Understanding LRSAA: A Breakthrough in Remote Sensing Image Processing
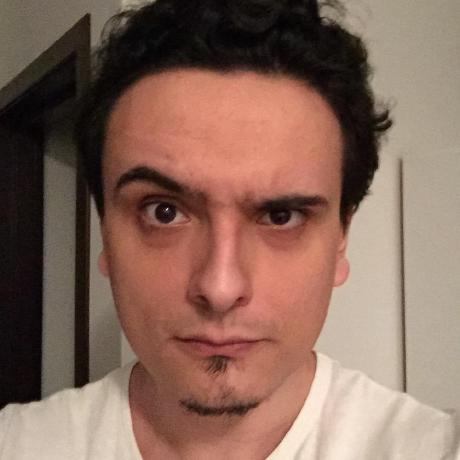
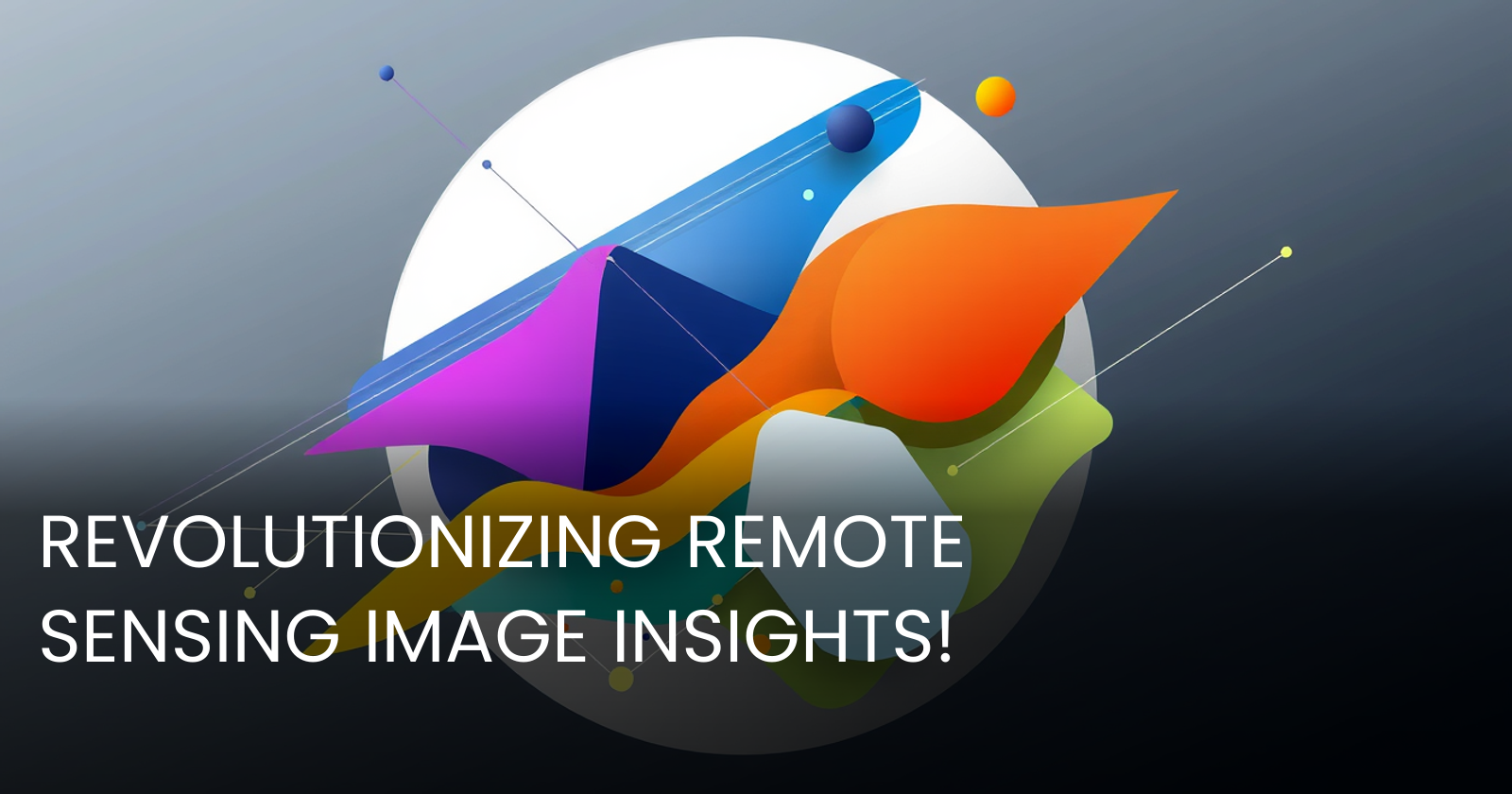
- Arxiv: https://arxiv.org/abs/2411.07802v1
- PDF: https://arxiv.org/pdf/2411.07802v1.pdf
- Authors: Wuzheng Dong
- Published: 2024-11-12
Let's dive deeply into the intriguing paper "Large-scale Remote Sensing Image Target Recognition and Automatic Annotation" (LRSAA). This research presents a transformative approach to recognizing and annotating targets in expansive remote sensing images. If you're not steeped in the jargon of machine learning or satellite imagery, don’t worry. This article will break down the technology, explore its potential applications in business, and compare it to current state-of-the-art approaches in the field.
Main Claims of the Paper
The LRSAA framework proposes a novel system that integrates advanced object detection models—specifically YOLOv11 and MobileNetV3-SSD—through ensemble learning. This integration achieves superior performance by capitalizing on the strengths of each model while minimizing computational resources. The paper highlights that this approach not only strikes a balance between accuracy and speed but also excels in resource-constrained conditions.
New Proposals and Enhancements
The LRSAA framework is notable for several innovative features:
- Ensemble Learning: Combines YOLOv11 and MobileNetV3-SSD models to leverage their combined capabilities.
- EIOU Metric for NMS: Uses the Enhanced Intersection Over Union (EIOU) metric against the conventional IoU, allowing for more nuanced object detection with geometric considerations.
- Poisson Disk Sampling: Implements this technique for image segmentation, ensuring balanced dataset distribution and improved data representativeness.
Business Applicability: Revenue and Optimizations
For businesses involved in geographical data analysis, urban planning, disaster management, or environmental monitoring, the LRSAA framework offers a powerful tool for enhancing data processing capabilities. Companies can leverage this technology in multiple ways:
- Real-time Monitoring and Disaster Response: Optimized for time-sensitive applications, such as tracking environmental phenomena or responding to natural disasters.
- Resource Management: Improves the efficiency of resource allocation through precise and rapid assessments of large geographic areas.
- Data-Driven Decision Making: An enhanced ability to process vast amounts of image data can lead to better strategic decisions in sectors like agriculture, forestry, and urban planning.
Training Insights and Hyperparameters
LRSAA's training regimen utilizes the XView dataset, a comprehensive collection of satellite images. Initial training employs these datasets at smaller scales (e.g., 640×640 pixels) to enhance processing speed and accuracy. The framework employs synthetic data generation to boost model performance by creating a richer training dataset, enhancing model robustness and adaptability.
Hyperparameters and Training Techniques
Hyperparameter tuning and the effective use of ensemble learning with Poisson disk sampling form the core of LRSAA’s training methodology. While specific hyperparameter values aren't detailed, the integration of diverse techniques indicates a robust framework primed for optimization across various conditions.
Hardware Requirements
The paper emphasizes optimizing computational efficiency, yet specific hardware requirements aren't detailed directly. However, given the use of MobileNetV3 (known for its efficiency on mobile platforms), it's reasonable to infer that LRSAA is well-suited for deployment on devices ranging from edge computing platforms to cloud environments.
Target Tasks and Datasets
The framework is especially designed for large-scale remote sensing applications, exemplified by its demonstration on the XView dataset and subsequent application to satellite images of urban areas like Tianjin.
Comparing LRSAA with Other State-of-the-Art Alternatives
The evaluations indicate that LRSAA outperforms existing models like YOLOv5, R-CNN, and Mask R-CNN across various evaluation metrics on smaller, constraint-rich datasets. This marks a significant advancement in object detection, especially for large-scale images where traditional models struggle with accuracy and computational demand.
Conclusion and Future Perspectives
The LRSAA framework represents a significant leap forward in remote sensing image processing, offering enhanced accuracy and resource efficiency through innovative model integrations and techniques. As companies continue to seek more nuanced and rapid analysis of geographic and environmental data, tools like LRSAA will allow businesses to unlock new levels of insight and operational efficiency.
Future work may see this framework adapted for even broader datasets and conditions, with improvements in real-time processing capabilities and deployments across diverse platforms, from mobile devices to expansive cloud networks.
In summary, LRSAA is not just a step forward technologically; it's a gateway to a suite of new business opportunities and operational efficiencies across a multitude of industries reliant on large-scale image data analysis.
Subscribe to my newsletter
Read articles from Gabi Dobocan directly inside your inbox. Subscribe to the newsletter, and don't miss out.
Written by
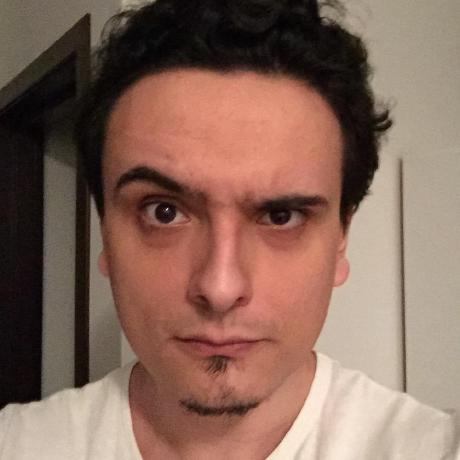
Gabi Dobocan
Gabi Dobocan
Coder, Founder, Builder. Angelpad & Techstars Alumnus. Forbes 30 Under 30.