AI Ethics: Why AWS Machine Learning Certification Emphasizes Ethical AI?

Table of contents
- Building Trust in AI with Responsible Data Handling
- Fairness and Bias in AI: Reducing Discrimination in ML Models
- Accountability: Building Transparent and Traceable AI Systems
- Legal Compliance: Keeping Up with Evolving Regulations
- Practical Tools and Real-World Applications: Bridging Theory and Practice
- Preparation Guide for AWS Certified Machine Learning - Specialty Certification
- Why AWS Certification is Essential for Ethical AI?
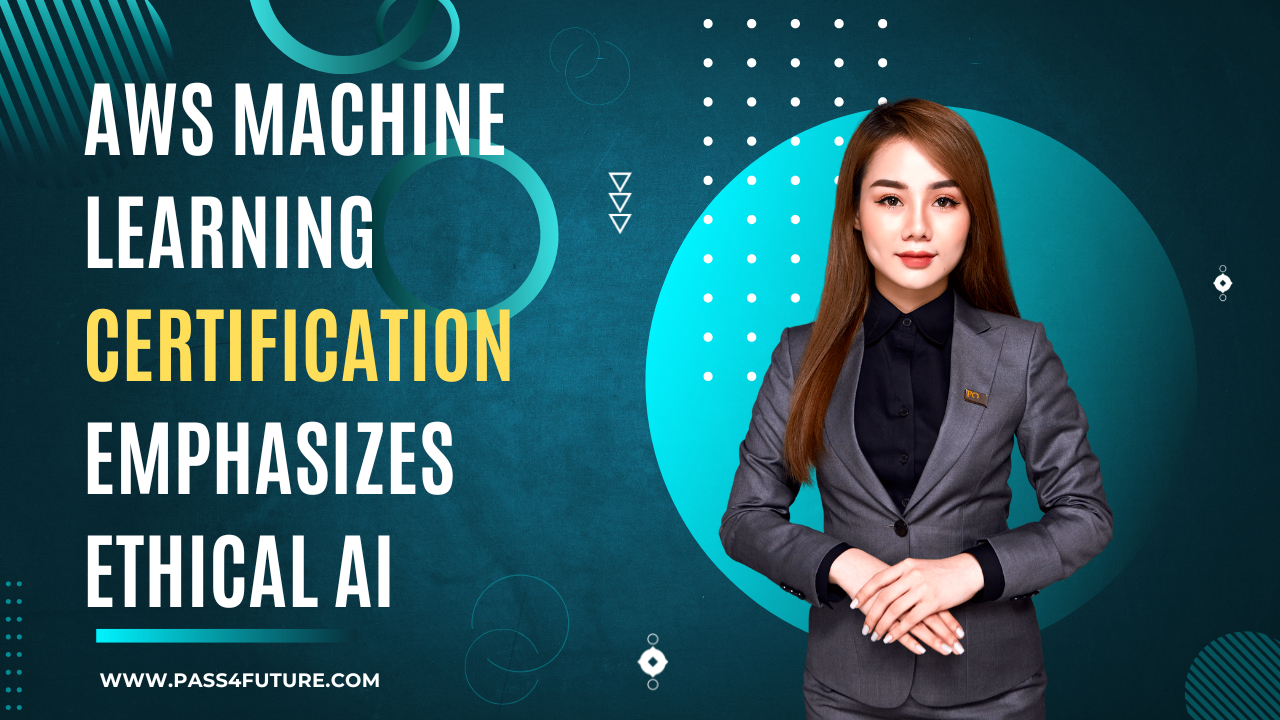
With the rapid rise of AI technologies, ethical considerations in machine learning have never been more critical. The AWS Certified Machine Learning - Specialty certification recognizes this need and emphasizes ethical AI as a core component of its curriculum. For AWS, AI ethics isn’t just a buzzword; it’s an essential framework that guides how machine learning (ML) should be responsibly developed and deployed, ensuring trust, fairness, and accountability in every AI-driven outcome.
Let’s take a closer look at why ethical AI is a significant part of AWS's certification, how AWS professionals are trained to uphold ethical standards, and what this means for anyone looking to pursue the certification.
Building Trust in AI with Responsible Data Handling
In any ML project, the data being used holds immense power and responsibility. Certified AWS professionals are trained to recognize the importance of responsible data collection, ensuring data privacy and consent. Through AWS tools like AWS Sagemaker and AWS Identity and Access Management (IAM), certified professionals learn how to keep user data secure and maintain compliance with privacy regulations like GDPR.
Responsible data handling ensures that models are transparent, trustworthy, and safe for users. By addressing issues of data privacy and consent, AWS helps prevent data misuse that could harm users and tarnish public trust in AI applications.
Next up section will address another key area: ensuring fairness and reducing bias in AI models, an essential concern for anyone working with ML.
Fairness and Bias in AI: Reducing Discrimination in ML Models
One of the biggest challenges in machine learning is ensuring that models are fair and unbiased. Without proper training, an ML model could unintentionally perpetuate discrimination based on race, gender, or other sensitive attributes. AWS addresses this by emphasizing fairness and bias mitigation in its certification training.
AWS-certified professionals are taught techniques to detect and reduce bias, such as re-sampling data, feature selection, and analyzing model outputs for unintended impacts. AWS tools like SageMaker Clarify allow developers to measure and monitor fairness within their models, ensuring outcomes are as equitable as possible. AWS certification equips ML professionals with strategies to build fair, unbiased models, promoting trust and inclusivity in their applications.
Next up section will explore why accountability is key to building transparent AI systems that businesses and users can rely on.
Accountability: Building Transparent and Traceable AI Systems
Accountability is a crucial element of ethical AI. AWS-certified professionals are trained to make AI systems traceable, meaning every decision and output can be tracked and explained. This transparency is vital for industries like healthcare and finance, where AI decisions can have profound impacts on people’s lives.
AWS provides tools and techniques that help professionals create audit trails for their ML workflows. For example, Amazon CloudWatch and AWS CloudTrail allow monitoring of all activities, making it easy to trace back and verify every step in an ML model’s lifecycle. Certification training reinforces the importance of transparency, teaching professionals how to document models and communicate AI decisions to stakeholders, which builds credibility and confidence in AI applications.
Next up section about understanding legal and ethical compliance is vital for any ML professional, especially as regulatory frameworks evolve globally.
Legal Compliance: Keeping Up with Evolving Regulations
Laws and regulations surrounding AI ethics are constantly changing, especially with the global push toward responsible AI practices. The AWS Machine Learning certification teaches professionals about these evolving standards and compliance requirements. AWS ensures that certified ML practitioners understand and adhere to frameworks like GDPR and CCPA, ensuring their models and practices meet legal standards.
Certified professionals learn to work within regulatory boundaries, making sure that all data handling, storage, and processing align with privacy laws. This focus on compliance helps businesses avoid legal risks while ensuring that their AI initiatives remain ethical and respectful of user rights.
Next up section will summarize how certification provides professionals with the practical tools and knowledge to apply ethical principles in real-world scenarios.
Practical Tools and Real-World Applications: Bridging Theory and Practice
Beyond theoretical knowledge, the AWS Machine Learning certification provides practical experience with AWS tools like SageMaker, Rekognition, and Amazon Translate. These tools include ethical AI features, empowering professionals to integrate ethical standards into every phase of their ML projects, from data processing to model deployment.
This hands-on training ensures that AWS-certified ML professionals are prepared to address ethical challenges in real-world settings, making them not just skilled technologists but also responsible stewards of AI innovation.
Preparation Guide for AWS Certified Machine Learning - Specialty Certification
Here’s a streamlined approach to preparing for the AWS Certified Machine Learning - Specialty exam:
Understand the Exam Structure
The exam covers four main domains:
Data Engineering (20%): Data ingestion, transformation, and storage.
Exploratory Data Analysis (24%): Data visualization and distribution analysis.
Modeling (36%): Model selection, training, and tuning.
Machine Learning Implementation and Operations (20%): Model deployment and optimization.
Focus on domains with more weight, such as Modeling, to maximize your preparation efforts.
Gather Study Resources
AWS Training: Use AWS’s "Machine Learning Pathway" and "Exam Readiness" courses.
Books: "AWS Certified Machine Learning Specialty Study Guide" and "Machine Learning with AWS" are helpful.
Practice Exams: Take Pass4future AWS Certified Machine Learning Certification exam questions and try additional practice tests from platforms like Study4exam or A Cloud Guru.
Gain Hands-On Experience
Hands-on practice with AWS tools is essential. Focus on:
Amazon SageMaker: Train and deploy models.
AWS Glue and Lambda: Practice data transformation and serverless compute.
SageMaker Clarify: Detect and mitigate bias, a key component of ethical AI.
Use the AWS Free Tier for practical projects that reinforce learning and ethical AI applications.
Study AI Ethics and Bias Mitigation
Understanding AI ethics is critical for the exam. Use resources like:
AWS Documentation: Read AWS whitepapers on ML ethics.
SageMaker Clarify: Practice tools for bias detection and explainability.
Why AWS Certification is Essential for Ethical AI?
AWS Certified Machine Learning certification isn’t just about ML tools and techniques; it’s a pathway to becoming a responsible AI practitioner. With a strong focus on ethical AI, the certification ensures that professionals are not only technically proficient but also mindful of the ethical implications of their work. From building fair models to ensuring transparency, the AWS ML certification shapes a new generation of AI professionals who are equipped to make ethical choices in every project they undertake.
So, whether you’re an aspiring data scientist, an AI engineer, or a seasoned ML practitioner, AWS’s emphasis on ethical AI prepares you to lead responsibly in the fast-evolving world of artificial intelligence.
Subscribe to my newsletter
Read articles from Emma Nancy directly inside your inbox. Subscribe to the newsletter, and don't miss out.
Written by
