What is Big Data? Understanding the 6 Vs of Big Data

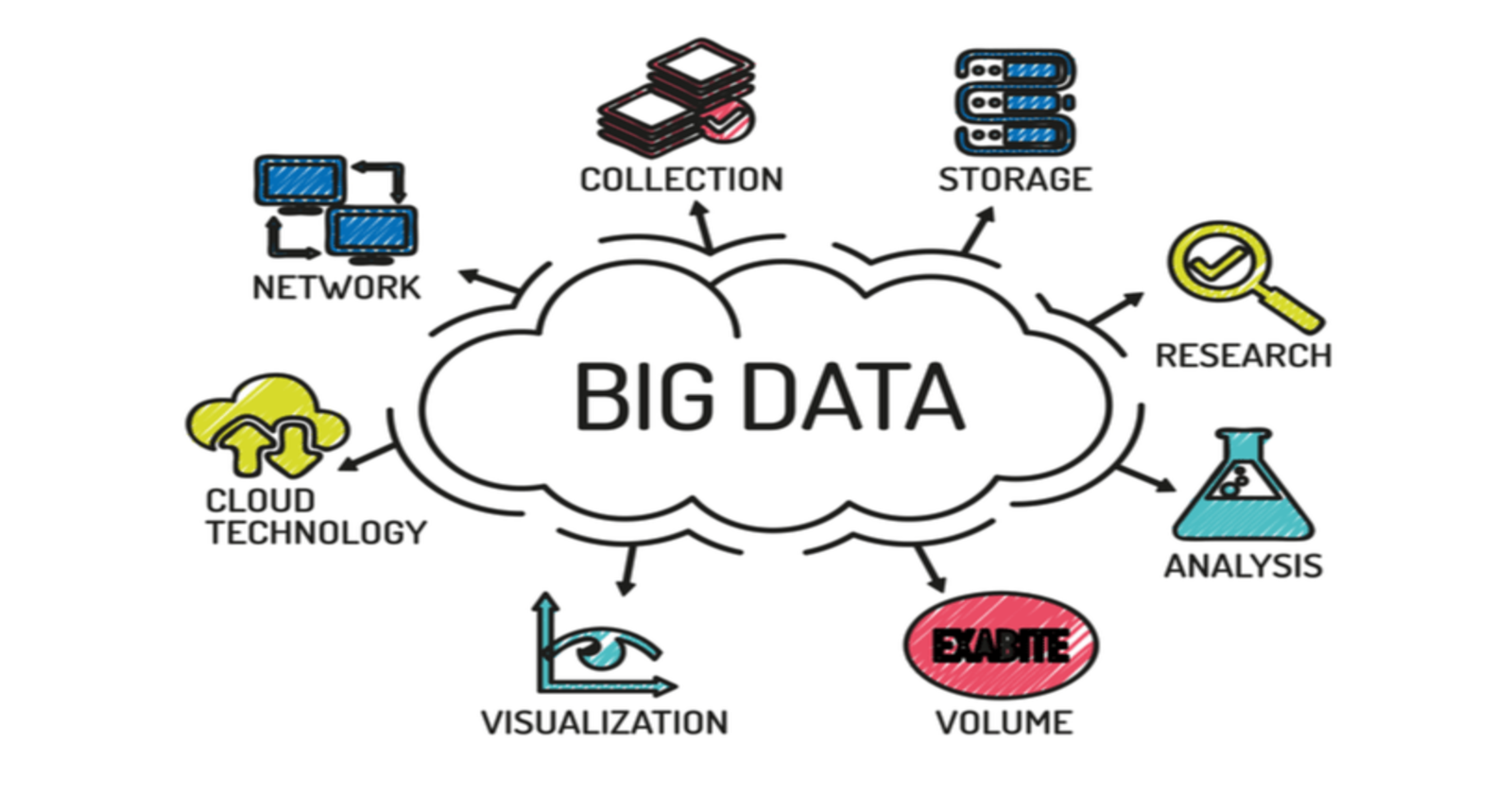
Introduction
Big Data is more than just a buzzword; it is a disruptive force that influences how we acquire, analyze, and use information. In this blog, we'll look at what Big Data really means and the 6 Vs—the fundamental characteristics that define its core and applications. These insights are inspired by the book Deciphering Data Architectures.
What is Big Data?
Big Data refers to datasets that are so enormous, complicated, and dynamic that typical data processing techniques cannot manage them successfully. This data is created from a variety of sources, including social media, IoT devices, sensors, transactions, and more, giving businesses the opportunity to discover previously unachievable trends, patterns, and insights.
The distinguishing factor of Big Data is not just its size but the speed, variety, and value it offers. To better understand it, we look at its six defining characteristics: Volume, Velocity, Variety, Veracity, Value, and Variability.
Deep Dive into Big Data and the 6 Vs
1. Volume: The Scale of Big Data
Volume refers to the vast quantities of data generated every second. It includes data from social media interactions, IoT sensors, financial transactions, and more. Key Insights:
Over 2.5 quintillion bytes of data are created daily.
Cloud storage solutions like AWS, Google Cloud, and Azure have emerged as scalable options to store and process this data.
Companies need distributed systems and architectures like Hadoop or Spark to handle the massive data size.
Example Use Case: Netflix collects terabytes of viewing data daily to analyze user preferences and recommend personalized content.
2. Velocity: The Speed of Data Generation
Velocity emphasizes the need for rapid processing. Data streams continuously and needs to be captured, analyzed, and acted upon in near real-time.
Technological Solutions:
Streaming platforms like Apache Kafka and Amazon Kinesis are used to handle high-speed data pipelines.
Real-time analytics tools like Tableau, Domo, and Looker are used to process insights on-the-fly.
Example Use Case: Fraud detection in banking requires analyzing thousands of transactions per second to flag unusual patterns immediately.
3. Variety: The Diversity of Data
The modern data landscape includes structured, semi-structured, and unstructured data formats.
Data Types Include:
Structured: Spreadsheets, relational databases.
Semi-structured: JSON, XML, and log files.
Unstructured: Social media posts, videos, audio files, and emails.
Challenges and Solutions:
The diversity of data formats makes integration difficult.
Data lakes (e.g., built on AWS S3 or Azure Data Lake) help store raw, diverse data for further processing.
Example Use Case: A single customer purchase generates receipts (structured), tweets or reviews (unstructured), and web cookies (semi-structured).
4. Veracity: The Trustworthiness of Data
Data quality is a critical challenge. Veracity involves ensuring that data is accurate, consistent, and reliable despite its source or format.
Key Strategies:
Implementing data cleaning and validation processes.
Using machine learning algorithms to detect anomalies and fill gaps in incomplete data.
Example Use Case: In healthcare, electronic medical records (EMRs) must be accurate to avoid misdiagnoses or incorrect treatments. Data cleaning ensures patient information is consistent across systems.
5. Value: Deriving Insights from Data
Value is the ultimate goal of Big Data. Without actionable insights, the rest of the Vs have no meaning.
Key Aspects:
Use AI and machine learning to unlock predictive and prescriptive insights.
Employ business intelligence tools (e.g., Power BI, Tableau) to visualize the data for decision-making.
Example Use Case:
Retailers like Amazon use purchase histories to predict trends and optimize inventory, improving both customer satisfaction and operational efficiency.
6. Variability: The Changing Nature of Data
Variability refers to fluctuations and inconsistencies in data over time.
Examples of Variability:
Seasonal spikes in e-commerce (e.g., Black Friday).
Changing customer preferences leading to new trends.
Challenges:
Handling these fluctuations requires dynamic and adaptive systems capable of scaling up or down as needed.
Example Use Case:
Social media platforms experience variability during global events (e.g., the FIFA World Cup) with surges in user-generated content.
Real-World Applications of the 6 Vs
To further illustrate the importance of Big Data and the 6 Vs, here are a few industry-specific examples:
Healthcare:
Wearable devices like Fitbits generate continuous streams of health data (velocity), requiring real-time analysis for monitoring patient vitals. The diversity of data includes heart rate (structured), voice notes to doctors (unstructured), and XML reports (semi-structured).E-commerce:
Platforms like eBay handle terabytes of data daily (volume) while addressing variability during holiday sales seasons.Smart Cities:
IoT devices in smart cities generate data from traffic sensors, weather stations, and utilities. The city planners analyze this data to optimize infrastructure and energy use.
Future of Big Data
As technology evolves, the dimensions of Big Data are expected to grow further:
AI Integration: Advanced AI will help in predictive analytics and uncovering insights faster.
Edge Computing: With IoT and 5G, data will increasingly be processed closer to the source, reducing latency.
Ethical Data Use: Growing concerns over data privacy and security will push organizations to adopt stricter compliance measures.
Call to Action
Big Data is not just a technical challenge but a massive opportunity for businesses and individuals. The 6 Vs provide a clear framework to understand and utilize its potential.
What are your thoughts on Big Data and its transformative power? Share your insights or ask questions in the comments below!
Subscribe to my newsletter
Read articles from Omkar Mante directly inside your inbox. Subscribe to the newsletter, and don't miss out.
Written by
