Unlocking the Future of Conversational Music Retrieval: Insights from Recent Advances
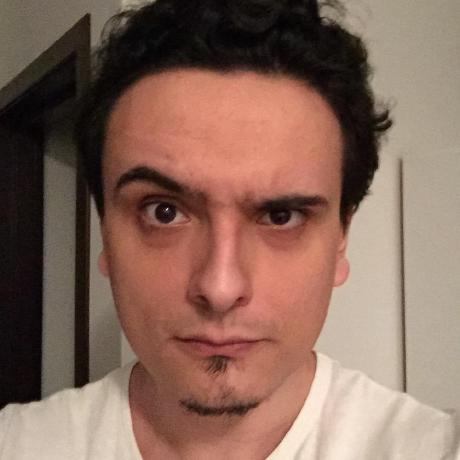

- Arxiv: https://arxiv.org/abs/2411.07439v1
- PDF: https://arxiv.org/pdf/2411.07439v1.pdf
- Authors: Juhan Nam, Taesu Kim, Daeyong Kwon, Keunwoo Choi, Seungheon Doh
- Published: 2024-11-11
In this article, we dive into an exciting, boundary-pushing paper that presents a novel framework for enhancing music discovery through conversational dialogue. This groundbreaking approach leverages human intent analysis and large language models (LLMs) to revolutionize how users interact with music recommendation systems. Let's explore the key innovations of this study and examine how they can be applied to optimize processes and generate new value in various industry sectors.
Main Claims of the Paper
The main claim of this research is the introduction of a sophisticated framework designed to generate human-like music discovery dialogues by utilizing user intents and large language models (LLMs). This framework addresses previous limitations found in music retrieval systems, particularly those dependent on single-turn interactions that don't fully capture user preferences over multiple dialogue turns. The research highlights the effectiveness of this approach by developing a taxonomy for user intents, music attributes, and system actions, which help create more natural and meaningful conversations.
New Proposals and Enhancements
One of the key enhancements proposed by this study is the method of cascading music database filtering to generate attribute sequences. This method replaces the traditional joint embedding techniques with a more flexible, data-driven approach that applies filtering to a multi-label music annotation database . Furthermore, the research introduces LP-MusicDialog, a large synthetic dataset of music dialogues created by combining human intent analysis with LLM-based dialogue generation.
Leveraging the Technology: Business Applications
Enhancing Music Recommendation Systems
Businesses in the streaming music industry, such as Spotify and Apple Music, can utilize this framework to offer enriched, multi-turn conversational experiences that cater to user preferences with greater precision. By adopting this framework, companies can improve customer engagement and retention by providing more personalized interactions than current static or single-turn solutions.
Potential for New Business Models
Beyond music, this technology can be adapted for any domain relying on data-driven recommendations. Fashion, film, and even food delivery services could harness the personalization capabilities offered by conversational systems that understand nuanced customer preferences over time.
Advancements in Customer Support
Businesses that manage high volumes of customer interaction might also deploy this technology within their support systems. By utilizing the dialogue handling capabilities described in this paper, companies can build systems that more effectively resolve customer issues by understanding their intent through multi-turn dialogues.
Hyperparameters and Model Training
The paper does not provide exhaustive details on specific hyperparameters but outlines the use of a pre-trained audio-text joint embedding model, TTMR++. For training, a chat embedding technique maximizes the similarity between chat history and target music, optimizing recommendation accuracy.
Hardware Requirements
While specifics on hardware requirements are not detailed in the text, implementing this framework likely requires considerable computational resources, particularly for training and fine-tuning large-scale language models like GPT-3. Typically, this implies access to GPUs or TPUs to efficiently manage the computational load.
Target Tasks and Datasets
This research focuses on conversational music retrieval as its primary target task . It contrasts the newly proposed LP-MusicDialog dataset against existing datasets like the Conversational Playlist Curation Dataset (CPCD), highlighting that LP-MusicDialog offers a broader vocabulary and more diverse dialogues .
Comparing to State-of-the-Art (SOTA) Alternatives
Comparisons are made between their proposed methods and other models, such as BM25 and Contriever. The results show that the newly proposed joint audio-text embedding model, TTMR++, outperformed other methods, demonstrating the importance of incorporating audio data into retrieval tasks. This highlights the value of more comprehensive, multimodal approaches in music recommendation systems compared to prior solutions that primarily focused on text-based metadata.
In summary, this paper offers significant advancements in the field of conversational recommendation systems, providing a framework that is not only applicable within the music industry but adaptable across any sector that benefits from advanced, user-centric interaction models. The potential applications of this research are vast, promising enhancements in user experience and unlocking new revenue possibilities for innovative businesses.
Subscribe to my newsletter
Read articles from Gabi Dobocan directly inside your inbox. Subscribe to the newsletter, and don't miss out.
Written by
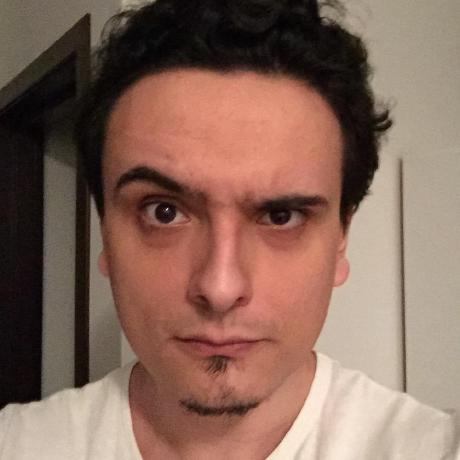
Gabi Dobocan
Gabi Dobocan
Coder, Founder, Builder. Angelpad & Techstars Alumnus. Forbes 30 Under 30.