Understanding PortalGen: A New Approach to Synthesizing Patient Portal Messages
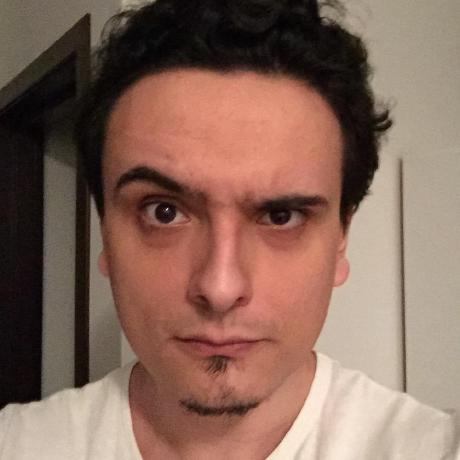

- Arxiv: https://arxiv.org/abs/2411.06549v1
- PDF: https://arxiv.org/pdf/2411.06549v1.pdf
- Authors: Sarah Masud Preum, Timothy E. Burdick, Parker Seegmiller, Joseph Gatto
- Published: 2024-11-10
In this article, we dive deep into a fascinating new paper that explores innovative ways to generate realistic patient portal messages. This breakthrough technology, named PortalGen, provides a HIPAA-friendly framework for producing synthetic patient messages without compromising privacy. Through the lens of this paper, we will discuss the groundbreaking proposals, potential business applications, and implications for healthcare service providers.
Main Claims of the Paper
The central claim of the paper revolves around PortalGen, a two-stage framework that uses large language models (LLMs) like GPT-3.5 to transform healthcare data into realistic patient messages. This framework aims to create a wide array of synthetic patient message corpora, circumventing the need for massive de-identification efforts and making large-scale data release more feasible. The paper argues that PortalGen achieves a delicate balance between generating high-quality synthetic data and maintaining privacy, outperforming existing medical Q&A datasets in both quality and style.
Innovative Proposals and Enhancements
PortalGen introduces a two-stage process for generating patient messages:
Stage 1: Few-Shot Prompting - This stage utilizes LLMs with few-shot learning to convert ICD-9 codes from healthcare databases into patient message prompts. This method offers a diverse array of message scenarios while maintaining healthcare data classification standards.
Stage 2: Grounded Generation - Here, the framework employs grounded text generation techniques. It incorporates a few de-identified patient messages during the prompt stage to ensure that the synthetically generated messages bear close resemblance to actual patient communication styles.
Business Applications and Potential
This framework can revolutionize how companies and healthcare institutions handle data privacy concerns while managing patient interactions. Here are some ways businesses might utilize this technology:
- Data Augmentation for AI Models: Researchers and developers can use this synthetic data to train AI models, improving the accuracy and efficiency of medical AI applications.
- Reducing Clinician Burnout: By automating the generation of patient responses and triaging incoming messages, healthcare providers can streamline workflows and decrease clinician workload.
- Customization of Patient Engagement Tools: Companies can develop tailored communication tools that adhere to privacy laws while ensuring patient messages are authentic and relevant.
Hyperparameters and Training Techniques
The paper doesn't delve deeply into specific hyperparameters used, but it outlines the training process within the constraints of HIPAA compliance. The grounded generation operates with a minimal set of examples (just 10 de-identified messages) to carefully balance privacy with realism. This setup minimizes the necessity for extensive data filtering or tweaking.
Hardware Requirements for Training and Running
The paper notes that PortalGen was evaluated using models run on limited GPU resources without internet access, capping at models smaller than 50 billion parameters like Mixtral 8x7b. This indicates a moderate level of computing power is sufficient, avoiding the need for large-scale hardware investments.
Target Tasks and Datasets
The target application domain in the paper is patient portal messages, using a dataset sourced from a healthcare system in the United States consisting of 610k patient messages.
Comparisons with State-of-the-Art Alternatives
PortalGen is contrasted against several baseline models, including GPT-2 fine-tuned with real patient messages and differential privacy settings. The results indicate that while GPT-2 models trained on real data offer the best absolute performance, PortalGen achieves superior balance in quality metrics such as perplexity and semantic similarity compared to privacy-preserving methods.
Conclusions and Areas for Improvement
PortalGen is a promising step forward for synthetic data generation, especially in sensitive domains like healthcare. However, the study is limited by its focus on a single healthcare dataset and constrained by the computational capabilities regarding model size. Future research could explore the expansion of the scope to multiple datasets and the integration of larger model capacities.
Through pioneering frameworks like PortalGen, healthcare technology continues to advance in ways that significantly ease clinician workloads without compromising on patient privacy, opening new avenues for AI integration in healthcare.
Subscribe to my newsletter
Read articles from Gabi Dobocan directly inside your inbox. Subscribe to the newsletter, and don't miss out.
Written by
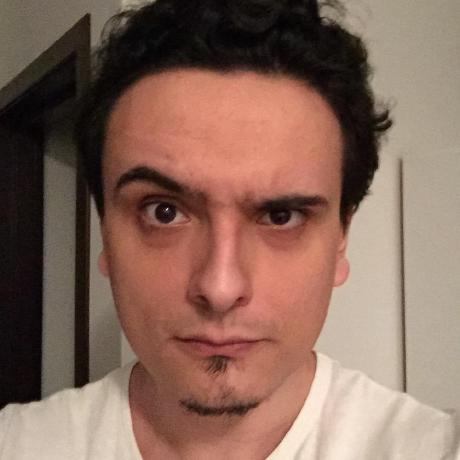
Gabi Dobocan
Gabi Dobocan
Coder, Founder, Builder. Angelpad & Techstars Alumnus. Forbes 30 Under 30.