Top 8 Modern GPUs for Machine Learning

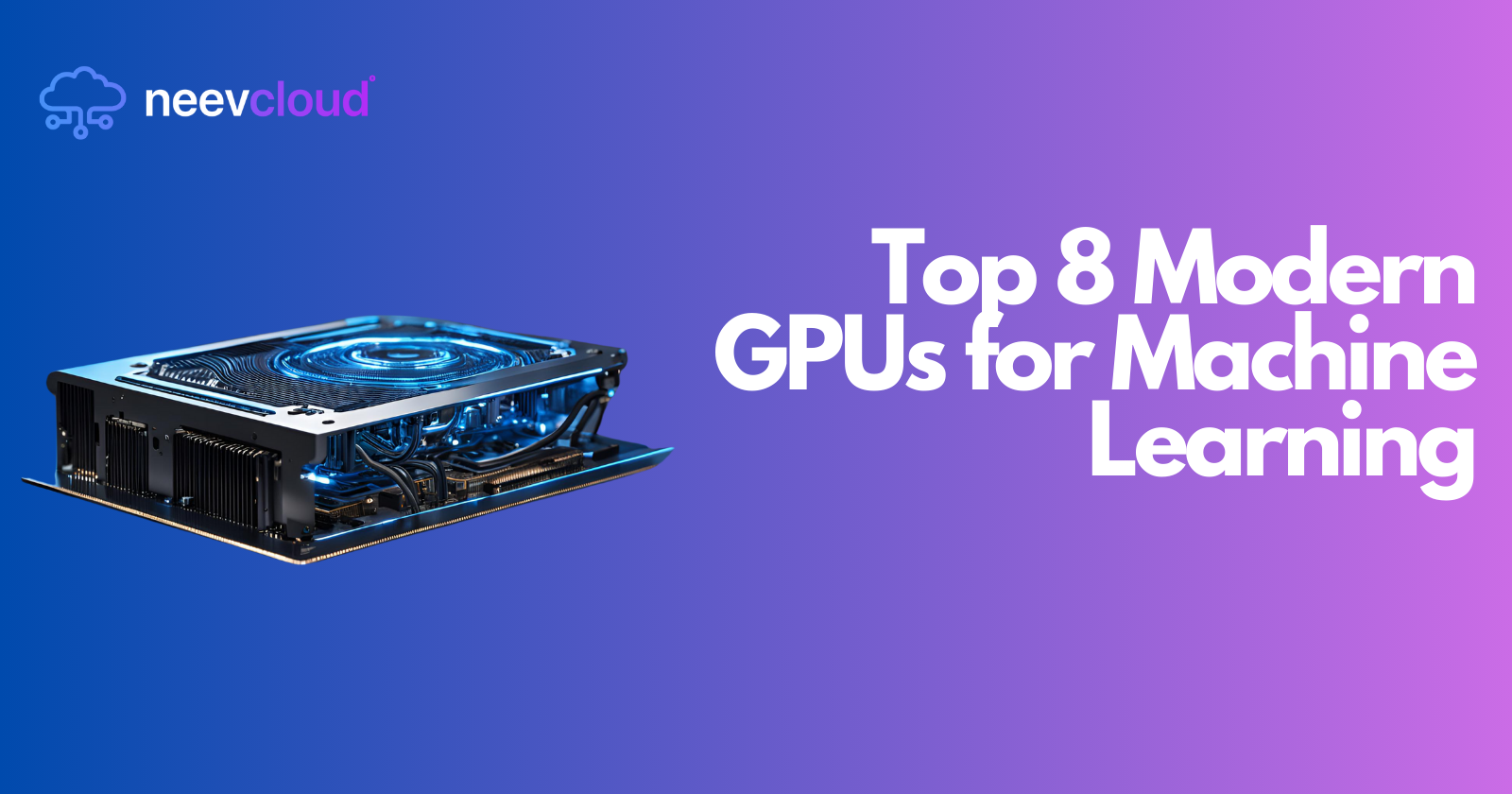
In the rapidly evolving field of machine learning, the choice of hardware can significantly impact the performance and efficiency of AI models. As developers increasingly turn to cloud-based solutions, understanding the capabilities of modern GPUs is essential. This blog post explores the top 8 modern GPUs that are making waves in machine learning, particularly in the context of cloud GPU services, and how they integrate into AI cloud infrastructures.
1. NVIDIA H100 Tensor Core GPU
The NVIDIA H100 is at the forefront of AI and machine learning workloads. With its 80 GB HBM3 memory and unprecedented performance, it is designed for both training and inference tasks. The H100 offers:
Performance: Up to 300 teraflops of AI performance.
Use Cases: Ideal for large-scale model training and high-performance computing (HPC).
Cloud Availability: Widely available across major cloud platforms like AWS, Google Cloud, and Azure.
The H100's architecture supports multi-instance GPU (MIG) technology, allowing multiple networks to run simultaneously on a single GPU, optimizing resource utilization in AI datacenters.
2. NVIDIA A100 Tensor Core GPU
Another powerhouse from NVIDIA, the A100 GPU is designed for versatility across various workloads. Key features include:
Memory: Available in configurations with up to 80 GB of memory.
Performance: Capable of delivering up to 20x higher performance than previous generations.
Application: Supports a wide range of AI workloads from training to inference.
The A100 is particularly effective in cloud environments where scalability and flexibility are paramount.
3. AMD MI250X
AMD's MI250X GPU is designed specifically for data centers and offers competitive performance for AI applications:
Memory: Features 128 GB of high-bandwidth memory.
Performance: Delivers up to 47 teraflops of double-precision performance.
Integration: Optimized for use in AMD's ROCm software platform, making it suitable for developers familiar with open-source tools.
The MI250X is gaining traction in cloud-based environments due to its cost-effectiveness and high performance.
4. Google TPU v4
Google's Tensor Processing Units (TPUs) are custom-built for machine learning tasks:
Performance: Each TPU v4 can deliver up to 275 teraflops.
Cloud Integration: Available exclusively through Google Cloud Platform, making it easy for developers to access powerful ML resources.
Efficiency: Designed for efficient scaling in distributed environments.
TPUs are particularly effective for large-scale training jobs, especially those utilizing TensorFlow.
5. NVIDIA L40S
The L40S is a newer addition to NVIDIA's lineup, targeting generative AI applications:
Memory: Comes with up to 48 GB GDDR6 memory.
Performance: Optimized for inference tasks with lower latency requirements.
Use Cases: Ideal for applications like real-time video processing and complex simulations.
Its efficient architecture makes it a strong contender in cloud-based GPU offerings.
6. Intel Data Center GPU Max Series
Intel's Data Center GPUs are designed to handle a variety of workloads:
Memory Options: Up to 64 GB of HBM2e memory.
Performance: Focused on delivering high throughput for AI inference tasks.
Integration: Works seamlessly with Intel's software ecosystem, providing developers with familiar tools.
These GPUs are becoming increasingly popular in cloud environments due to their flexibility and integration capabilities.
7. Qualcomm Cloud AI 100
Qualcomm's Cloud AI 100 is tailored for edge computing and AI inference:
Power Efficiency: Designed specifically for low power consumption while maintaining high performance.
Scalability: Effective in scenarios where multiple devices need to process data simultaneously.
Application Areas: Particularly useful in IoT applications where real-time processing is critical.
This GPU is an excellent choice for developers looking at edge AI solutions within cloud infrastructures.
8. NVIDIA T4 Tensor Core GPU
The T4 GPU is a versatile option that balances performance and cost:
Memory: Equipped with 16 GB GDDR6 memory.
Performance: Offers up to 65 teraflops of performance optimized for inference tasks.
Cloud Deployment: Widely available across various cloud platforms, making it accessible for many developers.
The T4 is particularly well-suited for smaller-scale projects or as part of a larger distributed system in an AI cloud environment.
Conclusion
Choosing the right GPU can significantly influence the success of machine learning projects. The options listed above represent some of the best modern GPUs available today, each offering unique advantages tailored to different workloads and budgets. As more organizations transition to cloud-based solutions, leveraging these powerful GPUs will be crucial for optimizing AI applications in AI Datacenter.
By utilizing cloud GPUs from providers like NeevCloud, developers can scale their projects efficiently while accessing cutting-edge technology without the overhead of managing physical infrastructure. With continuous advancements in GPU technology, staying informed about these developments will empower developers to make informed decisions that enhance their machine learning initiatives.
Incorporating these GPUs into your projects not only boosts performance but also aligns with the growing trend towards scalable, efficient AI solutions within the realm of cloud computing. Whether you're training complex models or deploying real-time inference systems, these modern GPUs are essential tools in the developer's arsenal.
Subscribe to my newsletter
Read articles from Tanvi Ausare directly inside your inbox. Subscribe to the newsletter, and don't miss out.
Written by
