Exploring the Potential of Dynamic Granularity Timeline Summarization (DTELS) for Business Optimization
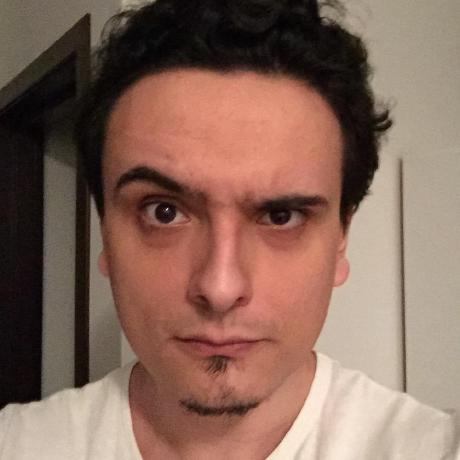

- Arxiv: https://arxiv.org/abs/2411.09297v1
- PDF: https://arxiv.org/pdf/2411.09297v1.pdf
- Authors: Jun Zhao, Kang Liu, Yubo Chen, Zhuoran Jin, Pengfei Cao, Tong Zhou, Chenlong Zhang
- Published: 2024-11-14
The field of natural language processing is rapidly evolving, and one of the exciting new developments is the introduction of Dynamic Granularity Timeline Summarization (DTELS). This technique is designed to address the limitations of traditional timeline summarization methods by offering flexible granularity tailored to user needs and the dynamic nature of news topics. This blog explores the DTELS approach, its applications, training specifics, and how it compares to state-of-the-art alternatives.
Main Claims and Contributions
The DTELS framework addresses a pressing issue in the timeline summarization domain—handling diverse granularity needs. Unlike static timeline approaches, DTELS allows for the creation of timelines that can adjust their granularity based on real-time user requirements or the particular nature of news events. The main contributions of DTELS include:
- Task Definition: DTELS introduces the concept of dynamic granularity in timeline summarization.
- Evaluation Framework: Provides metrics such as informativeness, granular consistency, factuality, and coherence.
- Large-scale Dataset: Compiles over 55,000 articles into a dataset supporting multi-granularity analysis.
- Advanced Models: Evaluates both large language models (LLMs) and existing timeline summarization techniques.
New Proposals and Enhancements
The core enhancement proposed by DTELS is its ability to generate dynamically structured timelines, which are tailored based on the granularity needs specified by different users or for different topics. This flexibility enables more personalized and contextually relevant summaries than traditionally possible with static systems. Additionally, the paper explores robust evaluation frameworks and datasets to enhance timeline generation fidelity.
How Companies Can Leverage DTELS
For businesses, the implications of DTELS are diverse and impactful:
- Personalized Content Delivery: Companies can use DTELS to provide customers with timelines that match their specific interests or information needs, thus improving user engagement.
- Efficient Reporting: Organizations involved in journalism or content aggregation can leverage DTELS for faster, more relevant news synthesis, tailored to specific audience segments.
- Crisis Management: DTELS allows for real-time summary adjustments during crisis management, providing stakeholders with the most pertinent information as events unfold.
- Data Breach Analysis: Businesses can use fine-grained timelines to understand the sequence and impact of data breaches, aiding better cybersecurity responses.
Hyperparameters and Model Training
In terms of model training, the DTELS framework employs large language models that need to handle varying contexts based on granularity requirements. The model performance is heavily influenced by factors such as context window size, with larger windows allowing for broader event coverage. The models are evaluated for their ability to create coherent, informative, and factually accurate summaries under different granularity settings.
Hardware Requirements
Running and training DTELS models, especially large language models, requires significant computational resources. The available models are evaluated using APIs, which, while effective, can be cost-prohibitive. Deploying these models in production scenarios will necessitate robust hardware capable of handling intensive computational loads.
Target Tasks and Datasets
DTELS is targeted at tasks that require nuanced understanding of temporal sequences, such as news reporting, historical analysis, and trend monitoring in social media. The accompanying dataset, DTELS-Bench, supports multi-source input and is particularly refined for use in Chinese language tasks.
Comparison with Other SOTA Alternatives
DTELS outperforms many current state-of-the-art (SOTA) methods by offering more flexibility in timeline construction. It provides superior performance in informativeness and coherence but still faces challenges in fully aligning its results with the desired granularity levels. The comparative analysis also highlights the potential for further improvements in model precision and dataset diversity.
In summary, DTELS represents a significant step forward in temporal text summarization, offering businesses a versatile tool for dynamic content processing. By tailoring summaries dynamically, companies can enhance their data analysis capabilities, improve customer engagement through personalized content, and optimize decision-making processes across various strategic domains.
Subscribe to my newsletter
Read articles from Gabi Dobocan directly inside your inbox. Subscribe to the newsletter, and don't miss out.
Written by
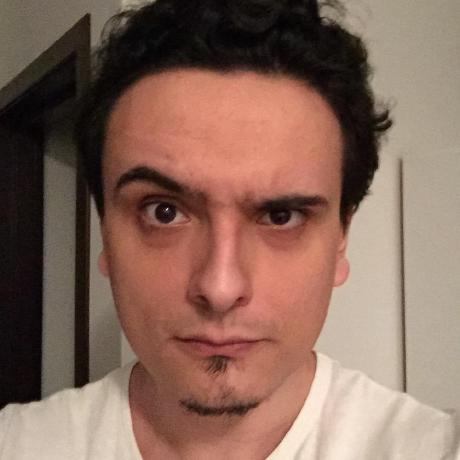
Gabi Dobocan
Gabi Dobocan
Coder, Founder, Builder. Angelpad & Techstars Alumnus. Forbes 30 Under 30.