Unlocking Customer Retention: The Power of Churn Prediction Models

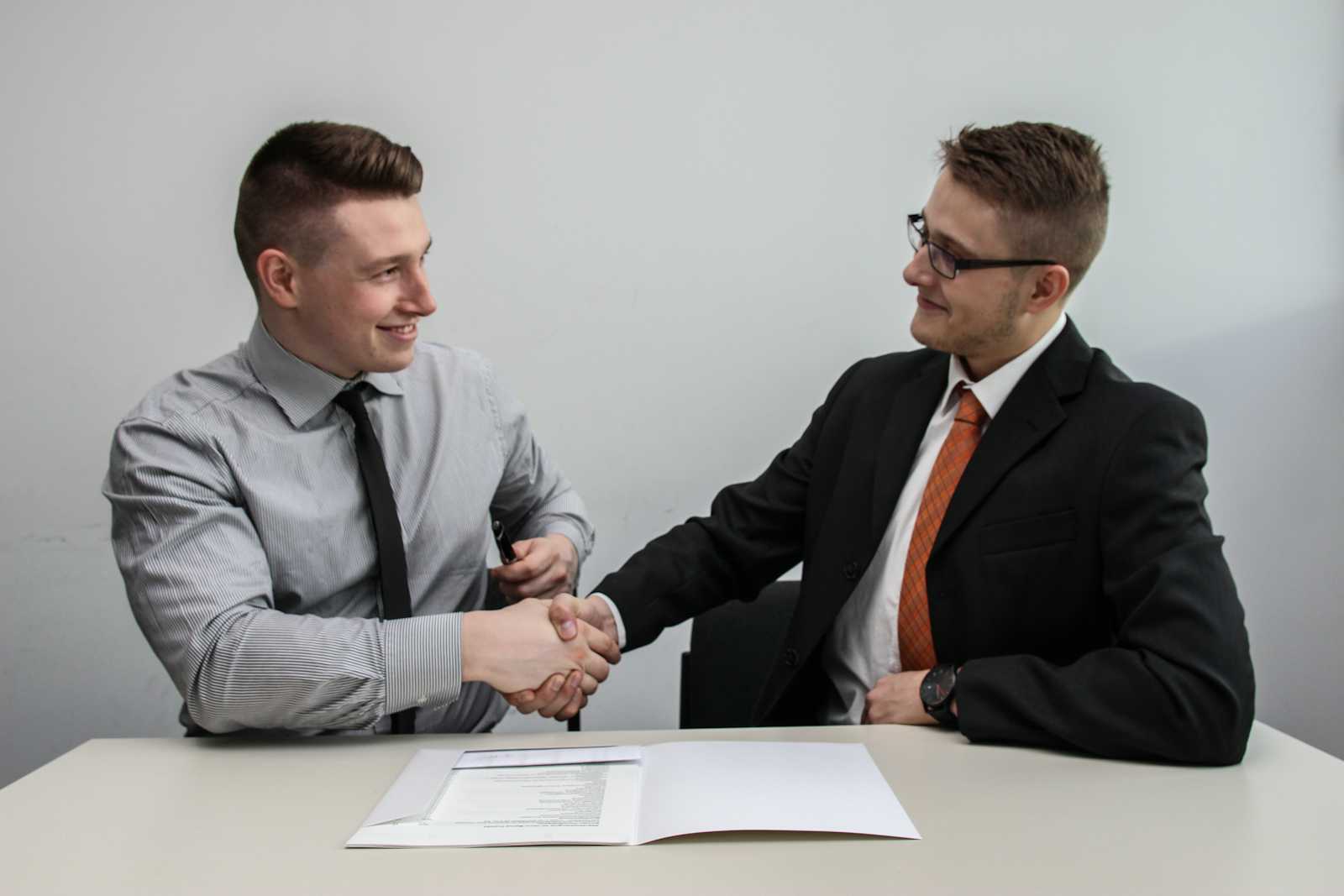
In today's hyper-competitive business landscape, customer retention is a critical factor for sustained growth and profitability. While acquiring new customers is essential, keeping existing ones is often more cost-effective and beneficial in the long run. One powerful tool that businesses are leveraging to enhance customer retention is churn prediction models. By analyzing a company's data through sophisticated algorithms, these models can predict which customers are at risk of leaving, allowing businesses to take proactive measures to retain them.
Understanding Customer Churn
What Is Customer Churn?
Customer churn refers to the loss of customers or clients over a given period. It's a vital metric because it directly impacts revenue and growth. Churn can occur in various forms:
Voluntary Churn: When customers choose to leave due to dissatisfaction, better offers from competitors, or changing needs.
Involuntary Churn: When customers leave due to factors beyond their control, such as payment failures or service terminations.
Why Do Customers Churn?
Understanding the reasons behind customer churn is essential for developing effective retention strategies. Common factors include:
Poor Customer Service: Negative experiences with support can drive customers away.
Lack of Engagement: If customers don't feel valued or connected to the brand, they're more likely to leave.
Competitive Offers: Better pricing or features from competitors can lure customers away.
Product Issues: Bugs, lack of features, or poor performance can lead to dissatisfaction.
The Cost of Customer Churn
Financial Impact
Customer churn can significantly affect a company's bottom line. The cost of acquiring a new customer can be five times higher than retaining an existing one. Moreover, lost customers mean lost revenue and potential negative word-of-mouth.
Brand Reputation
High churn rates can damage a company's reputation, making it harder to attract new customers. In today's digital age, dissatisfied customers can quickly spread their experiences online.
Competitive Disadvantage
Losing customers to competitors not only reduces your market share but also strengthens your competitors' positions.
Churn Prediction Models: A Strategic Solution
What Is a Churn Prediction Model?
A churn prediction model uses data analytics and machine learning algorithms to identify patterns and signals that indicate a customer is likely to leave. By analyzing historical data, the model learns which factors contribute to churn and applies this knowledge to predict future behavior.
How Do Churn Prediction Models Work?
Data Collection: Gathering data from various sources such as transaction histories, customer interactions, and demographic information.
Data Processing: Cleaning and organizing data to ensure accuracy.
Feature Selection: Identifying key variables that influence churn.
Model Training: Using machine learning algorithms to train the model on historical data.
Prediction: Applying the model to current data to predict which customers are at risk.
Actionable Insights: Providing insights that can inform retention strategies.
Benefits of Using Churn Prediction Models
Proactive Retention Strategies
By identifying at-risk customers early, businesses can intervene with targeted actions such as personalized offers, improved customer service, or engagement initiatives.
Personalized Marketing
Churn prediction models enable businesses to tailor their marketing efforts based on individual customer behavior, increasing the effectiveness of campaigns.
Improved Customer Lifetime Value
Retaining customers longer increases their lifetime value, leading to higher revenue and profitability.
Efficient Resource Allocation
Focusing efforts on customers who are more likely to churn allows for better use of resources, reducing wasted time and costs.
Implementing Churn Prediction Models
Data Collection and Preparation
Successful churn prediction relies on high-quality data. This includes:
Transactional Data: Purchase history, frequency, and value.
Behavioral Data: Website visits, app usage, and engagement metrics.
Demographic Data: Age, location, and other personal attributes.
Feedback Data: Customer satisfaction scores, reviews, and complaints.
Choosing the Right Model
Selecting the appropriate machine learning algorithm is crucial. Common models include logistic regression, decision trees, random forests, and neural networks. The choice depends on the complexity of the data and the specific needs of the business.
Integration into Business Processes
For maximum effectiveness, the churn prediction model should be integrated into existing customer relationship management (CRM) systems and workflows. This ensures that insights are accessible and actionable by the relevant teams.
Best Practices for Churn Prediction
Regular Model Updates
Customer behavior and market conditions change over time. Regularly updating the model with new data ensures it remains accurate and effective.
Cross-Functional Collaboration
Involving teams from marketing, sales, customer service, and IT can enhance the model's effectiveness by providing diverse insights and expertise.
Ethical Considerations
Respecting customer privacy and adhering to data protection regulations like GDPR and CCPA is essential when handling personal data.
Case Study: Transforming Retention with Churn Prediction
Consider a subscription-based streaming service facing high churn rates. By implementing a churn prediction model, they identified that users who didn't create personalized playlists were more likely to leave. The company launched a campaign encouraging playlist creation and provided recommendations. As a result, they saw a 15% reduction in churn over six months.
Challenges and Considerations
Data Privacy and Compliance
Handling customer data comes with responsibilities. Compliance with regulations is essential to protect customer privacy and maintain trust.
Model Accuracy and Maintenance
Churn prediction models require continuous monitoring and updating to maintain accuracy. As market conditions and customer behaviors change, the model must adapt accordingly.
Resource Investment
Developing and maintaining a churn prediction model requires investment in technology and expertise. However, the long-term benefits often outweigh the initial costs.
The Future of Churn Prediction
Advancements in artificial intelligence and machine learning are continually enhancing the capabilities of churn prediction models. Features like real-time analytics and more sophisticated algorithms offer even greater precision in identifying at-risk customers.
Integration with AI and Machine Learning
Future models will leverage deep learning and AI to handle more complex data sets and uncover hidden patterns that traditional models might miss.
Real-Time Analytics
Implementing real-time data processing allows businesses to react instantly to customer behavior changes, further reducing the likelihood of churn.
Conclusion
Customer churn poses a significant challenge, but with the right tools and strategies, it can be effectively managed. Churn prediction models offer a powerful means to anticipate customer behavior and implement proactive retention measures. By leveraging your company's data through these models, you can enhance customer satisfaction, increase loyalty, and drive sustainable growth.
Implementing a churn prediction model involves careful planning, quality data collection, and ongoing maintenance. However, the rewards—improved customer retention, increased revenue, and a stronger competitive position—make it a worthwhile investment for any business looking to thrive in today's market.
Empower your business with data-driven insights and take the first step towards a more profitable future by exploring the potential of churn prediction models.
Subscribe to my newsletter
Read articles from Nathan Ayers directly inside your inbox. Subscribe to the newsletter, and don't miss out.
Written by
