Exploring the Cutting Edge of Local-Global Attention Mechanisms: Unlocking Potential for Businesses
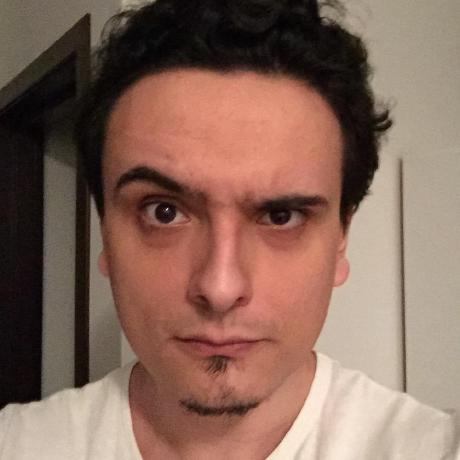
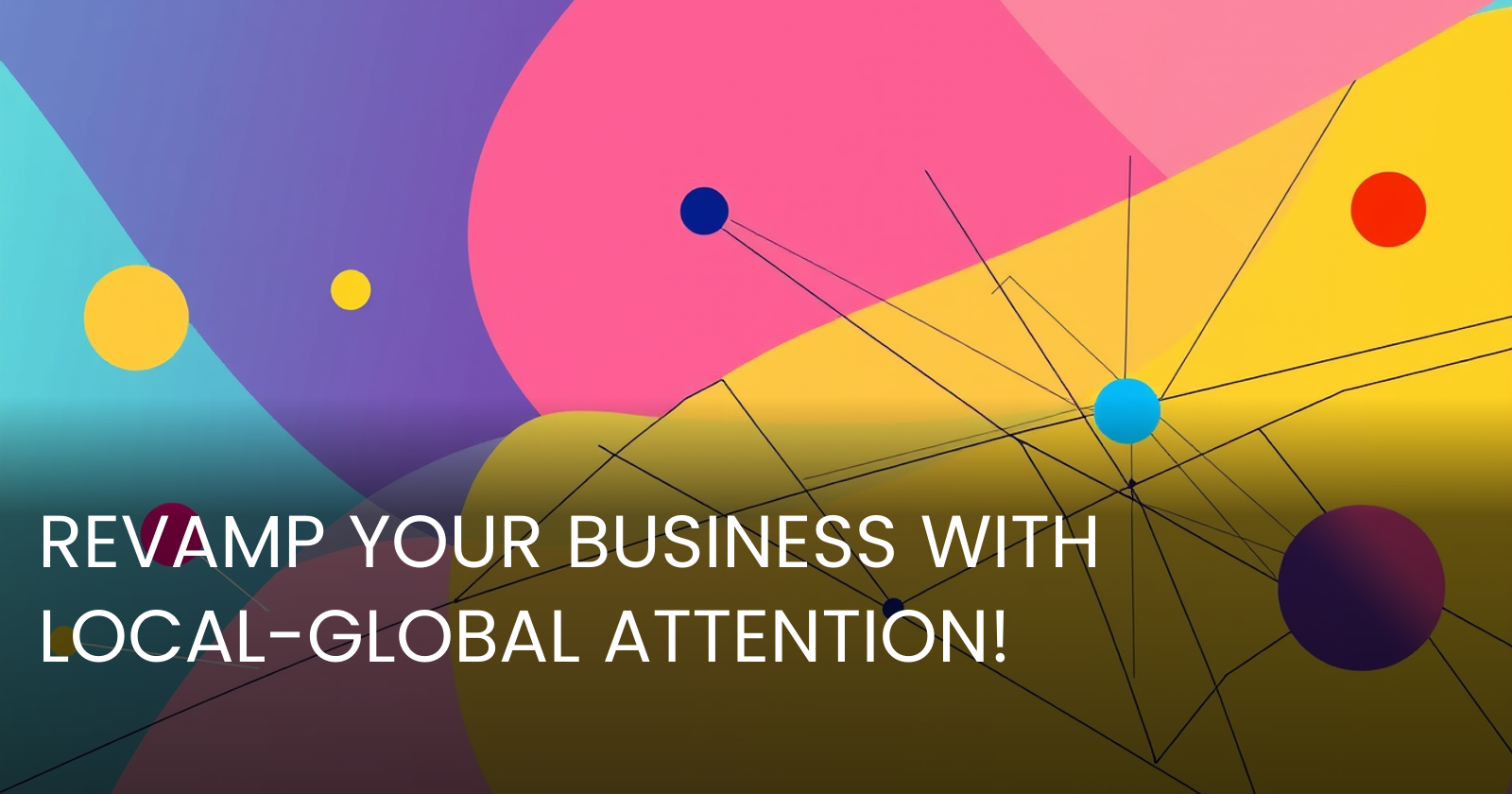
Authors: Yifan Shao
Published: 2024-11-14
Attention mechanisms have become pivotal in enhancing model performance, particularly in the realms of object detection and image classification. A recent scientific paper introduced a novel method known as Local-Global Attention (LGA), which aims to bridge the gap between capturing detailed local features and understanding broad, global contexts of data inputs. This comprehensive analysis intends to distill the technical innovations of this paper into accessible insights and explore its potential applicability in real-world business scenarios.
Main Claims of the Paper
The primary claim of this research is that the newly proposed Local-Global Attention mechanism significantly outperforms existing attention methods by effectively balancing local detail and global context. This innovation enables models to achieve superior accuracy, especially in complex scenarios like small object detection. The paper also emphasizes that this mechanism achieves improved performance without compromising computational efficiency, making it an attractive solution for resource-limited environments.
Novel Proposals and Enhancements
Local-Global Attention introduces a dual approach that integrates multi-scale convolutions with positional encoding. By incorporating learnable parameters (alpha parameters), LGA dynamically adjusts the emphasis on local vs. global features based on task requirements. This flexibility allows for optimized feature representation across different scales, enhancing object and image detection capabilities.
Business Applicability
Leveraging LGA for Innovation
Businesses across various sectors can leverage Local-Global Attention for advanced image processing and analytics applications. The potential use cases include:
Retail and E-commerce: Enhanced product categorization and automated inventory management through precise image recognition can streamline operations and reduce errors.
Healthcare: Improved diagnostic tools via better image analysis can lead to faster and more accurate diagnoses, potentially transforming telemedicine and diagnostic imaging.
Autonomous Vehicles: Superior object detection in real-time scenes can significantly enhance the safety and reliability of self-driving technologies.
Surveillance: The ability to detect and classify objects in various environmental conditions can bolster security systems and urban monitoring services.
These innovations can unlock new revenue streams by enabling companies to offer new services and improve existing systems through enhanced process efficiencies.
Model Architecture and Training
Hyperparameters and Training Specifications
Hyperparameters are crucial for setting up and training the model. The LGA model employs convolutional layers of variable kernel sizes (such as 3, 5, and 7), which process local patterns at different granularities. The model is equipped with a positional encoding mechanism to retain spatial relationships critical for object detection tasks. Training is typically conducted using standard optimizers like SGD and Adam, which are well-suited for handling complex and high-dimensional data.
Hardware Requirements
The Local-Global Attention mechanism is designed to be computationally efficient, allowing its integration into existing frameworks like MobileNetV3 and ResNet, both known for their applicability even on lightweight, mobile hardware. This design choice makes it feasible for businesses to implement this technology without extensive hardware upgrades.
Target Tasks and Datasets
This paper evaluates LGA's performance across diverse datasets such as VisDrone2019, VOC2012, COCOminitrain, and more, focusing on object detection and classification tasks. These datasets present a variety of challenges in terms of image complexity and object sizes, showcasing LGA's versatility and robustness.
Comparison with State-of-the-Art (SOTA)
The Local-Global Attention mechanism consistently outperforms other attention models like Squeeze-and-Excitation, CBAM, and ECA in terms of accuracy and adaptability to various datasets. The paper reports improvements in metrics such as mAP@50 and mAP@50-95 across different neural network backbones, indicating LGA's superior integration of local and global features.
Conclusion
The introduction of the Local-Global Attention mechanism marks a significant advancement in the realm of attention models. By uniting the best aspects of local and global feature processing within a single framework, it paves the way for more accurate and efficient machine learning applications. For businesses, this means new pathways to innovate and optimize processes, ultimately enabling a competitive edge in technology-centric domains. Such innovations not only provide practical solutions but also open the doors to unexplored markets and opportunities.
Subscribe to my newsletter
Read articles from Gabi Dobocan directly inside your inbox. Subscribe to the newsletter, and don't miss out.
Written by
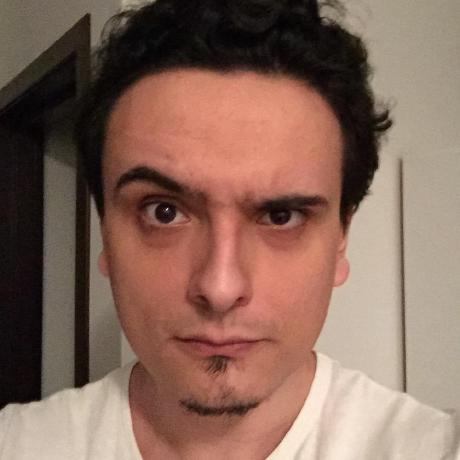
Gabi Dobocan
Gabi Dobocan
Coder, Founder, Builder. Angelpad & Techstars Alumnus. Forbes 30 Under 30.