Understanding Multi-Perspective Stance Detection: A Key to Responsible AI
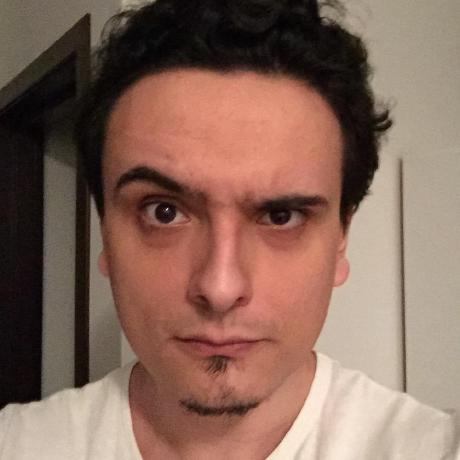

- Arxiv: https://arxiv.org/abs/2411.08752v1
- PDF: https://arxiv.org/pdf/2411.08752v1.pdf
- Authors: Fosca Giannotti, Lucia Passaro, Gizem Gezici, Praveen Bushipaka, Benedetta Muscato
- Published: 2024-11-13
The world of Natural Language Processing (NLP) constantly seeks methods to improve accuracy and applicability. One exciting proposal is "Multi-Perspective Stance Detection," a fresh approach altering how models process subjective tasks like stance detection. This blog unpacks a recent scientific paper exploring this method, delving into its implications, applications, and the tech details that can harness new business opportunities.
Main Claims of the Paper
The paper asserts that traditional NLP methods, which often condense multiple annotations into a single 'truth,' can be limiting. They propose a multi-perspective approach, allowing models to access varied annotations from human annotators, acknowledging the subjectivity and richness of diverse perspectives.
This methodology not only enhances ethical and responsible AI development by respecting differing viewpoints, but it also boosts model performance. By leveraging the variability in human annotations, the new models improve classification accuracy over standard approaches that use aggregated data.
New Proposals and Enhancements
The core enhancement in this paper is adopting a method that respects and uses the diversity inherent in subjective NLP tasks like stance detection. Instead of forcing a consensus (or majority rule), the new technique retains multiple annotations. This richer dataset provides models with a broader context, aiding in constructing more nuanced outputs. This approach is dubbed the 'perspectivist approach'.
Another critical feature includes 'chunking', where document inputs exceeding word limits of models like BERT and RoBERTa are broken into smaller segments to maintain context. By doing so, the models can process long documents more effectively without losing integral information.
Leveraging the Paper for Business
Businesses, especially those in sectors like content moderation, sentiment analysis, and market research, can significantly benefit from this approach. By integrating multi-perspective models:
Enhanced Content Moderation and Sentiment Analysis: Companies can achieve greater accuracy in determining sentiment or stance in content. This is crucial for social media platforms and companies monitoring brand reputation.
Product Development: Businesses can develop new tools that take subjective data into account. For instance, personalized customer service solutions that interpret different customer tones and sentiments more accurately.
Diversity and Inclusion Tools: Develop applications that ensure diverse perspectives are considered in training AI, promoting fairness in AI decisions across different demographic categories.
With these new applications, companies can optimize their processes and even unlock new revenue streams by offering differentiated AI products to the market.
Hyperparameters and Model Training
The models use base configurations of BERT and RoBERTa with chunking. A batch size of 32 and a four-epoch training cycle are typical, using 32GB Tesla V100 GPUs for their processing. By using default hyperparameters, including learning rates and dropout ratios, the researchers found that the multi-perspective models with chunking yielded the best results.
Hardware Requirements
To use these models, especially during the training phase, companies need access to high-performance computing resources. Specifically, the experiments utilized Tesla V100 GPUs with 32GB memory. This specification ensures that the computations required for processing large datasets and handling multiple perspectives can be efficiently managed.
Target Tasks and Datasets
The target task for this approach is stance classification, particularly using datasets that contain subjective interpretations. The dataset in this study consists of news documents annotated for stance (pro, neutral, against) using crowd-sourcing via the MTurk platform. Such datasets are rich in variability, perfectly suited to test the multi-perspective model approach.
Comparison with State-of-the-Art Alternatives
In comparison to standard models using single-label aggregation, the multi-perspective approach significantly increases the accuracy and robustness of stance detection tasks. The paper highlights that multi-perspective models outperform baseline models like BERT and RoBERTa configured traditionally, showing superior results and gaining confidence even with smaller, diverse datasets.
To conclude, the approach of incorporating diverse human perspectives into NLP models is a breakthrough that can revolutionize subjective learning tasks in AI. By respecting annotation diversity, companies can develop more inclusive and accurate AI solutions, paving the way for advancements in ethical and responsible AI.
Subscribe to my newsletter
Read articles from Gabi Dobocan directly inside your inbox. Subscribe to the newsletter, and don't miss out.
Written by
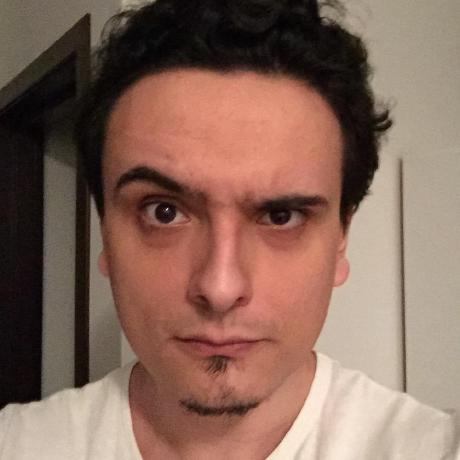
Gabi Dobocan
Gabi Dobocan
Coder, Founder, Builder. Angelpad & Techstars Alumnus. Forbes 30 Under 30.