Unraveling Sentiment Analysis with the IFDHN Model: A Game-Changer for Arabic Sentiment Detection
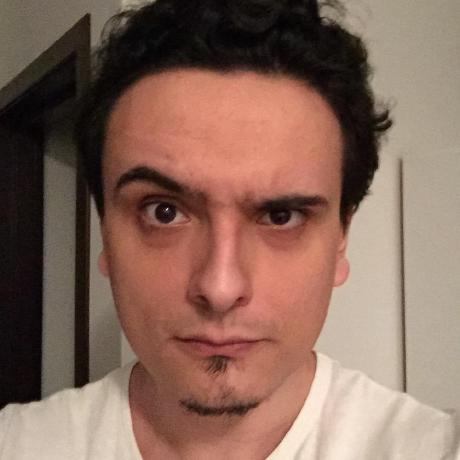

Authors: Yuke Mei, Nan Yan, Shuhao Guan, Cheng Xu, Yang Fang
Published: 2024-11-15
Sentiment analysis has emerged as a pivotal task, enabling computers to understand and interpret the emotions and opinions embedded within large volumes of text. Sentiment analysis is particularly challenging for the Arabic language due to its complex structure and diverse dialects. The recently proposed Improved Fuzzy Deep Hybrid Network (IFDHN) model and the ArSen dataset represent a significant leap forward in this quest, offering new tools and methods to tackle the nuances of Arabic sentiment analysis. Here, we delve into the key findings and implications of this research.
Key Claims of the Paper
The paper introduces two significant contributions to Arabic Sentiment Analysis (ASA): the ArSen dataset and the Improved Fuzzy Deep Hybrid Network (IFDHN) model. The ArSen dataset is meticulously annotated, focusing on sentiments expressed during the COVID-19 pandemic. It serves as a robust benchmark for evaluating sentiment analysis models. On the other hand, the IFDHN model is a novel approach leveraging fuzzy logic to enhance sentiment classification, offering superior performance with much less computational expense compared to existing large models like LLaMA-3.
Proposed Enhancements: Diving into IFDHN
The IFDHN Model
The IFDHN model is designed with two primary channels: Tweet Text and Numerical Context. It employs a combination of CNN (Convolutional Neural Network) and Bi-LSTM (Bidirectional Long Short-Term Memory) layers to process these inputs before applying fuzzy logic. This layered approach allows the model to capture more detailed sentiments beyond what simple text analysis can achieve.
Fuzzy Logic Integration
Fuzzy logic enables the IFDHN model to handle the ambiguity inherent in human language more effectively. By integrating fuzzy logic, the model is better equipped to process nuanced sentiments, leading to more accurate classifications.
Application Potential: How Companies Can Benefit
Businesses aiming to leverage sentiment analysis can harness the capabilities of the IFDHN model in several innovative ways:
Customer Feedback Analysis: By analyzing customer reviews and feedback across social media, companies can gain insights into customer satisfaction, potential areas of improvement, and emerging trends in consumer behavior.
Market Sentiment Analysis: Financial services can use the model to assess market sentiment regarding stocks or economic conditions based on news and social media data.
Targeted Marketing Campaigns: Marketing professionals can design more effective campaigns by understanding the prevailing sentiments around certain products or brands.
Crisis Management: During events like the COVID-19 pandemic, understanding public sentiment can help organizations tailor their responses and communications effectively.
Technical Insights: Training, Hyperparameters, and Hardware Requirements
Hyperparameters and Training
The IFDHN model features a configuration that includes a TextCNN with parallel CNN layers of varying kernel sizes (3, 4, and 5). It utilizes an embedding dimension of 128 and applies a dropout rate of 0.5 to mitigate overfitting. The model's training is efficiently structured to minimize resource usage.
Hardware Specifications
The implementation was tested using a NVIDIA RTX 4090 GPU, demonstrating its compatibility with modern high-performance computing environments. For smaller scale operations, the model's efficient design allows it to run on less powerful hardware without significant drops in performance.
Target Tasks and Datasets
The primary dataset utilized is the ArSen dataset, which contains tweets from the COVID-19 pandemic period. This dataset was developed to capture a diverse range of sentiments and topics related to the pandemic, providing a contemporary and relevant benchmark for Arabic sentiment analysis.
State-of-the-Art Comparison: Standing Tall Among Giants
When compared to other state-of-the-art models like RoBERTa and AraT5-Tweet-Base, the IFDHN model exhibits superior performance across multiple metrics, including accuracy and micro F1 scores. This model achieves comparable performance to sophisticated models such as LLaMA-3-8B, but demands only a fraction of the computational resources, positioning it as an ideal choice for practical applications.
Conclusion: A New Era for Arabic Sentiment Analysis
The unveiling of the IFDHN model marks a significant advancement in the field of sentiment analysis for the Arabic language. By offering both a powerful model and a high-quality dataset, this research paves the way for improved sentiment detection capabilities. Companies across various sectors can harness these tools to derive actionable insights, optimize their operations, and better understand their stakeholders, ultimately unlocking new revenue streams and efficiencies. As sentiment analysis continues to evolve, tools like IFDHN will undoubtedly play a critical role in delivering nuanced and accurate interpretations of human emotions in text.
Subscribe to my newsletter
Read articles from Gabi Dobocan directly inside your inbox. Subscribe to the newsletter, and don't miss out.
Written by
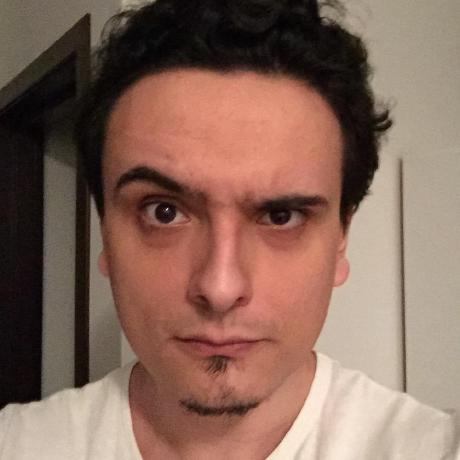
Gabi Dobocan
Gabi Dobocan
Coder, Founder, Builder. Angelpad & Techstars Alumnus. Forbes 30 Under 30.