to information creates a dangerous illusion.
Think of it this way: E=mc² sits like a flag atop Mount Everest. AI chatbots can instantly transport us to that summit, showing us the equation and its immediate surroundings. We might even explore the peak a bit, getting various perspectives on what the equation means. But this effortless teleportation doesn't make us mountaineers, just as it doesn't make us physicists.
The journey to truly understanding special relativity took Einstein a lifetime of dedicated work. For physics students today, it requires years of structured learning, building from fundamental principles through increasingly complex mathematical and theoretical frameworks. Just as you can't become physically strong by doing a few repetitions or attending the gym sporadically for a month, you can't develop deep scientific understanding through casual interactions with AI tools.
The Dangers of Artificial Expertise
This illusion of knowledge leads to a particularly concerning phenomenon known as the Dunning-Kruger effect, where individuals overestimate their own knowledge and capabilities. In the age of AI, this effect is amplified by unprecedented access to information, creating what we might call "artificial expertise."
The dangers of this artificial expertise are manifold:
Oversimplification: Complex domains get reduced to seemingly simple concepts, losing crucial nuance and context
Poor Judgment: Overconfidence leads to decision-making without proper consideration of complexities
Missed Details: Critical factors that domain experts learn through years of experience get overlooked
False Equivalence: Quick AI-assisted research gets equated with years of dedicated study and practice
Domain expertise, whether in physics, medicine, engineering, or any other field, is built through years of challenges, failures, and successes. These experiences cannot be shortcut through AI interactions, no matter how sophisticated the tools become.
Finding the Right Balance
AI tools undoubtedly have their place in modern learning. They can serve as valuable guides, offering quick reference points and helping us navigate vast information landscapes. However, we must remember that the transformation of information into knowledge remains a deeply personal journey that requires:
Clear learning objectives
Sustained effort and engagement
Critical thinking and questioning
Practical application of concepts
Regular review and reinforcement
The path from information to knowledge isn't a passive download but an active journey of discovery and understanding. While AI can illuminate the path, we must still walk it ourselves.
Looking Forward
As we continue to integrate AI tools into our learning processes, we must maintain a clear distinction between information access and genuine knowledge. The future of education lies not in replacing human effort with artificial intelligence but in leveraging these tools to enhance our natural learning processes while maintaining the essential elements of true understanding: engagement, practice, and purposeful effort.
Remember: Just as no one can exercise for you, no AI can learn for you. The journey to knowledge, like any meaningful achievement, requires personal investment and dedicated work. Access to information is merely the first step; what you do with that information determines whether it becomes true knowledge.
Subscribe to my newsletter
Read articles from Gerard Sans directly inside your inbox. Subscribe to the newsletter, and don't miss out.
Written by

Gerard Sans
Gerard Sans
I help developers succeed in Artificial Intelligence and Web3; Former AWS Amplify Developer Advocate. I am very excited about the future of the Web and JavaScript. Always happy Computer Science Engineer and humble Google Developer Expert. I love sharing my knowledge by speaking, training and writing about cool technologies. I love running communities and meetups such as Web3 London, GraphQL London, GraphQL San Francisco, mentoring students and giving back to the community.