StoryTeller: Revolutionizing Long Video Descriptions
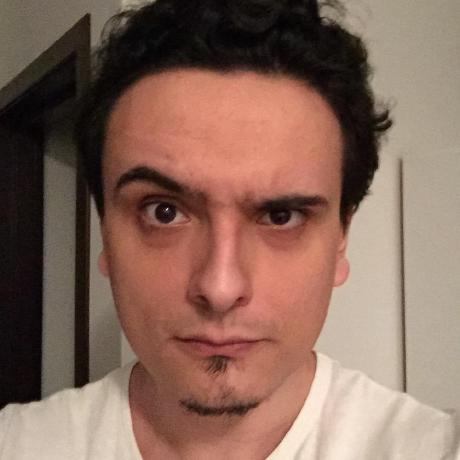

- Arxiv: https://arxiv.org/abs/2411.07076v1
- PDF: https://arxiv.org/pdf/2411.07076v1.pdf
- Authors: Ruicheng Le, Yuchen Zhang, Hanchong Zhang, Jianchao Wu, Yuan Lin, Yichen He
- Published: 2024-11-11
Understanding videos, especially long-form content, is a significant leap in the domain of artificial intelligence. The paper on "StoryTeller" brings forth innovative methods for generating detailed and coherent video descriptions over extended footage, such as movies, which are often rich in plot and character development. Let's dive into the key aspects of this research and explore how enterprises might harness this technology.
Main Claims of the Paper
The paper introduces StoryTeller, a groundbreaking automated system designed to produce dense descriptions for long videos by improving the character identification process over previous technologies. The authors claim that existing vision-language models (LVLMs) struggle with maintaining consistency and coherence in the storyline, especially for videos longer than a few seconds.
StoryTeller significantly surpasses existing models, like Gemini-1.5-pro, in delivering coherent video descriptors by an accuracy margin of 9.5% in their evaluations. This system integrates a novel audio-visual character identification process that enriches video descriptions across multiple large vision-language models (LVLMs).
New Proposals and Enhancements
StoryTeller stands out by incorporating an audio-visual character identification module along with a video segmentation component and a description generation module. The novel integration of these elements ensures that plot-level detail and consistency are maintained throughout the video analysis.
A new approach to processing dialogues and assigning IDs to characters helps keep track of each character’s role in the narrative across multiple segments of a video. The system ensures that characters are correctly identified regardless of scene breaks, which was a challenging aspect for previous models.
Applications and Business Opportunities
For businesses, the implications of such an AI tool are vast. Companies involved in media, entertainment, and video content platforms can leverage StoryTeller to enhance user engagement. For instance, video streaming services can use detailed descriptions generated by StoryTeller to improve content recommendation systems, making them more relatable by analyzing complex plot structures and character developments.
Moreover, advertising agencies could utilize this technology to automate the indexing and retrieval of video segments containing specific content features for targeted campaigns. It can also aid in generating trailers or summaries automatically, allowing content creators to focus on creative processes rather than the logistics of content promotion.
Training Aspects and Hyperparameters
Training the system involves a three-phase regime emphasizing pre-training their audio components, followed by fine-tuning on individual and combined audio-visual tasks like character tracking and identification. A large dataset, MovieStory101, specifically constructed for such tasks, facilitates the learning process.
Hyperparameters used include elements like the window-level configurations for processing audio, where a 30-second segment can be distilled into manageable text tokens. Training was carried out on highly resource-intense hardware setups using multiple GPUs to maximize the learning efficiency.
Hardware Requirements
Given the sophistication of the StoryTeller model, substantial computational resources are required. The model training took place across 16 H800 GPUs, indicative of the significant power dedicated to handling large-scale video understanding tasks.
Target Tasks and Datasets
The primary application target for StoryTeller is the movie and entertainment industry, requiring detailed descriptions of dynamically changing plots and complex character interactions. The authors have created a specialized dataset, MovieStory101, containing clipped narratives from over a hundred movies, while MovieQA provides a complementary evaluation framework through answer-question pairs.
Comparisons with State-of-the-Art (SOTA)
Compared to contemporary models like Gemini-1.5-pro and open-source models such as VILA1.5-8B, StoryTeller demonstrates a distinct advantage in enriching the descriptive capabilities of long films. It leverages a more comprehensive modality blend, extending beyond visuals alone by bringing audio and textual inputs into a unified processing model.
In summary, StoryTeller represents a significant advancement in the AI-driven analysis of long videos. Its effective multimodal approach not only automates but vastly improves the descriptive process of video content, opening new avenues for enterprises to utilize advanced technologies in enriching user experiences and optimizing digital content processing cycles. As this technology matures, we can anticipate even broader applications beyond entertainment, potentially transforming educational tools, video archiving systems, and more.
Subscribe to my newsletter
Read articles from Gabi Dobocan directly inside your inbox. Subscribe to the newsletter, and don't miss out.
Written by
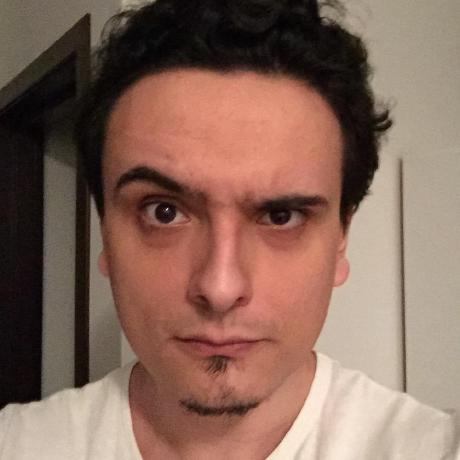
Gabi Dobocan
Gabi Dobocan
Coder, Founder, Builder. Angelpad & Techstars Alumnus. Forbes 30 Under 30.