Transforming Music Discovery with AI: A Dive into Conversational Music Retrieval
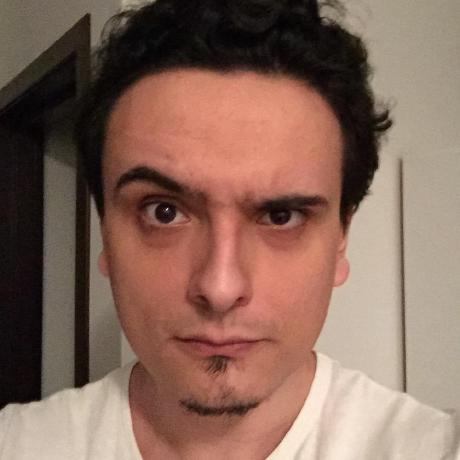

- Arxiv: https://arxiv.org/abs/2411.07439v1
- PDF: https://arxiv.org/pdf/2411.07439v1.pdf
- Authors: Juhan Nam, Taesu Kim, Daeyong Kwon, Keunwoo Choi, Seungheon Doh
- Published: 2024-11-11
Imagine being able to chat with a system that truly understands your musical taste, helping you discover new tunes that perfectly match your mood or activity. This level of interaction is becoming possible thanks to advancements in AI dialogue systems, particularly in the realm of music discovery. Let's explore a fascinating study that outlines a new framework designed to generate engaging, human-like music dialogues. This research doesn’t just add value in terms of technological innovation; it potentially reshapes how businesses can leverage AI to enhance user experiences and optimize media recommendation processes.
What Are the Main Claims?
The scientific paper introduces a sophisticated framework for generating human-like music discovery dialogues. The approach revolves around utilizing large language models (LLMs) enhanced with human intent analysis and cascading music database filtering. Essentially, the system is designed to understand what users are looking for and generate meaningful, fluid conversations that guide them to their ideal music choices.
New Proposals and Enhancements
The key innovations in this paper include the development of a taxonomy for music dialogue that incorporates user intents, system actions, and musical attributes. Additionally, the authors introduce LP-MusicDialog, a large-scale synthetic dialogue dataset creating pseudo music dialogues by filtering through a rich database of music attributes. By applying cascading filters and LLM-driven intent analysis, the framework moves away from the traditional static template responses, thus offering more dynamic and contextually relevant dialogue interactions.
How Can Companies Leverage This Technology?
The potential for expanding business models with this technology is truly exciting. Companies in the music streaming industry can use this to enhance their recommender systems, transforming passive music suggestions into active conversational experiences. Imagine integrated customer support or personalized DJ services that can offer real-time recommendations as users express their preferences.
Startups could explore building standalone applications focusing on niche recommendations, like workout music or study pods, that operate entirely through conversational interfaces. Retailers looking to incorporate music into their stores or brands can use this AI system to craft playlists that reflect a customer’s in-store activity or purchasing behavior.
Hyperparameters and Model Training Overview
While the paper does not exhaustively detail the hyperparameters in use, it emphasizes using large language models that are tailored with user intents and musical attributes. This involves processes like top-k sampling to ensure a diverse selection of musical attributes which support nuanced, human-like dialogue generations.
Hardware Requirements
Details on the hardware specifics aren't thoroughly laid out, but given the use of LLMs and complex filtering algorithms, we can infer a need for robust computing power. Companies looking to implement such a system would likely require machines with strong GPU capabilities to handle model training, coupled with extensive storage to maintain and access the large datasets involved.
Target Tasks and Datasets
The LP-MusicDialog dataset introduces a rich tapestry of synthetic dialogues. It leverages the Million Song Dataset as one of its foundational elements, allowing the generated dialogues to be open to deep customization based on user intents and music metadata. This dataset is designed to refine conversational music retrieval tasks, simulating real-world user interactions with a music discovery system.
Comparisons to State-of-the-Art Alternatives
Compared to other conversational music systems that rely heavily on static template responses and limited interaction depth, this new framework significantly enhances the dialogue naturalness and item relevance. The paper's approach surpasses existing methods by enriching conversations with dynamically generated music attributes, resulting in a higher quality and more satisfying user experience.
In wrapping up, this paper presents a transformative step towards making music discovery more engaging and personal. By incorporating user intent and applying advanced language models, businesses can elevate their service offerings and harness AI-driven insights to optimize user engagement and satisfaction. The future of dialogue-based music recommendation looks incredibly promising, inviting a host of new entrepreneurial ventures and technological advancements.
Subscribe to my newsletter
Read articles from Gabi Dobocan directly inside your inbox. Subscribe to the newsletter, and don't miss out.
Written by
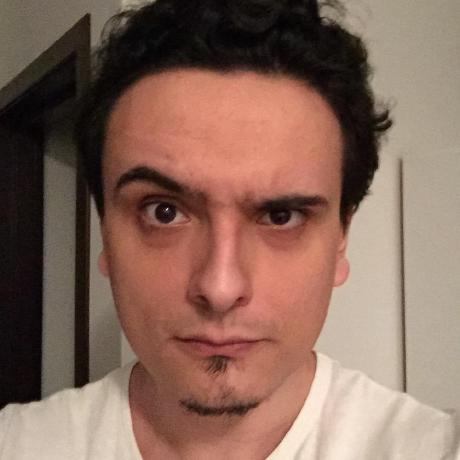
Gabi Dobocan
Gabi Dobocan
Coder, Founder, Builder. Angelpad & Techstars Alumnus. Forbes 30 Under 30.