AI: A Game Changer in Risk Management
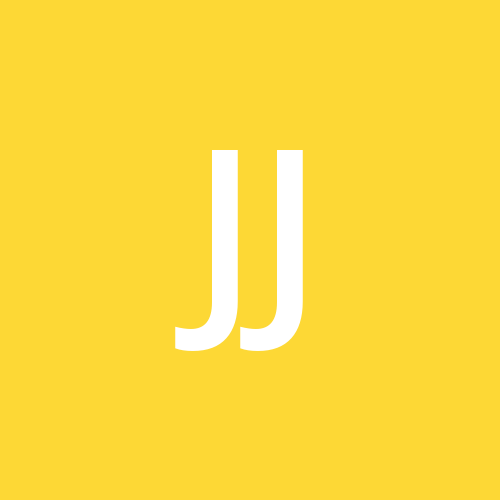
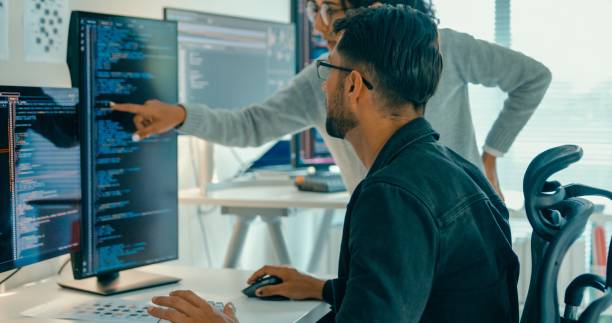
According to a report by McKinsey, AI can reduce risk management costs by 30% to 50% while improving efficiency and accuracy. This staggering statistic underscores AI's transformative impact on the BFSI sector, particularly in risk management. While effective in their time, traditional risk management practices are increasingly becoming outdated in the face of rapid technological advancements. As financial institutions grapple with complex and fast-evolving risk landscapes, AI is a pivotal tool that enhances accuracy, efficiency, and predictive capabilities.
The Evolution of Risk Management:
Risk management has always been fundamental to maintaining financial stability. Historically, it relied heavily on statistical models, expert judgment, and historical data analysis. However, these methods often fell short in predicting black swan events or adapting to the increasing complexity of financial markets. The 2008 financial crisis highlighted the limitations of traditional risk management approaches, leading to a surge in interest in more advanced technologies, including AI.
AI's entry into risk management represents a significant shift. Unlike traditional methods that depend on static models and past data, AI leverages machine learning algorithms, big data analytics, and real-time processing to continuously adapt to new information. This shift not only enhances the ability to predict risks but also allows for more dynamic and proactive risk management strategies.
How AI is Transforming Risk Management:
AI is revolutionising risk management in several key ways. First, it enhances predictive accuracy. Traditional risk models often fail to account for new, unforeseen risks. AI, however, can process vast amounts of data from diverse sources, identifying patterns and correlations that humans might overlook. This capability allows financial institutions to anticipate risks with greater precision.
Second, AI improves efficiency. Risk management processes have traditionally been labor-intensive, requiring significant manual input for data collection, analysis, and reporting. AI automates these tasks, significantly reducing the time and resources needed. For example, AI-driven tools can automatically aggregate data from multiple sources, perform complex analyses, and generate risk reports in real-time. This automation frees up risk managers to focus on more strategic tasks, such as interpreting results and making informed decisions.
Third, AI enhances real-time risk monitoring. In today’s fast-paced financial environment, the ability to monitor and respond to risks in real-time is crucial. AI-powered systems can continuously analyse data streams, flagging potential risks as they emerge. This real-time capability is particularly valuable in areas like market risk, where conditions can change rapidly.
Key AI Technologies in Risk Management:
Several AI technologies are particularly impactful in transforming risk management:
Machine Learning (ML): ML algorithms can identify patterns and correlations in large datasets that are not immediately apparent to humans. These insights enable more accurate risk predictions and the development of adaptive risk models.
Natural Language Processing (NLP): NLP allows AI systems to analyse unstructured data, such as news articles, social media posts, and regulatory updates. By processing this information, AI can assess risks that may not be captured by traditional data sources.
Predictive Analytics: Predictive analytics, powered by AI, enables institutions to forecast potential risks based on historical data and current trends. This proactive approach allows for the mitigation of risks before they materialise.
Robotic Process Automation (RPA): RPA can automate repetitive, rule-based tasks in risk management, such as data entry and compliance checks. This automation reduces the likelihood of human error and speeds up processes.
Challenges and Considerations:
While AI offers significant benefits, its implementation in risk management is not without challenges. One of the primary concerns is the "black box" nature of many AI models. These models, while highly accurate, often lack transparency, making it difficult for risk managers to understand how decisions are made. This opacity can be problematic, particularly in regulatory environments where transparency and explainability are crucial.
Another challenge is data quality. AI systems rely heavily on large volumes of high-quality data. Inaccurate or incomplete data can lead to flawed risk assessments. Financial institutions must ensure robust data governance practices to support AI-driven risk management.
Finally, there is the issue of ethical considerations. The use of AI in risk management raises questions about fairness, bias, and accountability. Financial institutions must navigate these ethical challenges carefully to maintain trust and compliance.
Conclusion:
AI is undoubtedly a game changer in risk management, offering unprecedented levels of accuracy, efficiency, and real-time monitoring. As financial institutions continue to adopt AI technologies, they will need to address the associated challenges, including model transparency, data quality, and ethical considerations. By doing so, they can fully harness the transformative potential of AI, creating more resilient and proactive risk management frameworks that can adapt to the ever-evolving financial landscape.
Subscribe to my newsletter
Read articles from Jennifer Jose directly inside your inbox. Subscribe to the newsletter, and don't miss out.
Written by
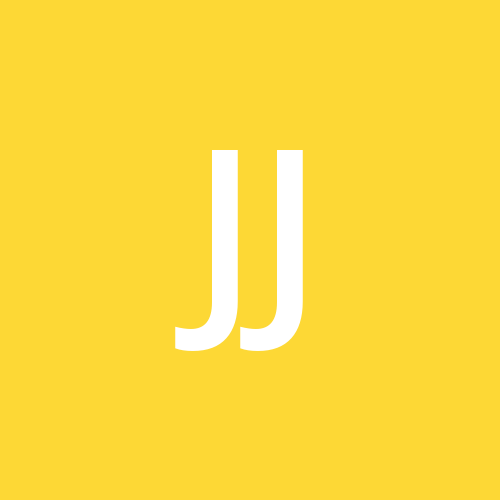