Why Human-Assisted Data Collection Is a Must for AI/ML Training

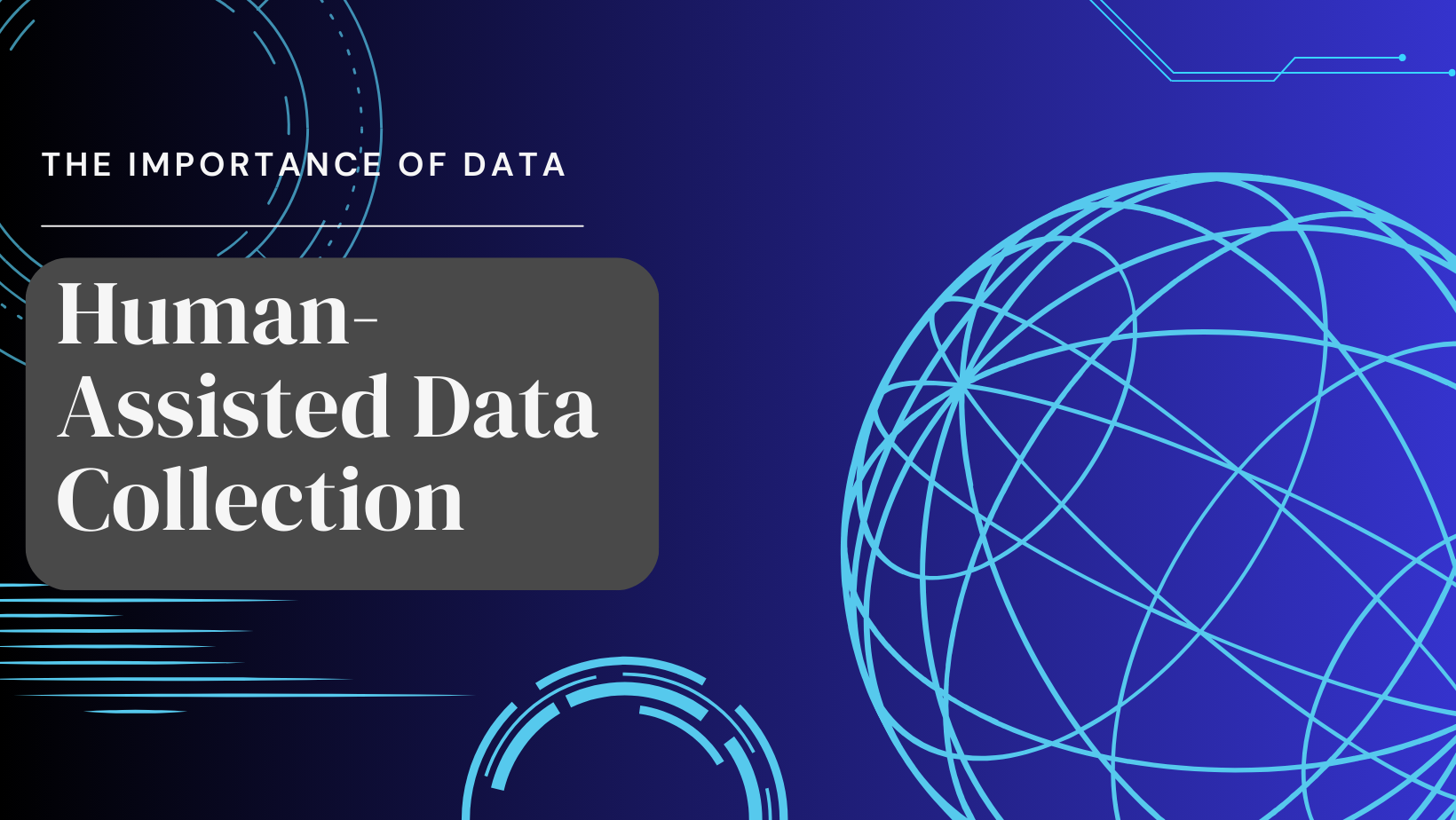
Despite the rapid advancement of AI and ML technologies, organizations are hitting significant roadblocks due to data quality challenges. Even with robust AI programs, 6% of global annual revenue is lost due to underperforming models. This poor performance is primarily due to low-quality and incorrect data that was collected and labeled during the AI/ML model training.
Companies that use automated systems can efficiently gather and label data at scale, but they often struggle with accuracy, completeness, and bias, especially when dealing with complex or unstructured data. This increases the need to involve human assistants in overcoming these challenges.
To get a more reliable AI/ML model, organizations are improving their data collection process and opting for a human-in-the-loop approach rather than a fully automated process. This blog will shed some light on the importance of the human-assisted data collection process for training AI/ML models.
Why Human Assistance Is Crucial in AI-Powered Data Collection
AI-powered data collection uses AI technologies to automate and enhance the gathering of data from various sources. This approach ensures faster processing and reduces manual labor, but it still often requires human oversight for specific tasks. Below are the reasons why including human assistance in the data collection process can help in accurate data collection for AI/ML training:
1. Ensures High Data Quality
Human intervention plays a crucial role in collecting and validating data for AI/ML training. While automated processes are fast, they can lead to errors, especially with unstructured or noisy data. Human oversight ensures errors are corrected promptly, minimizing inaccuracies in datasets to maintain high data quality for AI/ML model training. For example, in medical imaging, radiologists validate the dataset of scans to catch misclassifications, ensuring the model learns from accurate data and delivers reliable results.
2. Adds Context and Nuance
AI algorithms can miss subtle meanings that are critical for real-world applications. Humans understand the context and interpret it accurately, ensuring this is reflected in the training datasets so that the AI models function as desired. They help collect datasets that align contextually with the AI model's idea to improve the model's efficiency. This is particularly important for applications in healthcare, legal sectors, or customer service, where contextual accuracy plays a vital role.
3. Reduces Bias and Improves Fairness
Automated systems, without human oversight, may perpetuate biases. Human involvement in data collection and labeling helps to gather accurate datasets to reflect diverse, inclusive, and fair representations, thereby ensuring bias reduction in AI training. This proactive intervention ensures that AI models promote ethical outcomes and avoid unintended discrimination, especially in high-impact areas like hiring, lending, and law enforcement.
4. Identifies and Handles Edge Cases
Edge cases—uncommon or unexpected situations—pose a significant challenge for AI models, as they are often underrepresented in datasets. Human intervention ensures these rare instances are correctly identified, collected, and labeled, allowing AI models to generalize better across diverse scenarios. For example, in autonomous driving, unexpected road conditions (e.g., animals crossing highways) must be included in the data fed to the systems. Without human oversight, AI systems might fail to respond adequately in critical situations, compromising reliability.
5. Addresses Data Gaps and Errors in Real-Time
Data gaps and errors are the primary errors found when data is collected with automated tools. Many tools cannot validate the data collected from different sources. The human-in-the-loop approach in data collection and management allows immediate validation and correction, preventing these gaps in the collected and labeled data from compromising the model's output. For example, supply chain management systems rely on updated data to predict inventory needs and prevent stockouts. Missing or delayed data—like inaccurate shipment statuses or stock level—can lead to inventory shortages or overstock, negatively impacting operations and sales.
6. Supports Adaptability and Continuous Learning
AI models must adapt to changing patterns and evolving contexts, such as shifting consumer behavior or regulatory changes. Human intervention helps collect updated and refined data continuously, ensuring that AI models stay relevant and effective over time. By monitoring trends and providing new data to the AI systems, humans enable incremental learning that aligns AI systems with real-world changes.
7. Ensures Ethical Compliance and Data Privacy
With increasing concerns about data privacy and ethical AI, human oversight is essential to ensure compliance with regulatory frameworks like GDPR and CCPA. Humans help in monitoring the ethical collection and usage of data, especially when dealing with sensitive information, ensuring that AI systems are developed and deployed responsibly. This supervision mitigates risks associated with data misuse and fosters trust in AI-driven processes.
8. Minimizes Errors in Datasets for Data Annotation
AI-powered data collection can introduce errors, such as mislabeling, duplication, or misclassification, which can compromise the accuracy of datasets and disrupt the data annotation process. Since annotated data serves as the foundation for training reliable AI/ML models, any inaccuracies in the raw data can propagate through the annotation stage, leading to suboptimal model performance. Human intervention ensures that collected data is thoroughly reviewed and corrected before it enters the annotation pipeline, preserving dataset quality. Accurate data annotation services are critical for ensuring the AI model’s precision and reliability, and without human oversight, flawed data could result in poor predictions, reduced accuracy, and model failures.
What’s Next
AI and ML models rely on high-quality data, but automated processes often fall short in ensuring accuracy and fairness, requiring human intervention. To achieve this, organizations can either build in-house teams or outsource to data collection service providers. In-house teams offer control but demand significant time, resources, and management. Outsourcing, on the other hand, provides scalable, efficient services, leveraging external expertise and tools to streamline data collection while meeting compliance and quality standards, making it a practical option for many businesses.
In conclusion, the human-in-the-loop approach to data collection remains indispensable, whether through in-house teams or outsourcing. Organizations must weigh these pros and cons carefully to ensure their AI/ML models are trained on high-quality, reliable datasets, setting the foundation for better performance, fairness, and ethical compliance in real-world applications.
Subscribe to my newsletter
Read articles from Alvaro Dee directly inside your inbox. Subscribe to the newsletter, and don't miss out.
Written by

Alvaro Dee
Alvaro Dee
Alvaro Dee is a Data Analyst at SunTec Data- a global outsourcing company that specializes in data management and support services. With over five years of experience in his field, Dee has developed a strong understanding of related areas such as database management, data cleaning, data visualization, data mining, research, and annotation.