United Domain Cognition Network (UDCNet) for Salient Object Detection
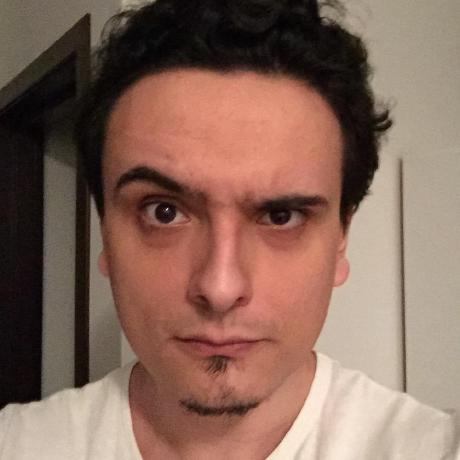
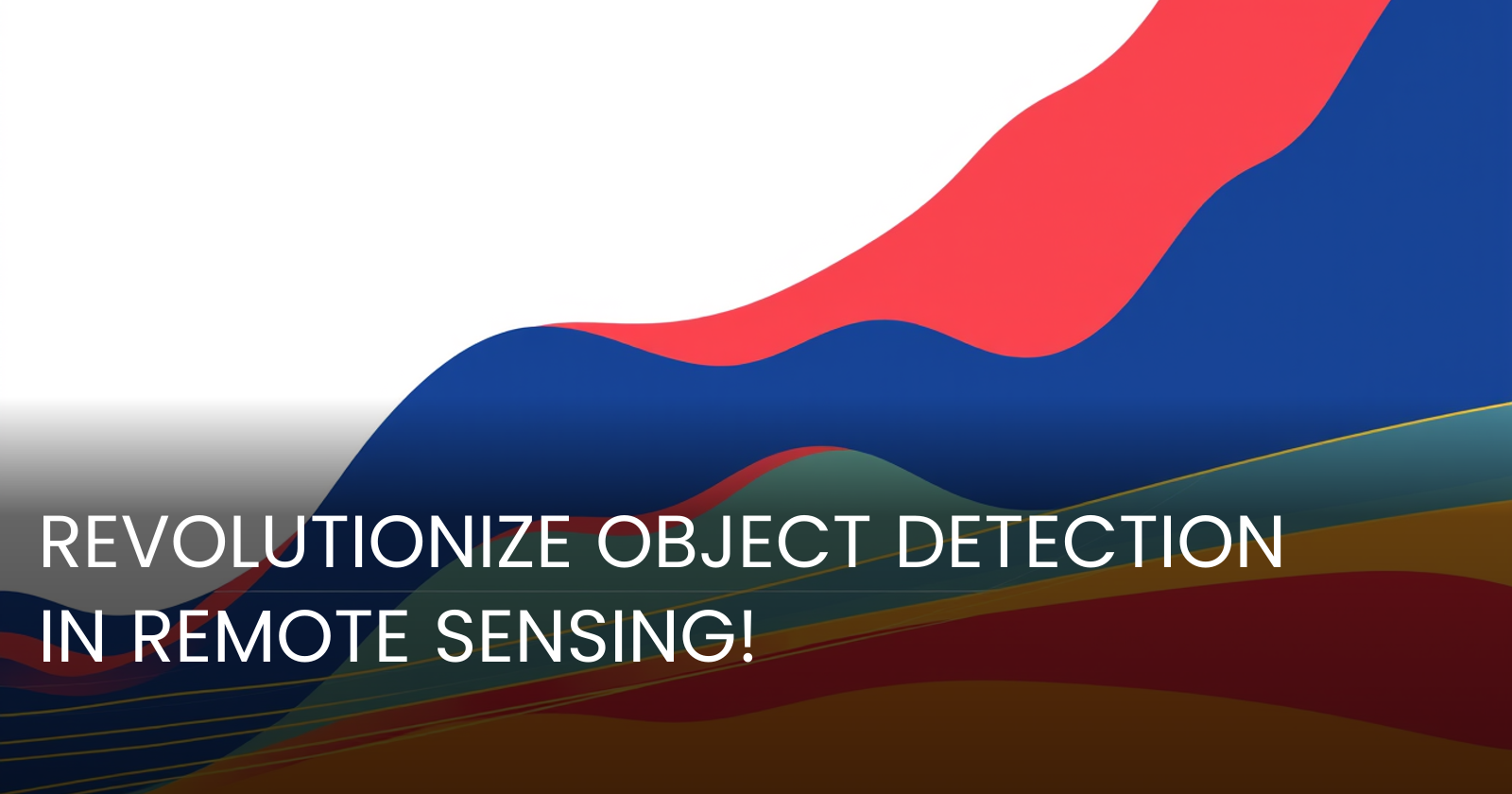
- Arxiv: https://arxiv.org/abs/2411.06703v1
- PDF: https://arxiv.org/pdf/2411.06703v1.pdf
- Authors: Lei Luo, Jian Yang, Yanguang Sun
- Published: 2024-11-11
Introduction
The world of remote sensing is vast and complex, filled with images that hold immense value for various applications like environmental monitoring, urban planning, and disaster management. A recent scientific paper introduces a groundbreaking approach called the United Domain Cognition Network (UDCNet), which aims to improve salient object detection in optical remote sensing images (ORSIs). Let's dive into the essence of this innovation, exploring its components, benefits, and potential applications for businesses in a way that's easy to understand for tech enthusiasts and business leaders alike.
Core Claims of the Paper
UDCNet proposes a novel method for salient object detection, which is crucial for distinguishing significant objects in a scene. The core claim of the paper is that by integrating both global frequency and local spatial information, UDCNet achieves superior detection accuracy over existing methods. Conventional salient object detection methods in remote sensing typically focus on local spatial features, which can limit their effectiveness. UDCNet leverages the Fourier transform to overcome this by incorporating global information, which enhances the detection capabilities.
New Proposals and Enhancements
Components of UDCNet
Frequency-Spatial Domain Transformer (FSDT) Block: This novel block combines local spatial and global frequency features, optimizing them through spatial perception self-attention (SPSA) and frequency perception self-attention (FPSA), along with an adaptive fusion strategy (AFS).
Dense Semantic Excavation (DSE) Module: This module is designed to capture higher-level semantics using advanced atrous convolutions and dense connections, which guide the precise localization of salient objects.
Dual-Branch Joint Optimization (DJO) Decoder: This decoder features two branches for optimizing both the structure and boundary information of detected objects, by using edge and saliency branches.
How Companies Can Capitalize on This Innovation
Unlocking Revenue and Optimizing Processes
Remote Sensing Services: Companies specializing in satellite imaging can use UDCNet for improved image analysis, facilitating more accurate land use mapping, deforestation monitoring, and urban development planning.
Security and Surveillance: Enhanced detection capabilities can be applied in surveillance systems, offering more precise monitoring and threat detection in both urban and rural settings.
Agricultural Monitoring: Farmers and agribusinesses could leverage the detailed object detection to monitor crop health, forecast yields, and optimize resource allocation, thereby increasing productivity and reducing costs.
New Product and Business Opportunities
AI-enhanced Imaging Platforms: Development of software platforms that use UDCNet for advanced imaging capabilities across various sectors such as environmental science, military applications, and urban development.
Customizable Analysis Solutions: Offering tailor-made solutions for industries that require specific detection capabilities, from tracking wildlife movements to identifying changes in coastal regions.
Understanding Hyperparameters and Model Training
The model's hyperparameters are fine-tuned to balance performance and resource utilization. For UDCNet, the channel hyper-parameter is set to 128, which strikes an ideal balance between model accuracy and computational complexity. The training process involves extensive use of self-attention mechanisms and convolution techniques optimized for both speed and accuracy.
Hardware Requirements
Training and running UDCNet demands significant computational power. The model's complexity, characterized by its dense semantic features and dual-branch optimization, typically requires powerful GPU systems to perform efficiently without bottlenecks. This setup is crucial to handle the billions of operations per second required for real-time processing.
Target Tasks and Datasets
UDCNet is particularly designed for tasks such as salient object detection in complex environments primarily consisting of optical remote sensing images. The model was rigorously tested on datasets like ORSSD, EORSSD, and ORSI-4199, which are benchmarks in the field, providing comprehensive scenarios for validation.
Comparison with State-of-the-Art Alternatives
When compared with current state-of-the-art methods, UDCNet demonstrates significantly improved accuracy and generalization capabilities. The model outperforms 24 existing salient object detection methods in both natural and remote sensing images. This is attributed to its ability to integrate global frequency information with local spatial features, something few other models achieve as effectively.
Conclusion
UDCNet represents a pivotal advancement in the realm of remote sensing, offering a sophisticated method for improving object detection accuracy in optical images. By bridging the gap between local spatial features and global frequency information, it sets a new benchmark for the industry. Companies across various sectors can harness this technology to enhance their imaging capabilities, opening doors to innovative applications and business models. Whether it's in enhancing security, optimizing agricultural processes, or advancing digital surveillance, the potential applications of UDCNet are vast and varied.
Subscribe to my newsletter
Read articles from Gabi Dobocan directly inside your inbox. Subscribe to the newsletter, and don't miss out.
Written by
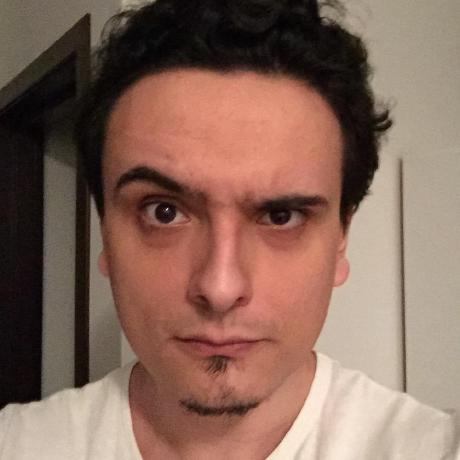
Gabi Dobocan
Gabi Dobocan
Coder, Founder, Builder. Angelpad & Techstars Alumnus. Forbes 30 Under 30.